Graph-Based Semisupervised Learning for Acoustic Modeling in Automatic Speech Recognition.
IEEE/ACM Trans. Audio, Speech & Language Processing(2016)
摘要
In this paper, we investigate how to apply graph-based semisupervised learning to acoustic modeling in speech recognition. Graph-based semisupervised learning is a widely used transductive semisupervised learning method in which labeled and unlabeled data are jointly represented as a weighted graph; the resulting graph structure is then used as a constraint during the classification of unlabeled data points. We investigate suitable graph-based learning algorithms for speech data and evaluate two different frameworks for integrating graph-based learning into state-of-the-art, deep neural network DDN-based speech recognition systems. The first framework utilizes graph-based learning in parallel with a DNN classifier within a lattice-rescoring framework, whereas the second framework relies on an embedding of graph neighborhood information into continuous space using an autoencoder. We demonstrate significant improvements in framelevel phonetic classification accuracy and consistent reductions in word error rate on large-vocabulary conversational speech recognition tasks.
更多查看译文
关键词
Speech recognition,Acoustics,Speech,Adaptation models,Speech processing,Semisupervised learning,Training
AI 理解论文
溯源树
样例
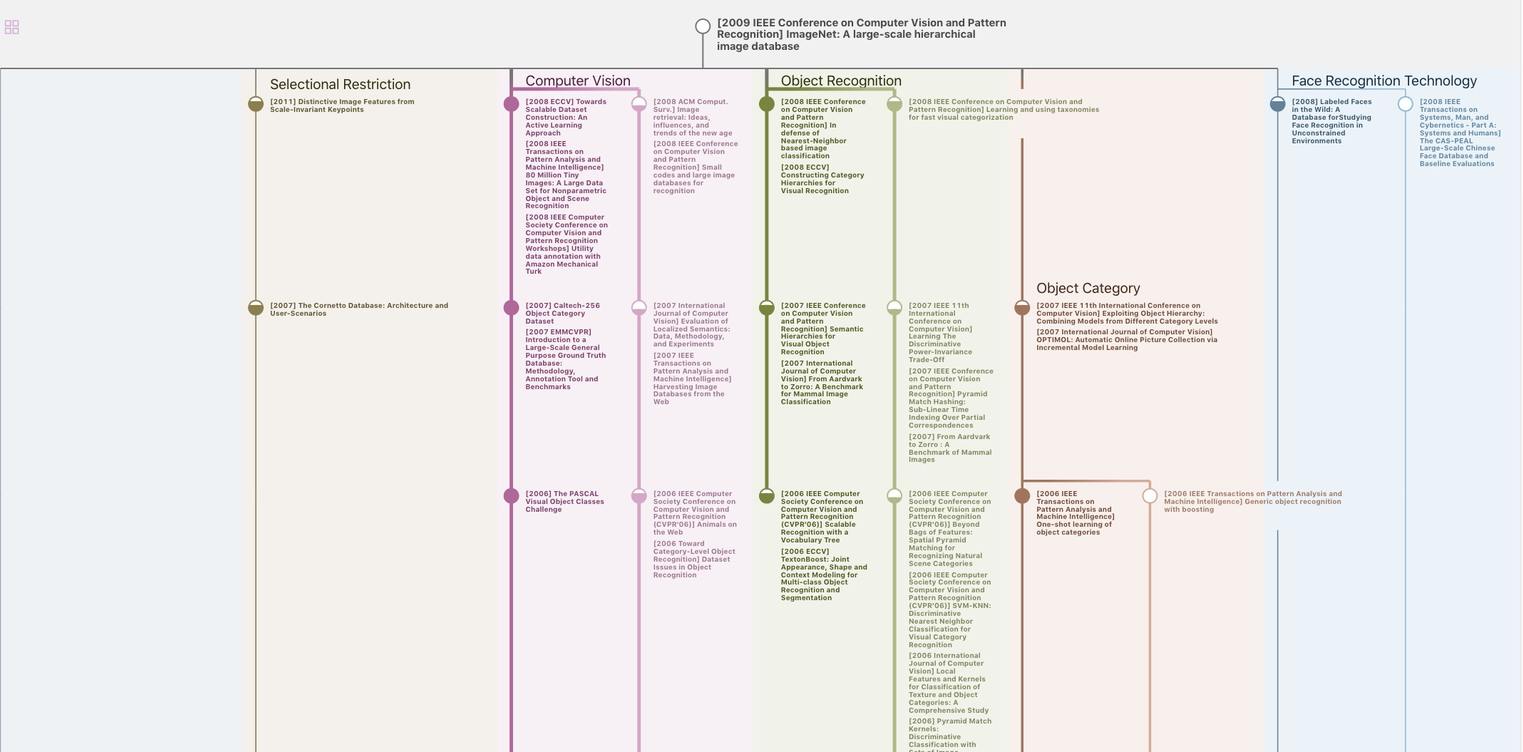
生成溯源树,研究论文发展脉络
Chat Paper
正在生成论文摘要