Detecting signals of detrimental prescribing cascades from social media.
Artificial Intelligence in Medicine(2016)
摘要
HighlightsPrescribing cascade can be associated with adverse effects.Timely detection of detrimental prescribing cascades is essential.Social media is a promising source to detect detrimental prescribing cascades.Sequence mining is used to signal detrimental prescribing cascades from social media. MotivationPrescribing cascade (PC) occurs when an adverse drug reaction (ADR) is misinterpreted as a new medical condition, leading to further prescriptions for treatment. Additional prescriptions, however, may worsen the existing condition or introduce additional adverse effects (AEs). Timely detection and prevention of detrimental PCs is essential as drug AEs are among the leading causes of hospitalization and deaths. Identifying detrimental PCs would enable warnings and contraindications to be disseminated and assist the detection of unknown drug AEs. Nonetheless, the detection is difficult and has been limited to case reports or case assessment using administrative health claims data. Social media is a promising source for detecting signals of detrimental PCs due to the public availability of many discussions regarding treatments and drug AEs. ObjectiveIn this paper, we investigate the feasibility of detecting detrimental PCs from social media. MethodsThe detection, however, is challenging due to the data uncertainty and data rarity in social media. We propose a framework to mine sequences of drugs and AEs that signal detrimental PCs, taking into account the data uncertainty and data rarity. ResultsWe conduct experiments on two real-world datasets collected from Twitter and Patient health forum. Our framework achieves encouraging results in the validation against known detrimental PCs (F1=78% for Twitter and 68% for Patient) and the detection of unknown potential detrimental PCs (Precision@50=72% and NDCG@50=95% for Twitter, Precision@50=86% and NDCG@50=98% for Patient). In addition, the framework is efficient and scalable to large datasets. ConclusionOur study demonstrates the feasibility of generating hypotheses of detrimental PCs from social media to reduce pharmacists' guesswork.
更多查看译文
关键词
Adverse effect,Detrimental prescribing cascade,Drug,Existence uncertainty,Order uncertainty,Sequence mining,Social media
AI 理解论文
溯源树
样例
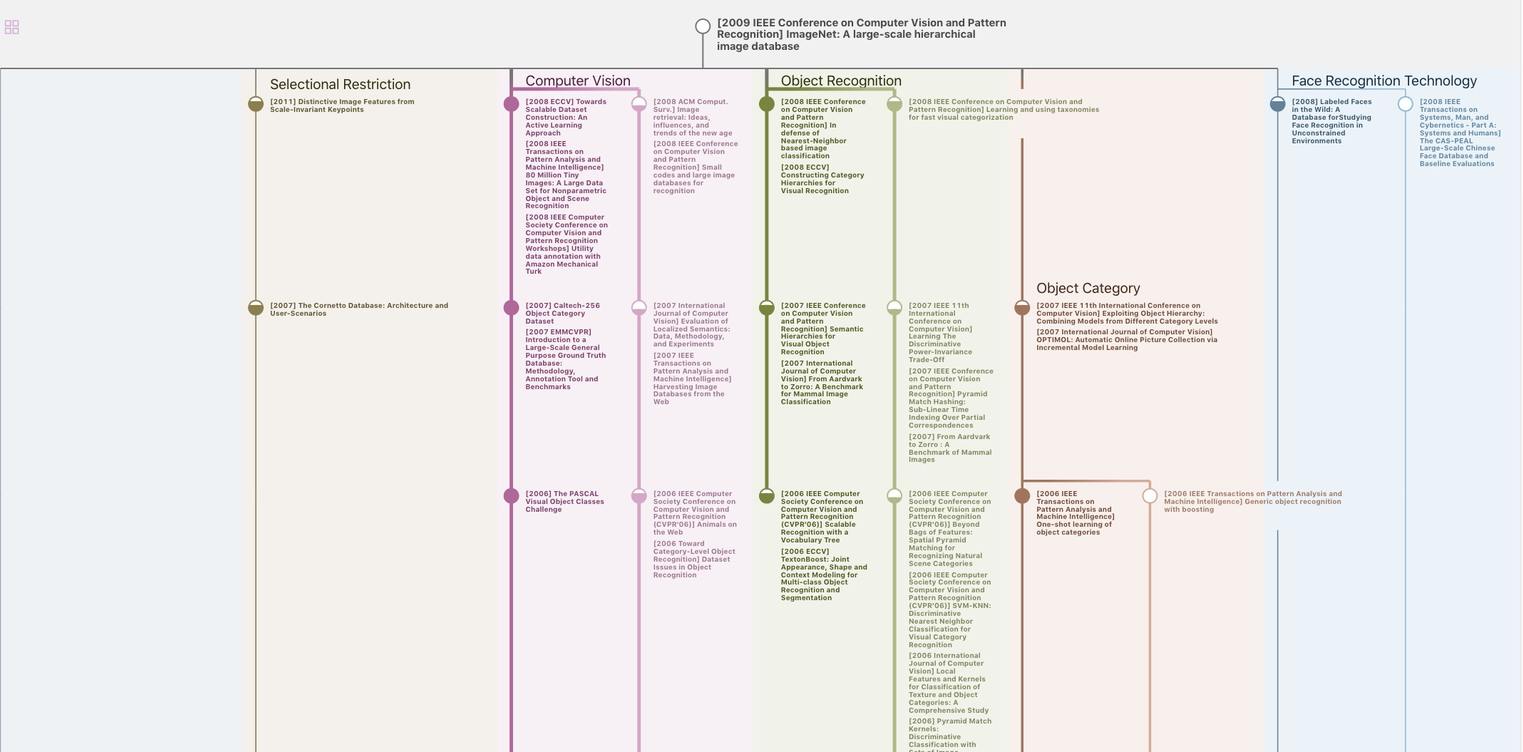
生成溯源树,研究论文发展脉络
Chat Paper
正在生成论文摘要