Robust label compression for multi-label classification.
Knowl.-Based Syst.(2016)
摘要
This paper deals with label compression of multi-label classification.It is the first paper considering outliers in label compression.Outliers in the feature space are taken into account.Irregular label correlations can also be thought as outliers.This paper tackles this problem by using l2,1-norm. Label compression (LC) is an effective strategy to reduce time cost and improve classification performance simultaneously for multi-label classification. One main limitation of existing LC methods is that they are prone to outliers. Here outliers include outliers in the feature space and outliers in the label space. Outliers in the feature space are obtained due to data acquisition devices. Outliers in the label space refer to label vectors that are inconsistent with the regular label correlations. In this paper, we propose a new LC method, termed robust label compression (RLC), based on l2,1-norm to deal with outliers in the feature space and label space. The objective function of RLC consists of two losses: the encoding loss to measure the compression error and the dependence loss to measure the relevance between the instances and the obtained code vectors after compressing the label vectors. To achieve robustness to outliers, we utilize the l2,1-norm on both losses. We propose an efficient optimization algorithm for it and present theoretical analysis. Experiments across six data sets validate the superiority of our proposed method to state-of-art LC methods for multi-label classification.
更多查看译文
关键词
Multi-label classification,Label compression,Encoding loss,Dependence loss,Outliers,l2,1-norm
AI 理解论文
溯源树
样例
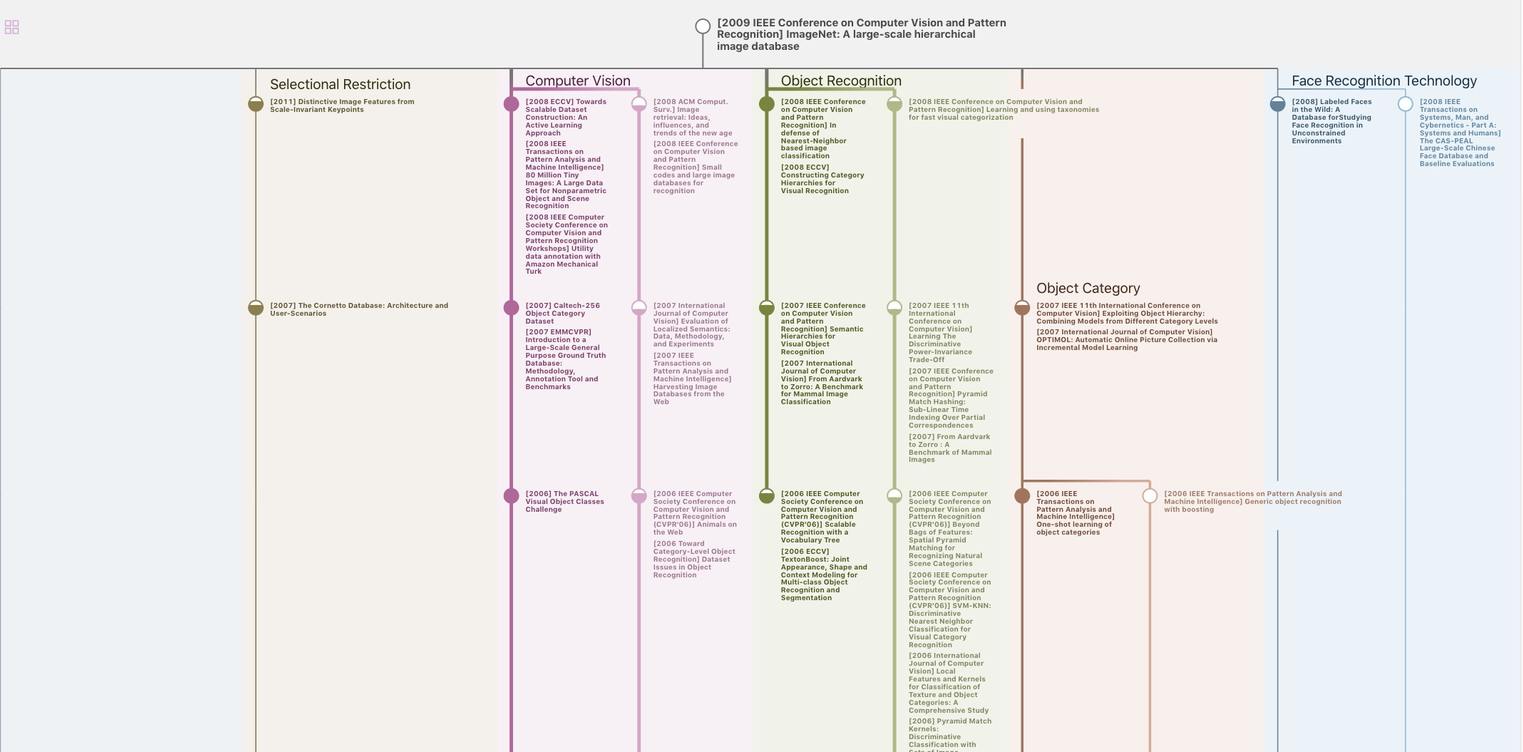
生成溯源树,研究论文发展脉络
Chat Paper
正在生成论文摘要