Novelty Based Ranking Of Human Answers For Community Questions
SIGIR '16: The 39th International ACM SIGIR conference on research and development in Information Retrieval Pisa Italy July, 2016(2016)
摘要
Questions and their corresponding answers within a community-based question answering (CQA) site are frequently presented as top search results for Web search queries and viewed by millions of searchers daily. The number of answers for CQA questions ranges from a handful to dozens, and a searcher would be typically interested in the different suggestions presented in various answers for a question. Yet, especially when many answers are provided, the viewer may not want to sift through all answers but to read only the top ones. Prior work on answer ranking in CQA considered the qualitative notion of each answer separately, mainly whether it should be marked as best answer. We propose to promote CQA answers not only by their relevance to the question but also by the diversification and novelty qualities they hold compared to other answers. Specifically, we aim at ranking answers by the amount of new aspects they introduce with respect to higher ranked answers (novelty), on top of their relevance estimation. This approach is common in Web search and information retrieval, yet it was not addressed within the CQA settings before, which is quite different from classic document retrieval. We propose a novel answer ranking algorithm that borrows ideas from aspect ranking and multi-document summarization, but adapts them to our scenario. Answers are ranked in a greedy manner, taking into account their relevance to the question as well as their novelty compared to higher ranked answers and their coverage of important aspects. An experiment over a collection of Health questions, using a manually annotated gold-standard dataset, shows that considering novelty for answer ranking improves the quality of the ranked answer list.
更多查看译文
关键词
Community-based question answering,Novelty,Diversification
AI 理解论文
溯源树
样例
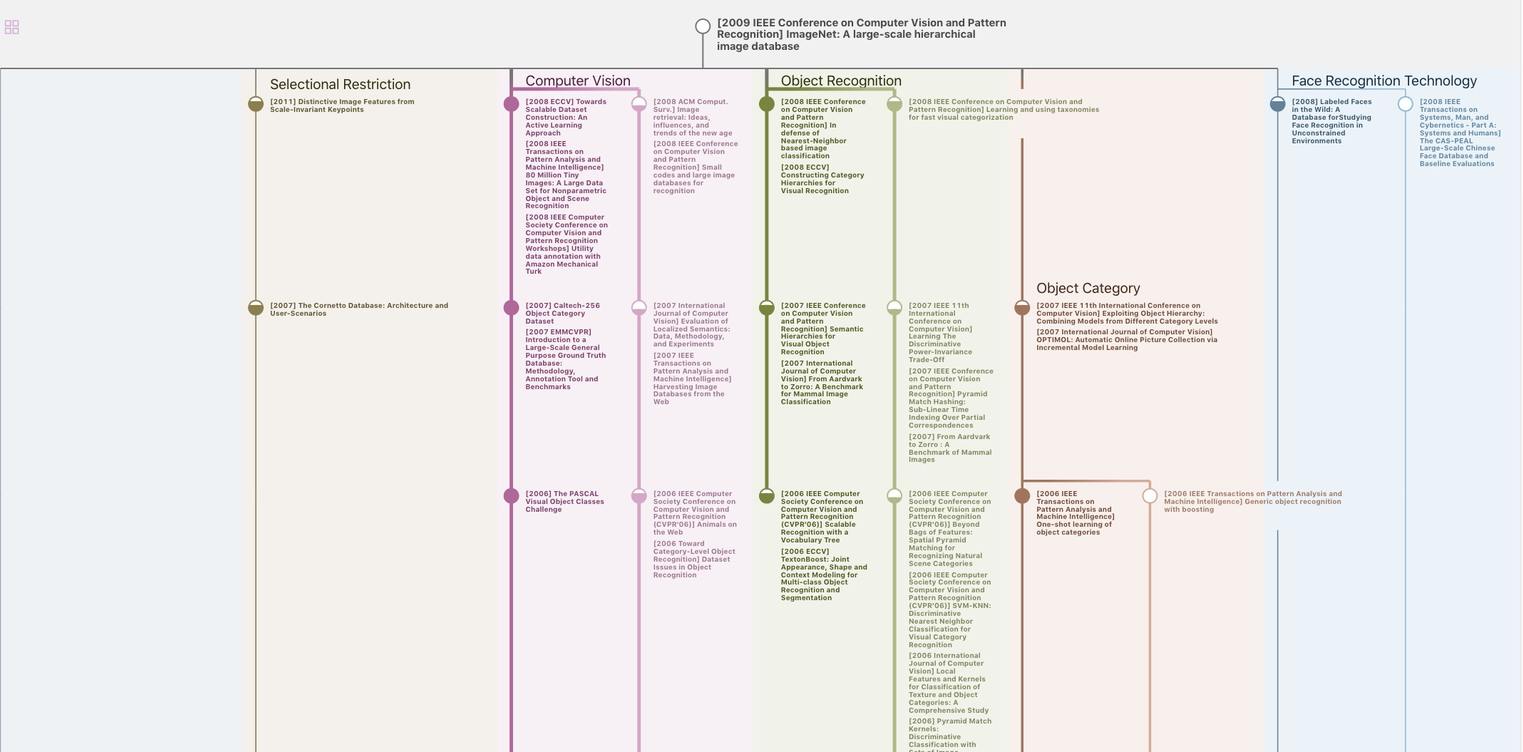
生成溯源树,研究论文发展脉络
Chat Paper
正在生成论文摘要