Pulp: A System For Exploratory Search Of Scientific Literature
SIGIR '16: The 39th International ACM SIGIR conference on research and development in Information Retrieval Pisa Italy July, 2016(2016)
摘要
Despite the growing importance of exploratory search, information retrieval (IR) systems tend to focus on lookup search. Lookup searches are well served by optimising the precision and recall of search results, however, for exploratory search this may be counterproductive if users are unable to formulate an appropriate search query. We present a system called PULP that supports exploratory search for scientific literature, though the system can be easily adapted to other types of literature. PULP uses reinforcement learning (RL) to avert the user from context traps resulting from poorly chosen search queries, trading off between exploration (presenting the user with diverse topics) and exploitation (moving towards more specific topics). Where other RL-based systems suffer from the "cold start" problem, requiring sufficient time to adjust to a user's information needs, PULP initially presents the user with an overview of the dataset using temporal topic models. Topic models are displayed in an interactive alluvial diagram, where topics are shown as ribbons that change thickness with a given topics relative prevalence over time. Interactive, exploratory search sessions can be initiated by selecting topics as a starting point.
更多查看译文
关键词
Exploratory search,topic models,bandit algorithms,scientific literature search,query formulation
AI 理解论文
溯源树
样例
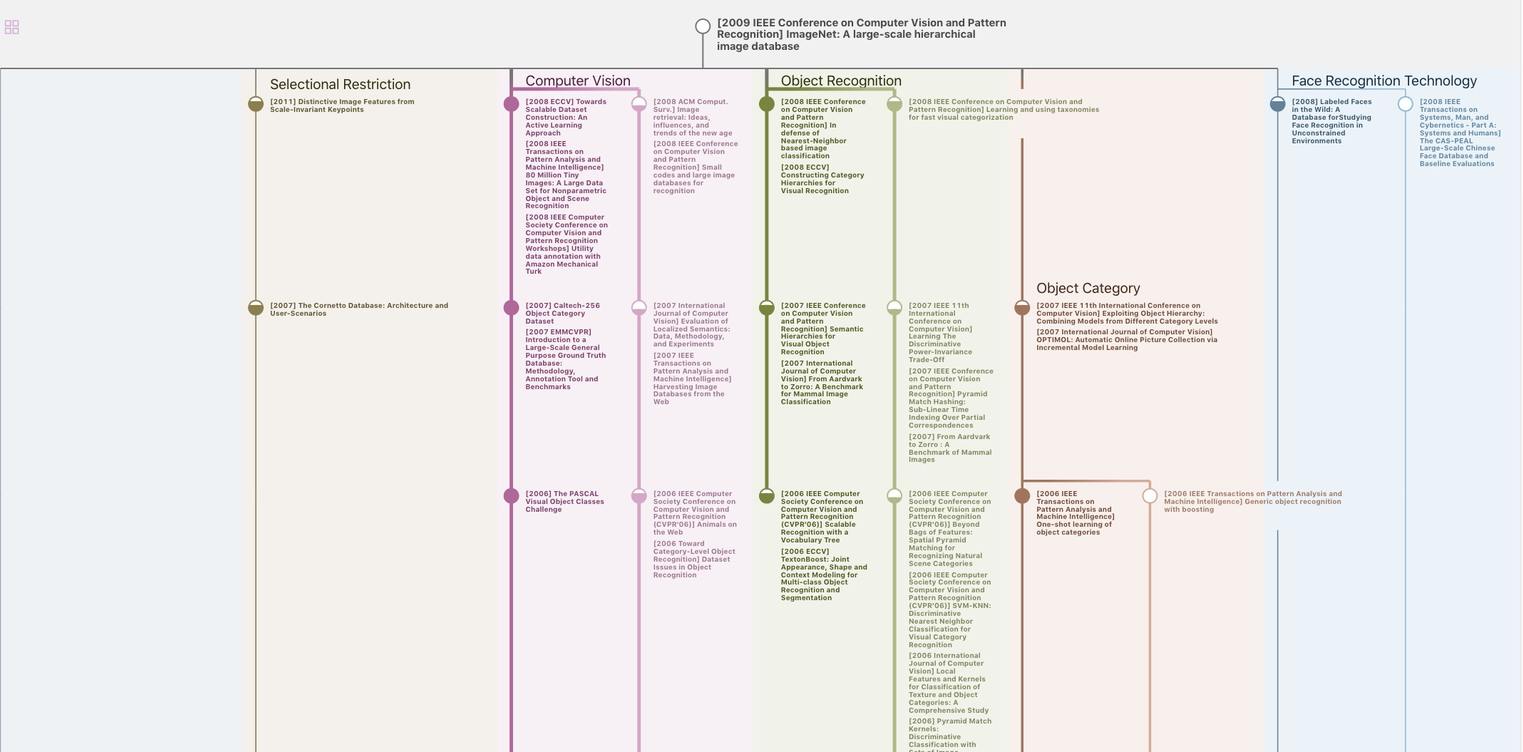
生成溯源树,研究论文发展脉络
Chat Paper
正在生成论文摘要