Learning From the Crowd: Observational Learning in Crowdsourcing Communities.
CHI(2016)
摘要
Crowd work provides solutions to complex problems effectively, efficiently, and at low cost. Previous research showed that feedback, particularly correctness feedback can help crowd workers improve their performance; yet such feedback, particularly when generated by experts, is costly and difficult to scale. In our research we investigate approaches to facilitating continuous observational learning in crowdsourcing communities. In a study conducted with workers on Amazon Mechanical Turk, we asked workers to complete a set of tasks identifying nutritional composition of different meals. We examined workers' accuracy gains after being exposed to expert-generated feedback and to two types of peer-generated feedback: direct accuracy assessment with explanations of errors, and a comparison with solutions generated by other workers. The study further confirmed that expert-generated feedback is a powerful mechanism for facilitating learning and leads to significant gains in accuracy. However, the study also showed that comparing one's own solutions with a variety of solutions suggested by others and their comparative frequencies leads to significant gains in accuracy. This solution is particularly attractive because of its low cost, minimal impact on time and cost of job completion, and high potential for adoption by a variety of crowdsourcing platforms.
更多查看译文
关键词
Crowdsourcing, nutritional assessment, observational learning
AI 理解论文
溯源树
样例
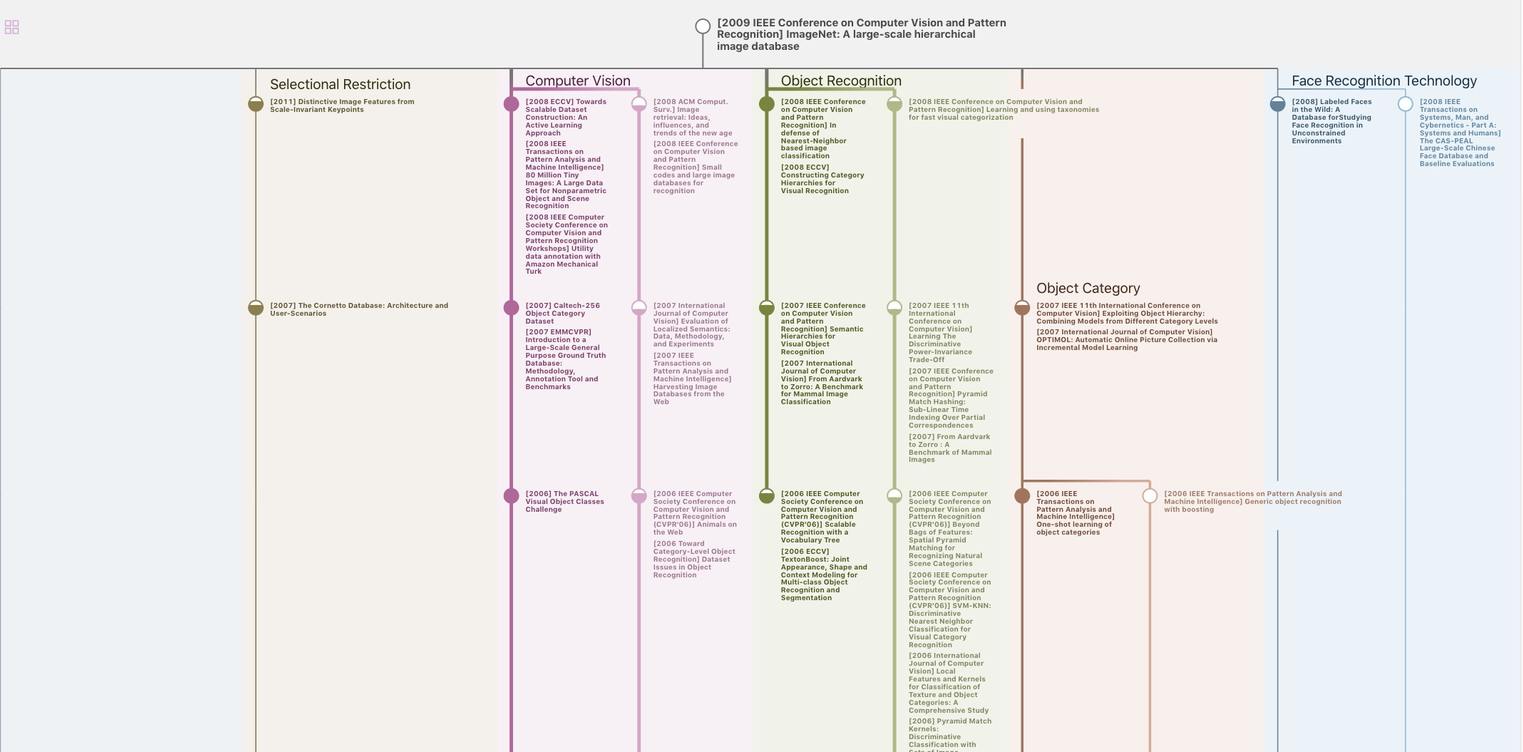
生成溯源树,研究论文发展脉络
Chat Paper
正在生成论文摘要