Pac Continuous State Online Multitask Reinforcement Learning With Identification
AAMAS '16: Proceedings of the 2016 International Conference on Autonomous Agents & Multiagent Systems(2016)
摘要
One key feature of a general intelligent autonomous agent is to be able to learn from past experience to improve future performance. In this paper we consider how an agent can leverage prior experience from performing reinforcement learning in order to learn faster in future tasks. We introduce the first, to our knowledge, probably approximately correct (PAC) RL algorithm COMRLI for sequential multi-task learning across a series of continuous-state, discreteaction RL tasks. We assume tasks are sampled from a finite number of clusters of Markov decision processes, and provide a bound on the number of steps on which the algorithm makes a suboptimal decision that is substantially smaller on later tasks. We also provide preliminary evidence to suggest our approach may be useful in practice, by showing encouraging simulation performance in a standard domain where it compares favorably to a state-of-the-art algorithm.
更多查看译文
关键词
reinforcement,identification,learning,online
AI 理解论文
溯源树
样例
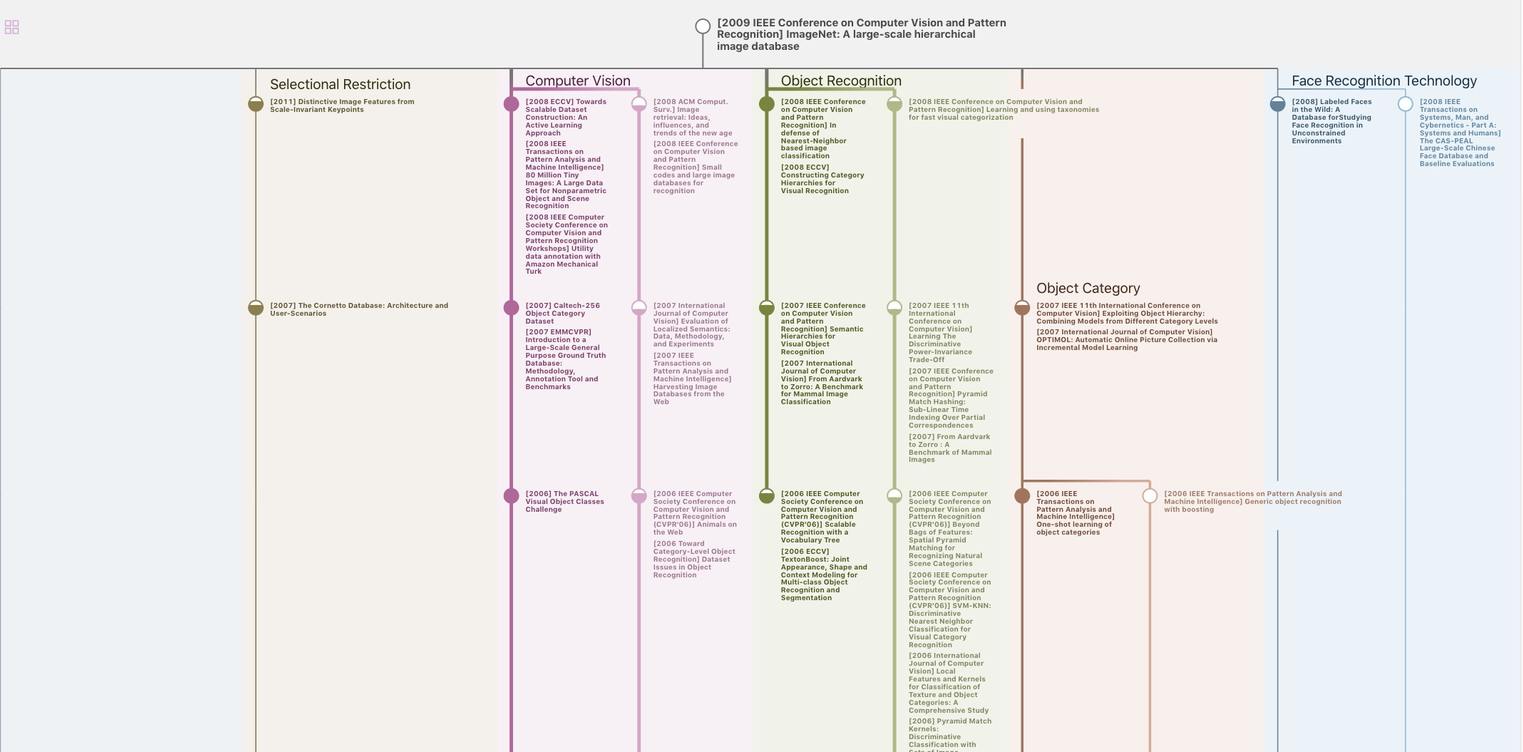
生成溯源树,研究论文发展脉络
Chat Paper
正在生成论文摘要