Image Classification With Orchard Metadata
2016 IEEE International Conference on Robotics and Automation (ICRA)(2016)
摘要
Low cost and easy to use monocular vision systems are able to capture large scale, dense data in orchards, to facilitate precision agriculture applications. Accurate image parsing is required for this purpose, however, operating natural outdoor conditions makes this a complex task due to the undesirable intra-class variations caused by changes in illumination, pose and tree types, etc. Typically these variations are difficult to explicitly model and discriminative classifiers strive to be invariant to them. However, given the presence of structure, in both the orchard and how the data was obtained, a subset of these factors of variations can correlate with readily available metadata, including extrinsic experimental information such as the sun incidence angle, position within farm, etc. This paper presents a method to incorporate such metadata to aid scene parsing based on a multi-scale Multi-Layered Perceptron (MLP) architecture. Experimental results are shown for pixel segmentation over data collected at an apple orchard, leading to fruit detection and yield estimation. The results show a consistent improvement in segmentation accuracy with the inclusion of metadata under different network complexities, training configurations and evaluation metrics.
更多查看译文
关键词
image classification,orchard metadata,monocular vision systems,precision agriculture applications,image parsing,intra-class variations,multiscale multilayered perceptron architecture,MLP architecture,pixel segmentation,apple orchard,fruit detection,yield estimation
AI 理解论文
溯源树
样例
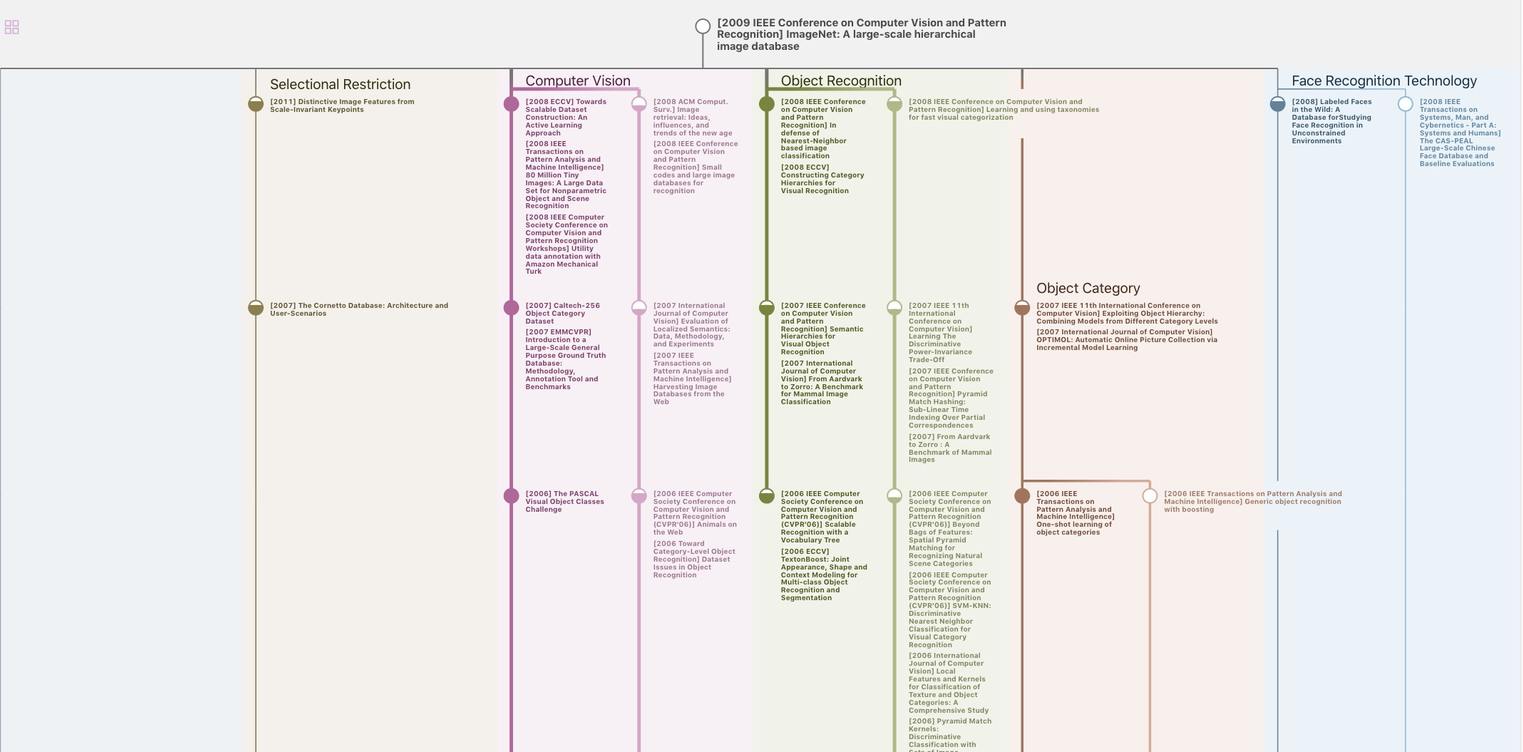
生成溯源树,研究论文发展脉络
Chat Paper
正在生成论文摘要