Scenenet: An Annotated Model Generator For Indoor Scene Understanding
2016 IEEE International Conference on Robotics and Automation (ICRA)(2016)
摘要
We introduce SceneNet, a framework for generating high-quality annotated 3D scenes to aid indoor scene understanding. SceneNet leverages manually-annotated datasets of real world scenes such as NYUv2 to learn statistics about object co-occurrences and their spatial relationships. Using a hierarchical simulated annealing optimisation, these statistics are exploited to generate a potentially unlimited number of new annotated scenes, by sampling objects from various existing databases of 3D objects such as ModelNet, and textures such as OpenSurfaces and ArchiveTextures. Depending on the task, SceneNet can be used directly in the form of annotated 3D models for supervised training and 3D reconstruction benchmarking, or in the form of rendered annotated sequences of RGB-D frames or videos.
更多查看译文
关键词
SceneNet framework,indoor scene understanding,3D scene annotation,hierarchical simulated annealing optimisation,statistics learning,3D object database,OpenSurfaces,ArchiveTextures,ModelNet database,3D reconstruction benchmarking,supervised training,rendered annotated sequences
AI 理解论文
溯源树
样例
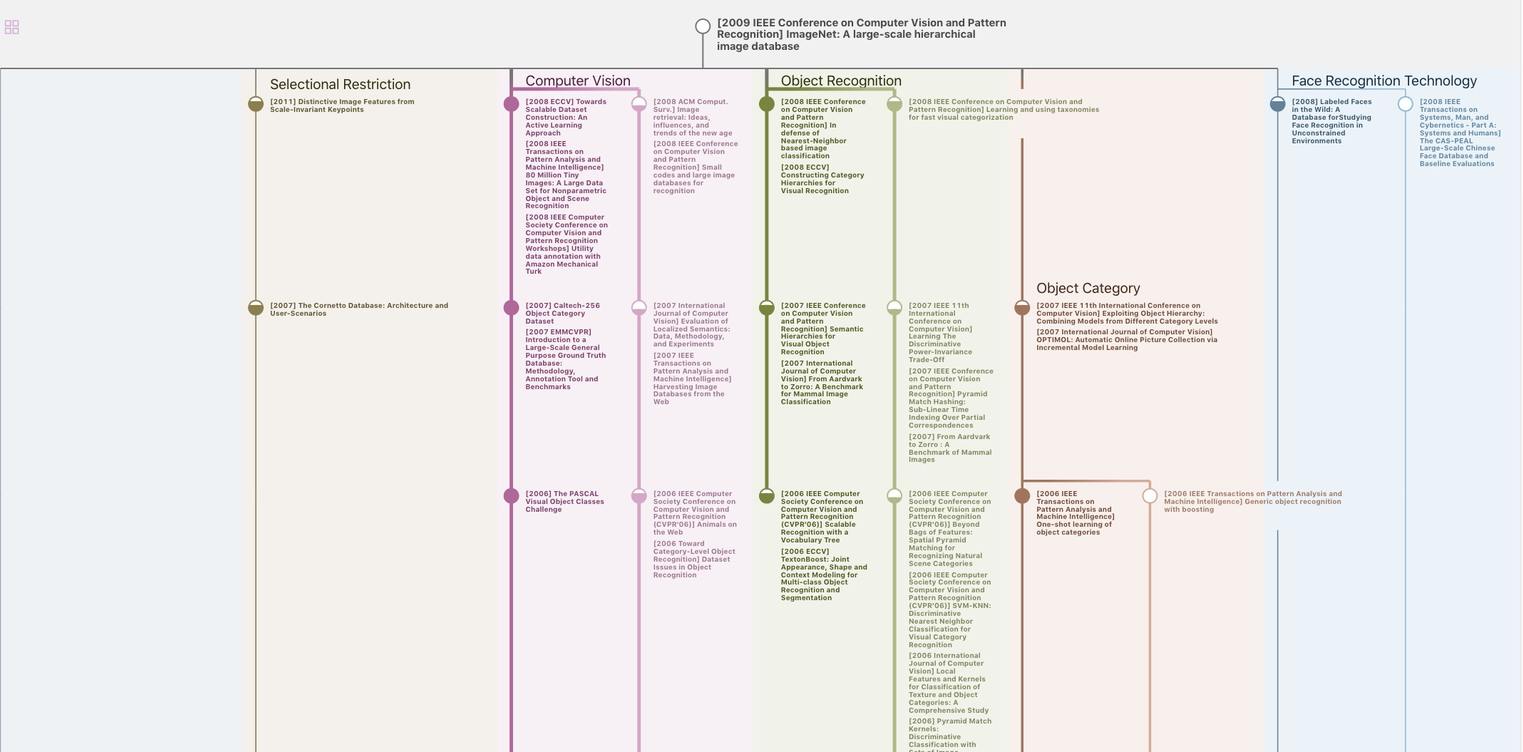
生成溯源树,研究论文发展脉络
Chat Paper
正在生成论文摘要