A Scalable Clustering-Based Local Multi-Label Classification Method
ECAI'16: Proceedings of the Twenty-second European Conference on Artificial Intelligence(2016)
摘要
Multi-label classification aims to assign multiple labels to a single test instance. Recently, more and more multi-label classification applications arise as large-scale problems, where the numbers of instances, features and labels are either or all large. To tackle such problems, in this paper we develop a clustering-based local multi-label classification method, attempting to reduce the problem size in instances, features and labels. Our method consists of low-dimensional data clustering and local model learning. Specifically, the original dataset is firstly decomposed into several regular-scale parts by applying clustering analysis on the feature subspace, which is induced by a supervised multi-label dimension reduction technique; then, an efficient local multi-label model, meta-label classifier chains, is trained on each data cluster. Given a test instance, only the local model belonging to the nearest cluster to it is activated to make the prediction. Extensive experiments performed on eighteen benchmark datasets demonstrated the efficiency of the proposed method compared with the state-of-the-art algorithms.
更多查看译文
AI 理解论文
溯源树
样例
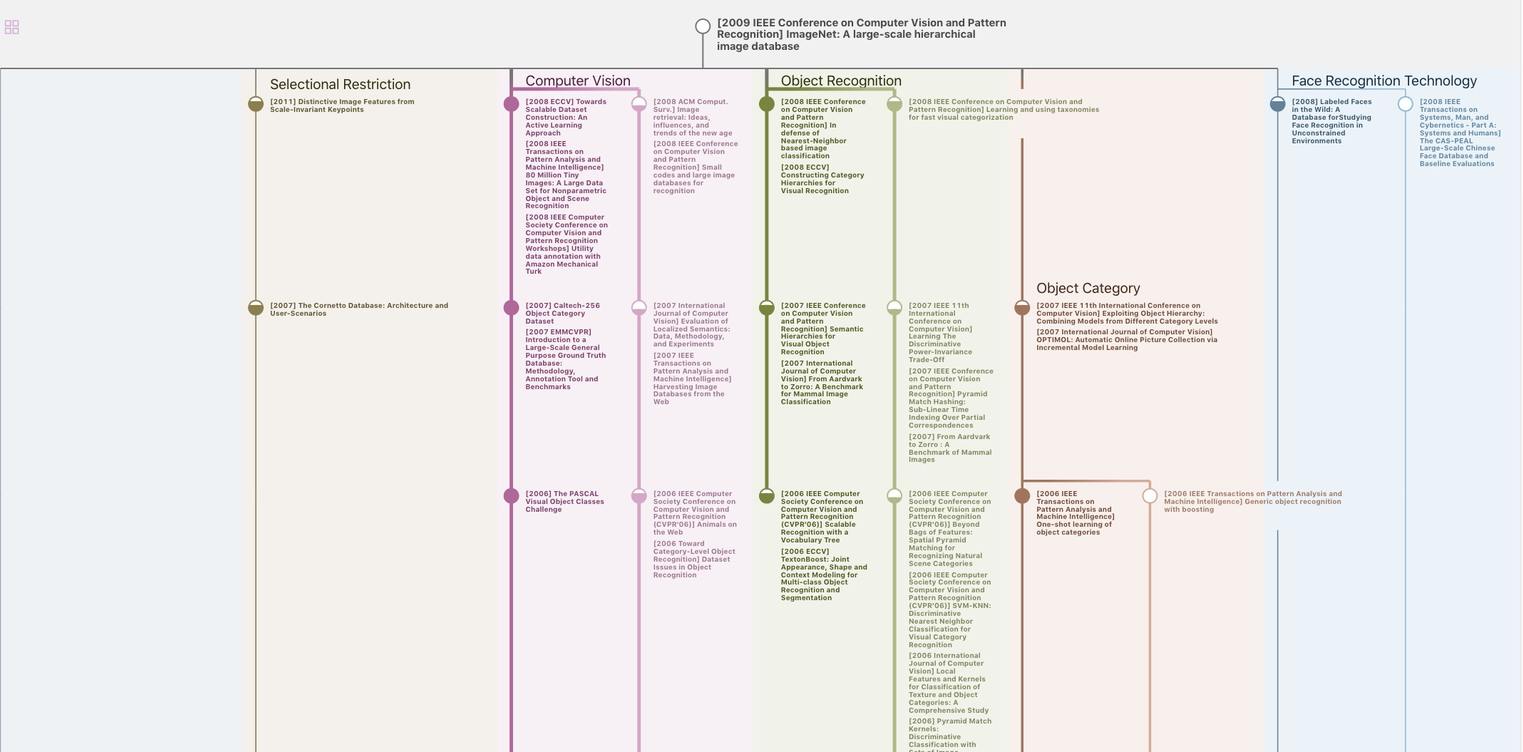
生成溯源树,研究论文发展脉络
Chat Paper
正在生成论文摘要