De-Anonymizing Social Networks And Inferring Private Attributes Using Knowledge Graphs
IEEE INFOCOM 2016 - The 35th Annual IEEE International Conference on Computer Communications(2016)
摘要
Social network data is widely shared, transferred and published for research purposes and business interests, but it has raised much concern on users privacy. Even though users identity information is always removed, attackers can still de-anonymize users with the help of auxiliary information. To protect against de-anonymization attack, various privacy protection techniques for social networks have been proposed. However, most existing approaches assume specific and restrict network structure as background knowledge and ignore semantic level prior belief of attackers, which are not always realistic in practice and do not apply to arbitrary privacy scenarios. Moreover, the privacy inference attack in the presence of semantic background knowledge is barely investigated. To address these shortcomings, in this work, we introduce knowledge graphs to explicitly express arbitrary prior belief of the attacker for any individual user. The processes of de-anonymization and privacy inference are accordingly formulated based on knowledge graphs. Our experiment on data of real social networks shows that knowledge graphs can strengthen de-anonymization and inference attacks, and thus increase the risk of privacy disclosure. This suggests the validity of knowledge graphs as a general effective model of attackers background knowledge for social network privacy preservation
更多查看译文
关键词
Social network data publishing,attack and privacy preservation,knowledge graph
AI 理解论文
溯源树
样例
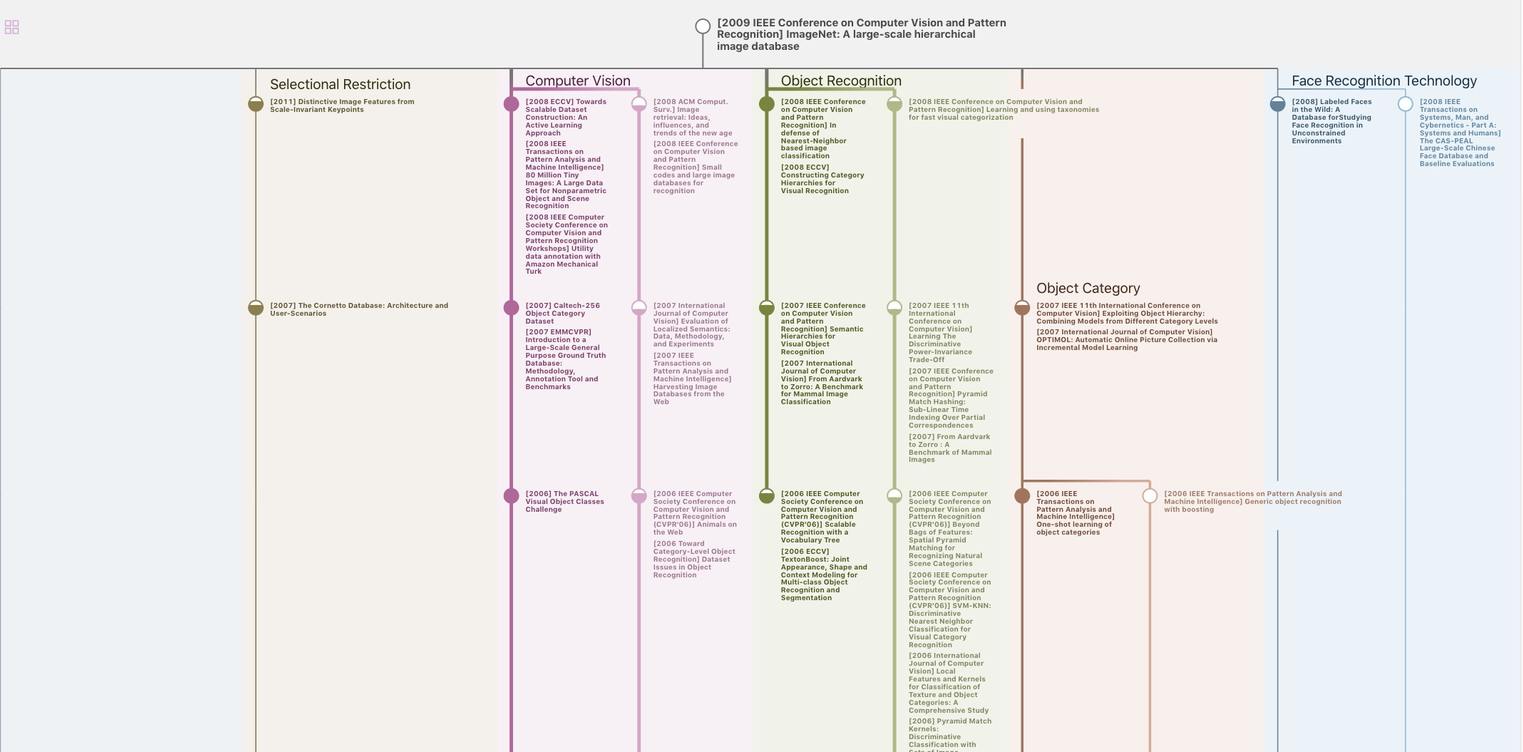
生成溯源树,研究论文发展脉络
Chat Paper
正在生成论文摘要