Evolving Controllers for Robots with Multimodal Locomotion.
Lecture Notes in Computer Science(2016)
摘要
Animals have inspired numerous studies on robot locomotion, but the problem of how autonomous robots can learn to take advantage of multimodal locomotion remains largely unexplored. In this paper, we study how a robot with two different means of locomotion can effective learn when to use each one based only on the limited information it can obtain through its onboard sensors. We conduct a series of simulation-based experiments using a task where a wheeled robot capable of jumping has to navigate to a target destination as quickly as possible in environments containing obstacles. We apply evolutionary techniques to synthesize neural controllers for the robot, and we analyze the evolved behaviors. The results show that the robot succeeds in learning when to drive and when to jump. The results also show that, compared with unimodal locomotion, multimodal locomotion allows for simpler and higher performing behaviors to evolve.
更多查看译文
关键词
Evolutionary Robotics,Multimodal locomotion,Navigation task
AI 理解论文
溯源树
样例
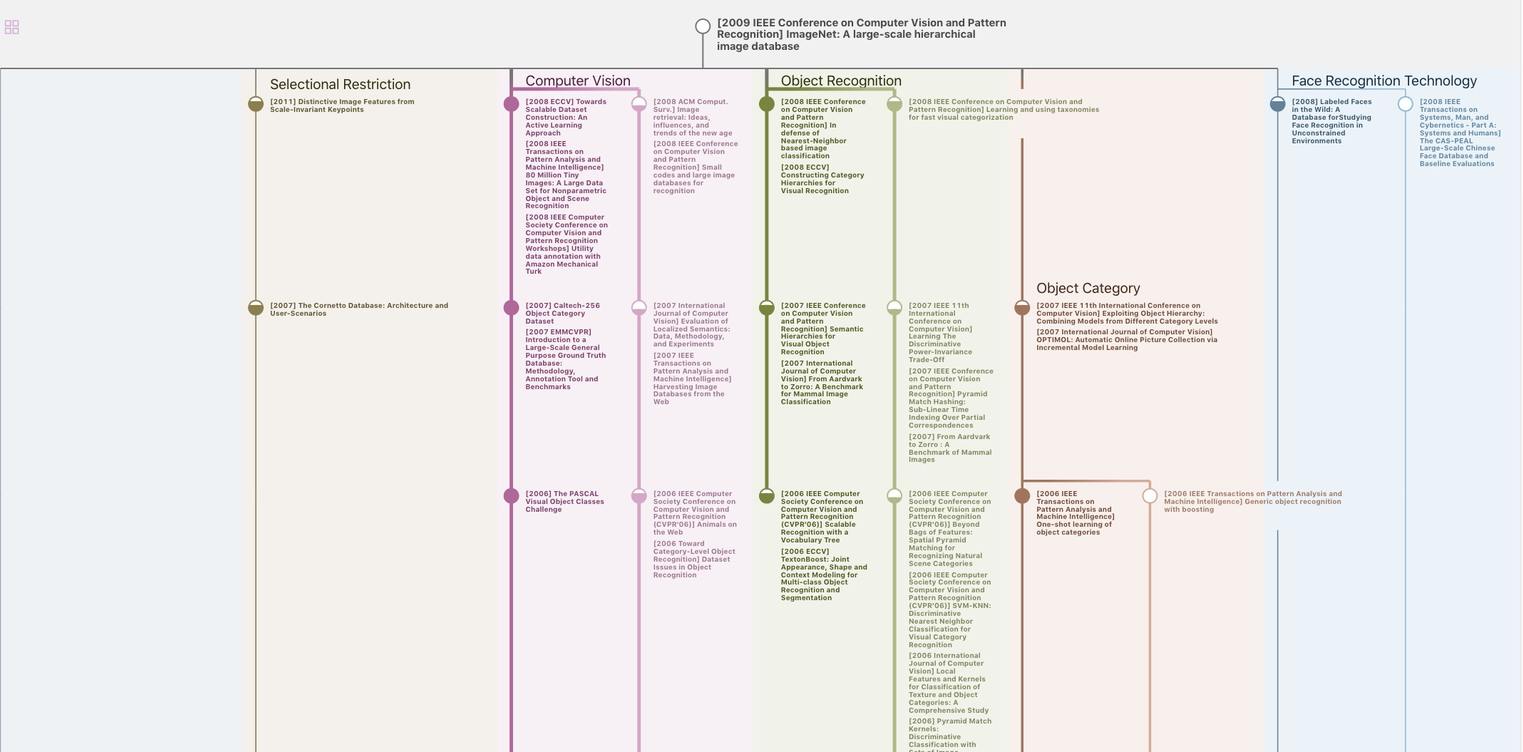
生成溯源树,研究论文发展脉络
Chat Paper
正在生成论文摘要