Redundancy reduction: does it help associative classifiers?
SAC 2016: Symposium on Applied Computing Pisa Italy April, 2016(2016)
摘要
The number of classification rules discovered in associative classification is typically quite large. In addition, these rules contain redundant information since classification rules are obtained from mined frequent itemsets and the latter are known to be repetitive. In this paper we investigate through an empirical study the performance of associative classifiers when the classification rules are generated from frequent, closed and maximal itemsets. We show that maximal itemsets substantially reduce the number of classification rules without jeopardizing the accuracy of the classifier. Our extensive analysis demonstrates that the performance remains stable and even improves in some cases. Our analysis using cost curves also provides recommendations on when it is appropriate to remove redundancy in frequent itemsets.
更多查看译文
关键词
reduction
AI 理解论文
溯源树
样例
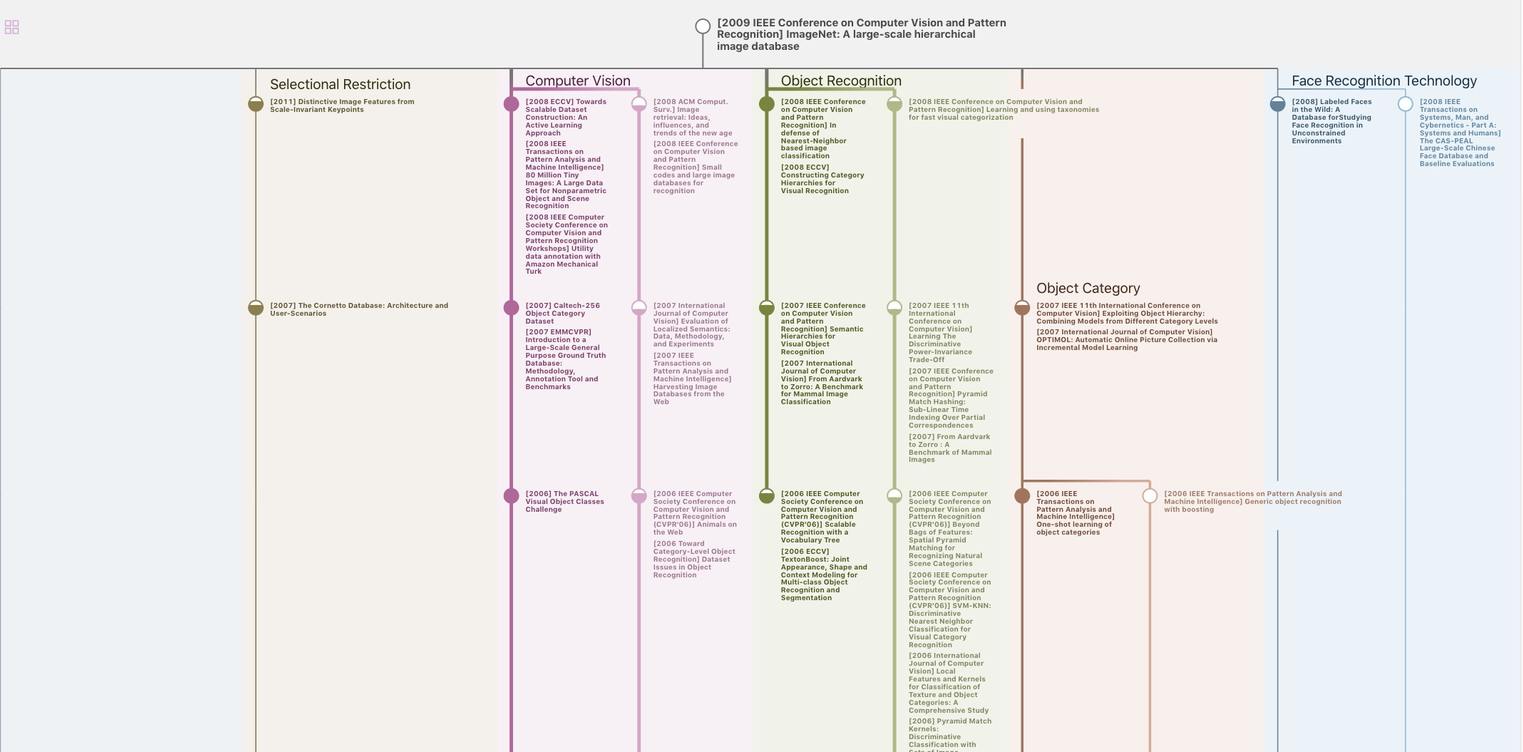
生成溯源树,研究论文发展脉络
Chat Paper
正在生成论文摘要