Variational Inference For Infinite Mixtures Of Sparse Gaussian Processes Through Kl-Correction
2016 IEEE INTERNATIONAL CONFERENCE ON ACOUSTICS, SPEECH AND SIGNAL PROCESSING PROCEEDINGS(2016)
Abstract
We propose a new approximation method for Gaussian process ( GP) regression based on the mixture of experts structure and variational inference. Our model is essentially an infinite mixture model in which each component is composed of a Gaussian distribution over the input space, and a Gaussian process expert over the output space. Each expert is a sparse GP model augmented with its own set of inducing points. Variational inference is made feasible by assuming that the training outputs are independent given the inducing points. In previous works on variational mixture of GP experts, the inducing points are selected through a greedy selection algorithm, which is computationally expensive. In our method, both the inducing points and hyperparameters of the experts are learned through maximizing an improved lower bound of the marginal likelihood. Experiments on benchmark datasets show the advantages of the proposed method.
MoreTranslated text
Key words
Gaussian process,variational inference
AI Read Science
Must-Reading Tree
Example
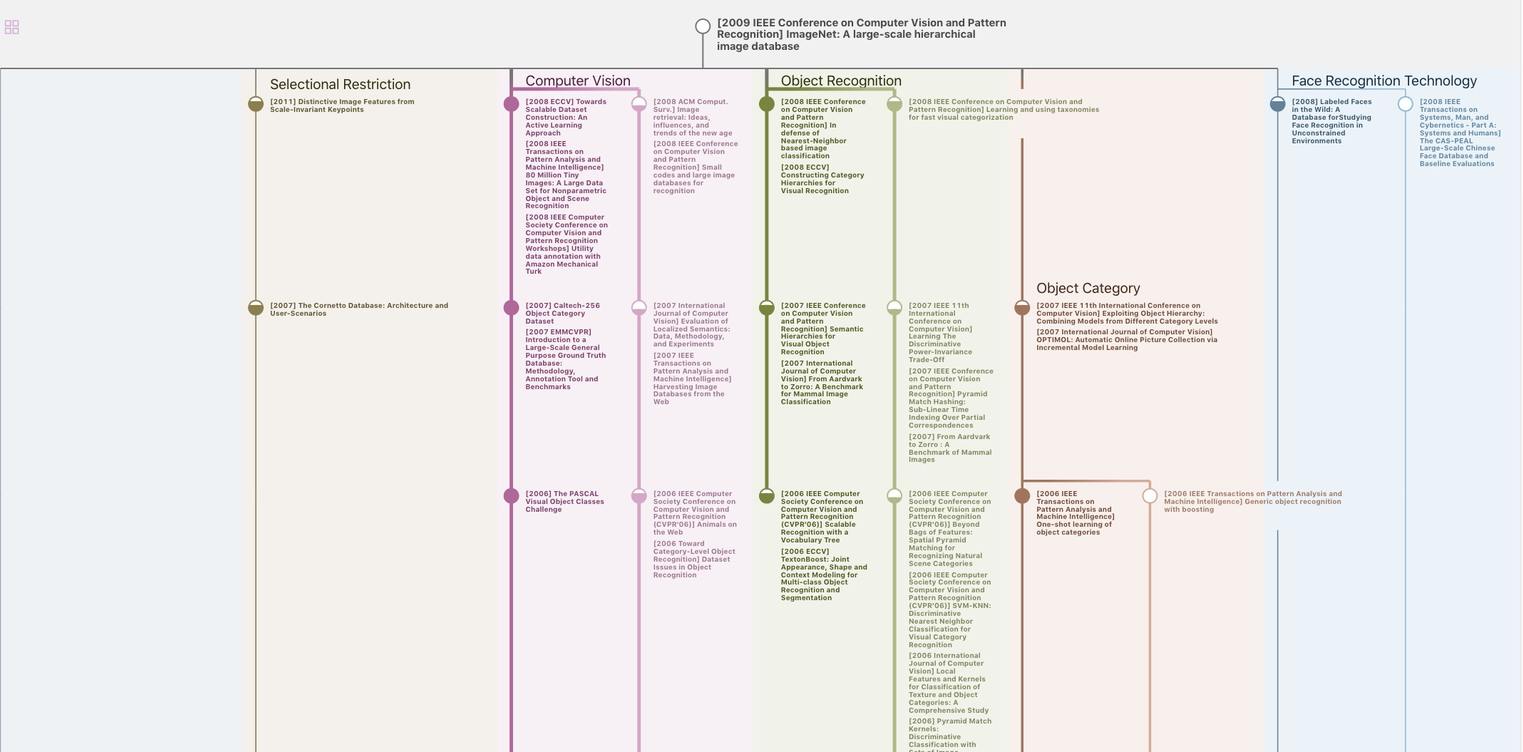
Generate MRT to find the research sequence of this paper
Chat Paper
Summary is being generated by the instructions you defined