Combining non-negative matrix factorization and deep neural networks for speech enhancement and automatic speech recognition.
2016 IEEE International Conference on Acoustics, Speech and Signal Processing (ICASSP)(2016)
摘要
Sparse Non-negative Matrix Factorization (SNMF) and Deep Neural Networks (DNN) have emerged individually as two efficient machine learning techniques for single-channel speech enhancement. Nevertheless, there are only few works investigating the combination of SNMF and DNN for speech enhancement and robust Automatic Speech Recognition (ASR). In this paper, we present a novel combination of speech enhancement components based-on SNMF and DNN into a full-stack system. We refine the cost function of the DNN to back-propagate the reconstruction error of the enhanced speech. Our proposal is compared with several state-of-the-art speech enhancement systems. Evaluations are conducted on the data of CHiME-3 challenge which consists of real noisy speech recordings captured under challenging noisy conditions. Our system yields significant improvements for both objective quality speech enhancement measurements with relative gain of 30%, and a 10% relative Word Error Rate reduction for ASR compared to the best baselines.
更多查看译文
关键词
Speech Enhancement, Automatic Speech Recognition, Non Negative Matrix Factorization, Deep Neural Network, CHiME-3 challenge
AI 理解论文
溯源树
样例
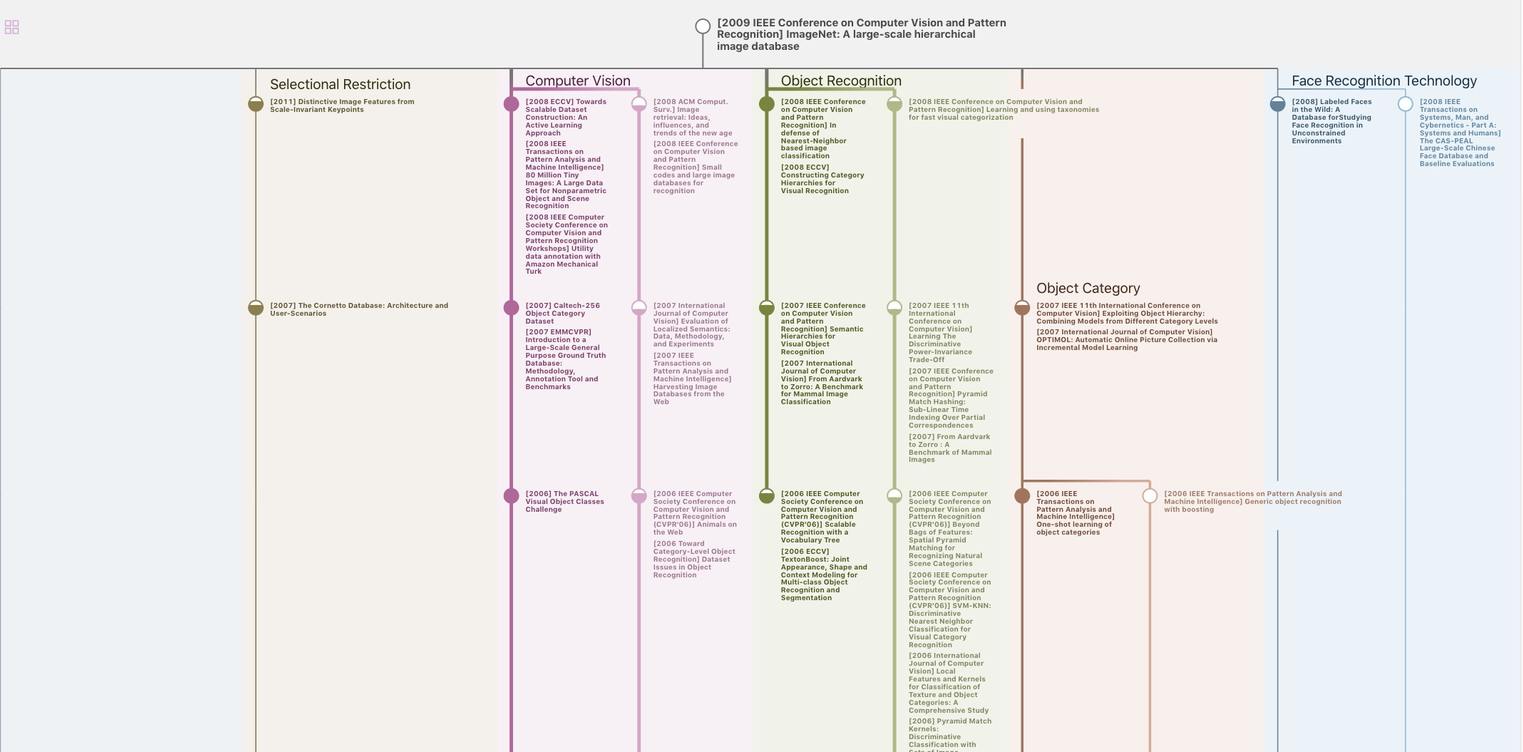
生成溯源树,研究论文发展脉络
Chat Paper
正在生成论文摘要