Variational Bayesian Image Fusion Based On Combined Sparse Representations
2016 IEEE International Conference on Acoustics, Speech and Signal Processing (ICASSP)(2016)
摘要
Hyper-spectral image fusion has been a hot topic in medical imaging and remote sensing. This paper proposes a Bayesian fusion model which combines the panchromatic (PAN) image and the low spatial resolution hyper-spectral (HS) image under the same framework. Sparsity constraint is introduced as double "spike-and-slab" priors, and anisotropic Gaussian noise is adopted for accuracy. To achieve reduction in computational complexity, we turn the anisotropic Gaussian distribution into isotropic one with modified linear transformation and propose a variational Bayesian expectation maximization (EM) algorithm to calculate the result. Experiment results show that our solution can achieve comparable performance in pan-sharpening to other state-of-art algorithms while largely reducing the computational complexity.
更多查看译文
关键词
Hyper-spectral image fusion,sparse prior,computational complexity,anisotropic Gaussian distribution,variational Bayesian algorithm
AI 理解论文
溯源树
样例
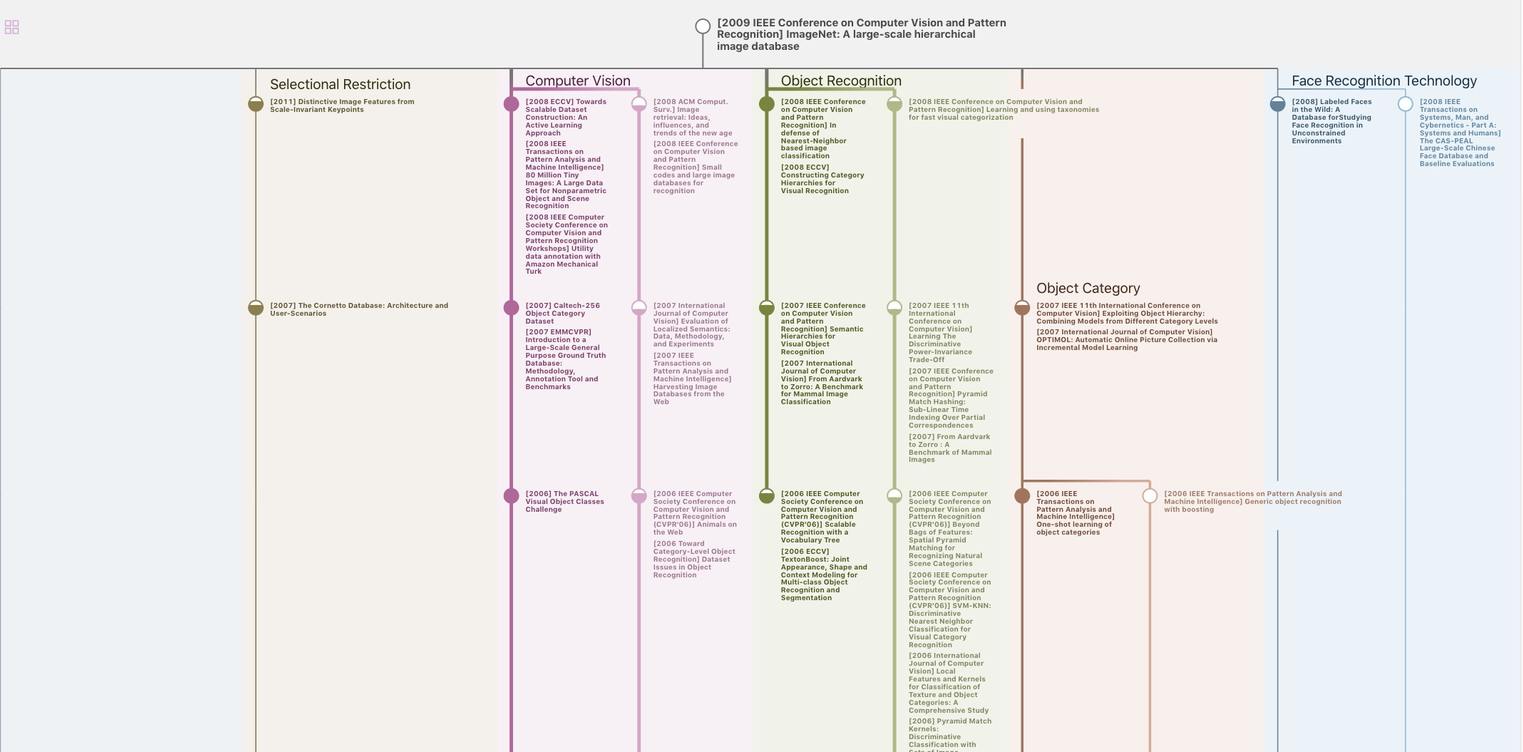
生成溯源树,研究论文发展脉络
Chat Paper
正在生成论文摘要