Multi-Kernel Based Nonlinear Models For Connectivity Identification Of Brain Networks
2016 IEEE International Conference on Acoustics, Speech and Signal Processing (ICASSP)(2016)
摘要
Partial correlations (PCs) of functional magnetic resonance imaging (fMRI) time series play a principal role in revealing connectivity of brain networks. To explore nonlinear behavior of the blood-oxygen-level dependent signal, the present work postulates a kernel-based nonlinear connectivity model based on which it obtains topology revealing PCs. Instead of relying on a single predefined kernel, a data-driven approach is advocated to learn the combination of multiple kernel functions that optimizes the data fit. Synthetically generated data based on both a dynamic causal and a linear model are used to validate the proposed approach in resting-state fMRI scenarios, highlighting the gains in edge detection performance when compared with the popular linear PC method. Tests on real fMRI data demonstrate that connectivity patterns revealed by linear and nonlinear models are different.
更多查看译文
关键词
fMRI,partial correlation,kernel-based regression,multiple kernel learning
AI 理解论文
溯源树
样例
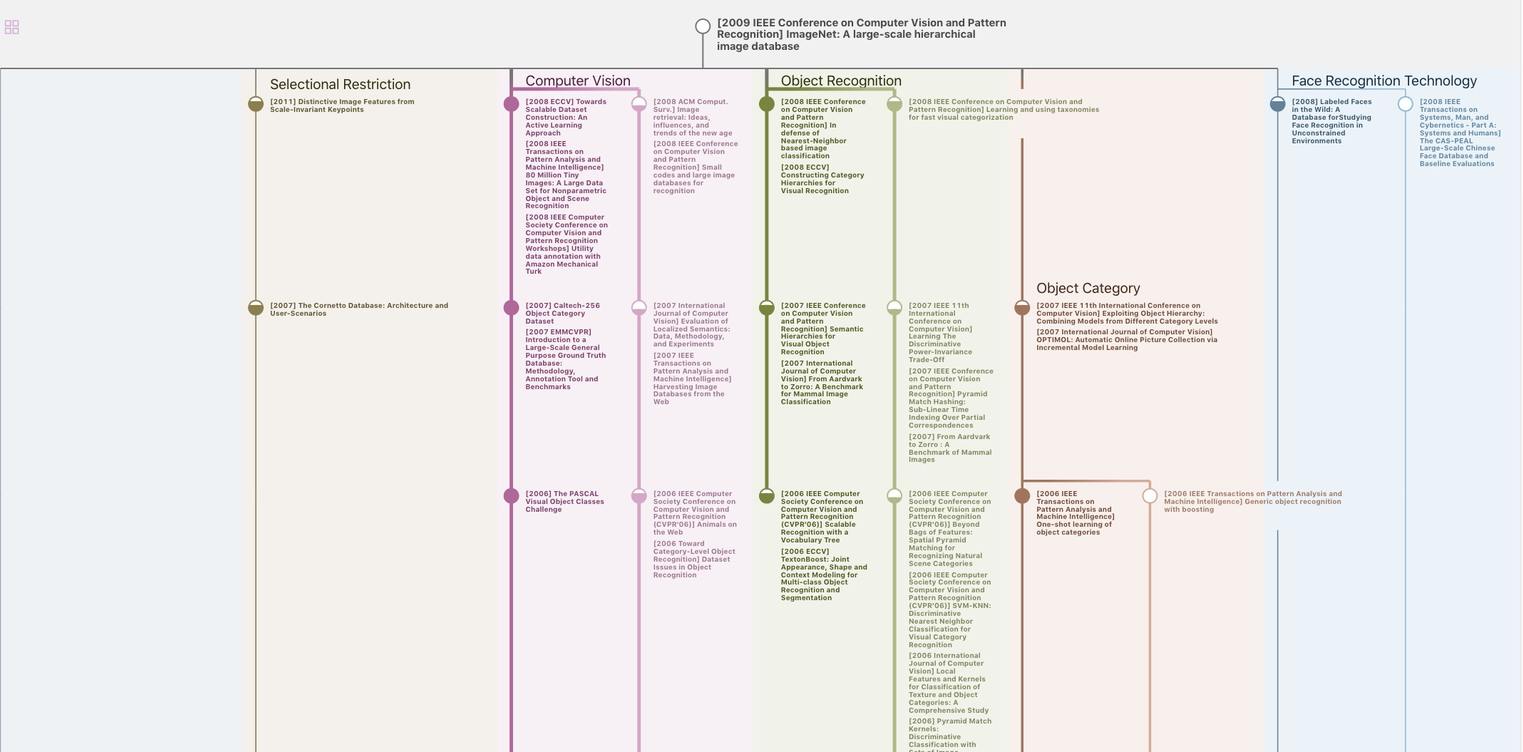
生成溯源树,研究论文发展脉络
Chat Paper
正在生成论文摘要