Kernelized Learning In Deep Scattering Convolution Networks
2016 IEEE International Conference on Multimedia and Expo (ICME)(2016)
摘要
This paper addresses the problem of automatic scattering feature selection for signal classification. While features derived from group invariant scattering networks are quite effective for signal classification. We argue that scattering networks are not always the appropriate choice as they are not learned for the objective at hand. In this paper, we explore jointly learning a deep scattering convolution network with a support vector machine by casting the problem as a multiple kernel learning problem. The convolution paths of the network are kernelized respectively to be selected in a large-margin context. We deduce scattering paths from the corresponding kernels after solving the kernel learning problem. Experiments on several datasets demonstrate the effectiveness of the proposed method over state-of-the-art techniques.
更多查看译文
关键词
wavelet filter,multiple kernel learning,scattering transform,digit analysis,texture recognition
AI 理解论文
溯源树
样例
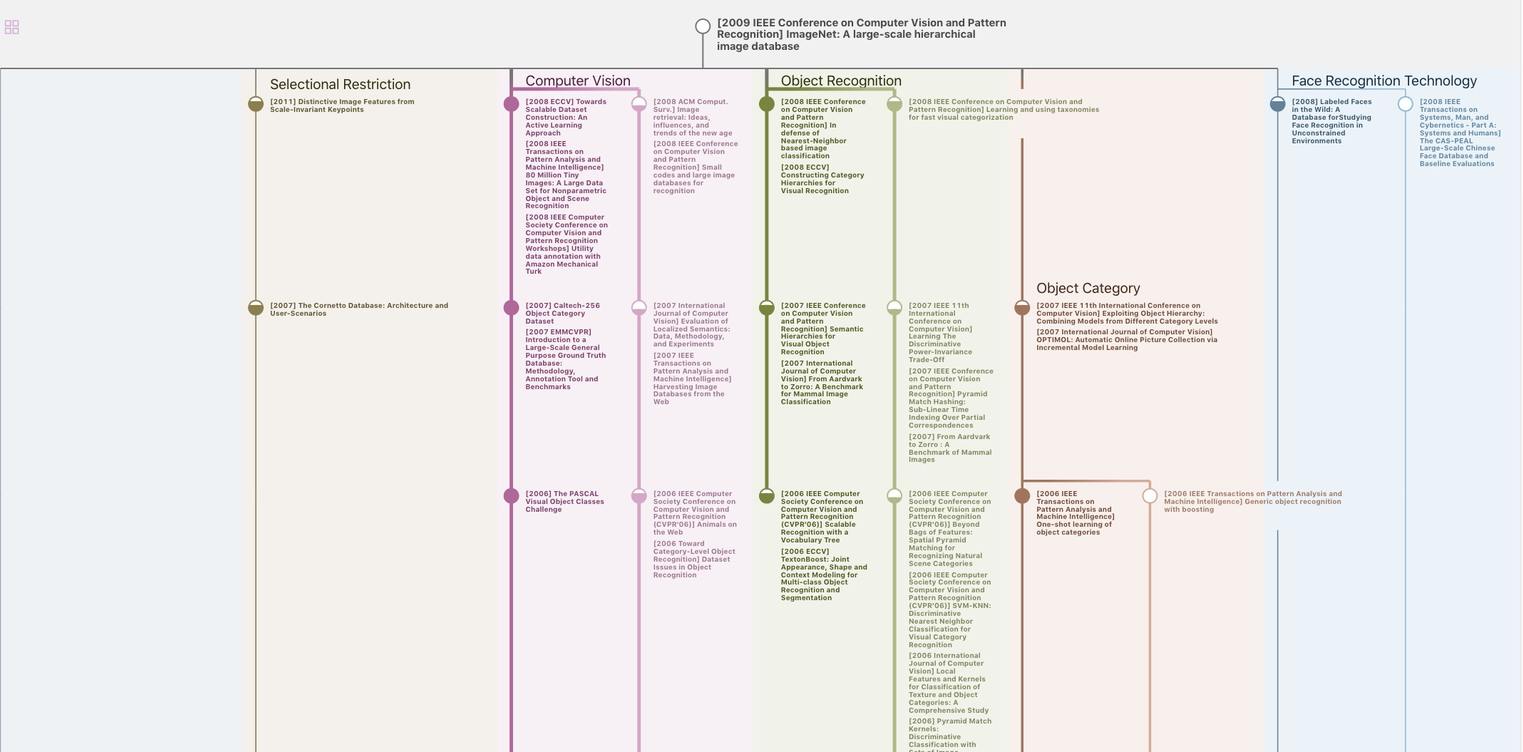
生成溯源树,研究论文发展脉络
Chat Paper
正在生成论文摘要