Detection of Mobile Malware: An Artificial Immunity Approach
2016 IEEE Security and Privacy Workshops (SPW)(2016)
摘要
Inspired by the human immune system, we explore the development of a new multiple detector set artificial immune system (mAIS) for the detection of mobile malware based on the information flows in Android apps. mAISs differ from conventional AISs in that multiple detector sets are evolved concurrently via negative selection. Typically, the first detector set is composed of detectors that match information flows associated with malicious apps while the second detector set is composed of detectors that match the information flows associated with benign apps. The mAIS presented in this paper incorporates feature selection along with a negative selection technique known as the split detector method (SDM). This new mAIS has been compared with a variety of conventional AISs and mAISs using a dataset of information flows captured from malicious and benign Android applications. Our preliminary results show that the newly designed mAIS outperforms the conventional AISs and mAISs in terms of accuracy and false positive rate of malware detection. This paper ends with a discussion of how mAISs can be used to solve dynamic cybersecurity problems as well as a discussion of our future research. This approach achieved 93.33% accuracy with a 0.00% false positive rate.
更多查看译文
关键词
mobile malware,static flow analysis,artificial immunity,negative selection,feature selection
AI 理解论文
溯源树
样例
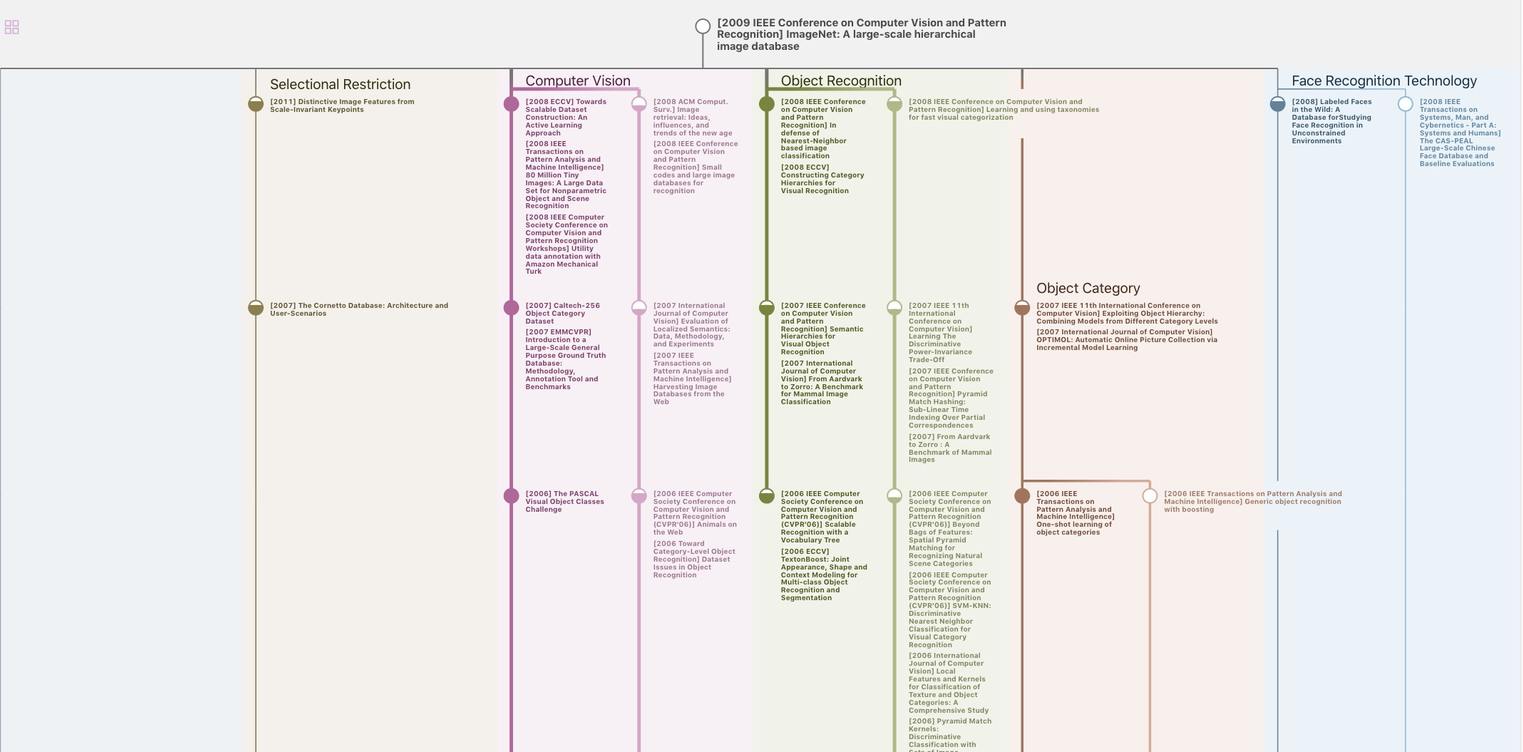
生成溯源树,研究论文发展脉络
Chat Paper
正在生成论文摘要