Predicting Pedestrian Crossing Using Quantile Regression Forests
2016 IEEE INTELLIGENT VEHICLES SYMPOSIUM (IV)(2016)
摘要
Future automated driving systems will require a comprehensive scene understanding. Considering these systems in an urban environment it becomes immediately clear that reasoning about the future behavior and trajectories of pedestrians represents one major challenge. In this paper we focus on predicting the pedestrians' time-to-cross when approaching a crosswalk. Due to the complexity of the underlying model, we propose a data-driven approach that by means of regression models learns the target variable. Instead of utilizing a standard mean regression, we propose the use of Quantile Regression. We show that this special type of regression is more suited to describe the variability of real world pedestrian trajectories. We examine and compare two approaches: Linear Quantile Regression and Quantile Regression Forest, which is an extended version of Random Forests. We present evaluations with real data and a detailed analysis emphasizing strengths and weaknesses of quantile regression for the target application.
更多查看译文
关键词
automated driving systems,scene understanding,urban environment,pedestrian behavior,pedestrian trajectories,pedestrian time-to-cross prediction,crosswalk,data-driven approach,linear quantile regression,quantile regression forest,random forests
AI 理解论文
溯源树
样例
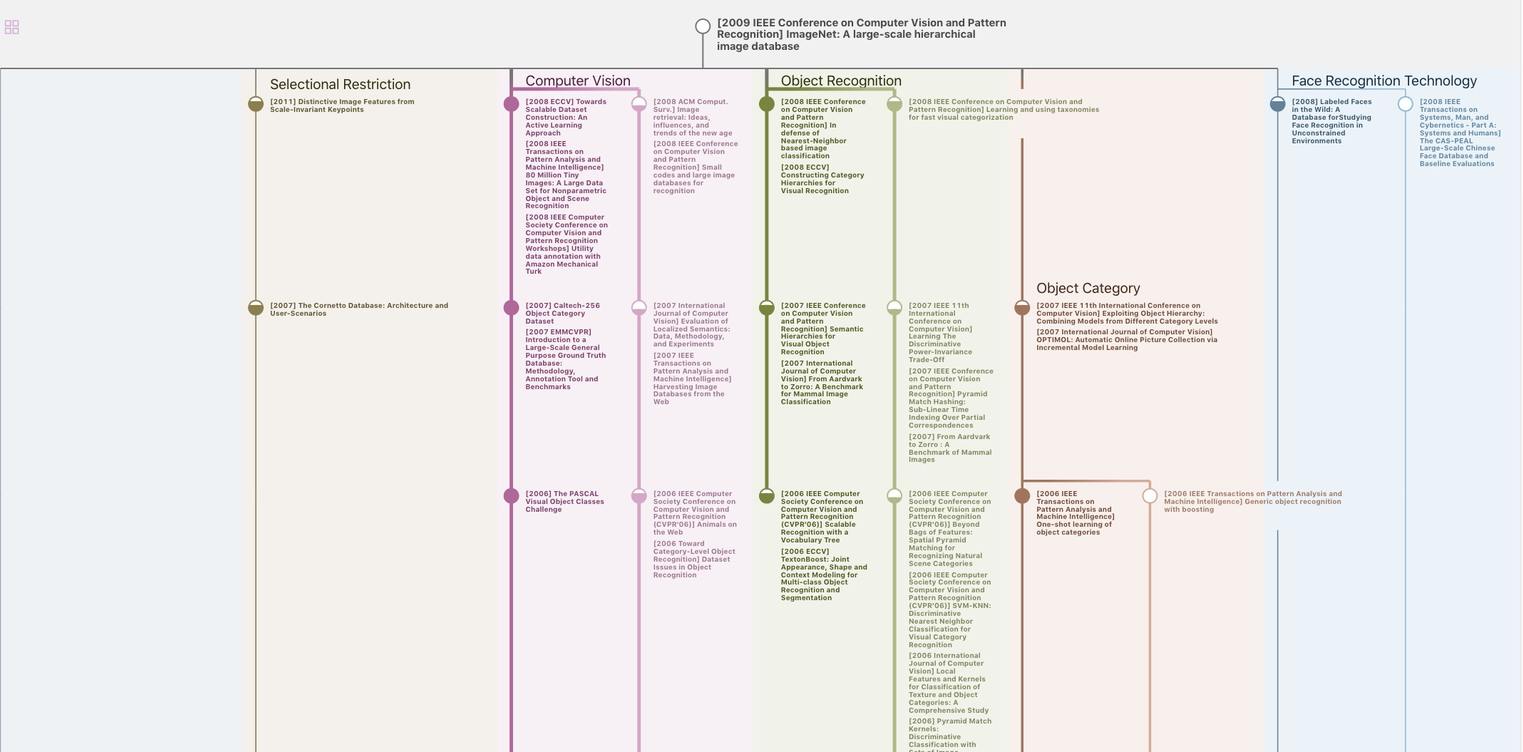
生成溯源树,研究论文发展脉络
Chat Paper
正在生成论文摘要