Semantic Stixels: Depth Is Not Enough
2016 IEEE Intelligent Vehicles Symposium (IV)(2016)
摘要
In this paper we present Semantic Stixels, a novel vision-based scene model geared towards automated driving. Our model jointly infers the geometric and semantic layout of a scene and provides a compact yet rich abstraction of both cues using Stixels as primitive elements. Geometric information is incorporated into our model in terms of pixel-level disparity maps derived from stereo vision. For semantics, we leverage a modern deep learning-based scene labeling approach that provides an object class label for each pixel.Our experiments involve an in-depth analysis and a comprehensive assessment of the constituent parts of our approach using three public benchmark datasets. We evaluate the geometric and semantic accuracy of our model and analyze the underlying run-times and the complexity of the obtained representation. Our results indicate that the joint treatment of both cues on the Semantic Stixel level yields a highly compact environment representation while maintaining an accuracy comparable to the two individual pixel-level input data sources. Moreover, our framework compares favorably to related approaches in terms of computational costs and operates in real-time.
更多查看译文
关键词
vision-based scene model,automated driving,pixel-level disparity maps,stereo vision,modern deep learning-based scene labeling approach,semantic Stixel level
AI 理解论文
溯源树
样例
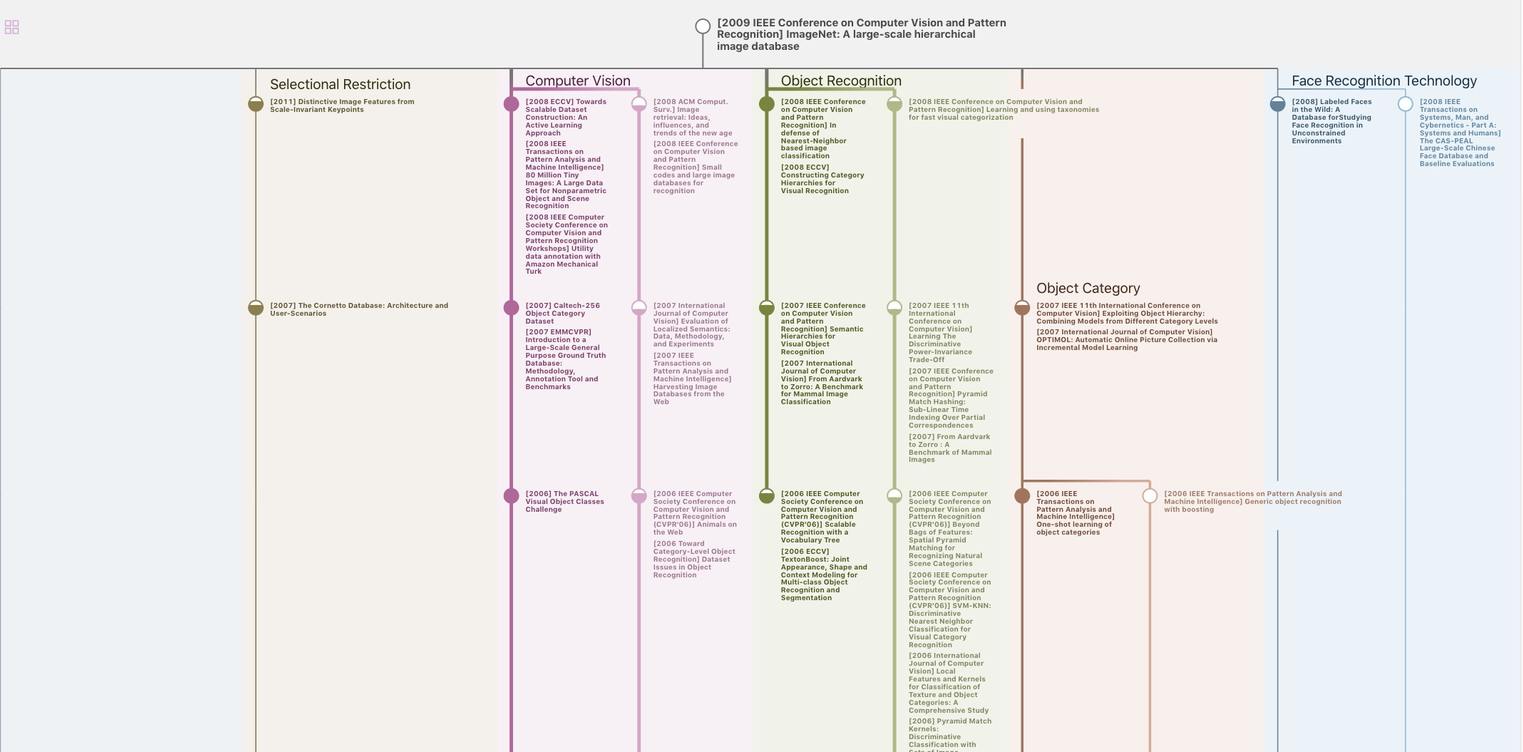
生成溯源树,研究论文发展脉络
Chat Paper
正在生成论文摘要