Appearance-Based Brake-Lights Recognition Using Deep Learning And Vehicle Detection
2016 IEEE Intelligent Vehicles Symposium (IV)(2016)
摘要
Vehicle following is one of the fundamental functions of an autonomous driving system. Detection and recognition of tail light signal is important to prevent an autonomous vehicle from rear-end collisions or accidents. Although sensors like acoustic sonar or commercialized Advanced Driving Assistance System (ADAS) products such as mobileye could be used for rear-end collision warning, a cost-effective approach is expected. In this paper, we have developed a novel two-stage approach to detect vehicles and recognize brake lights from a single image in real-time. Unlike previous approaches where pair taillight has to be extracted explicitly, we use vehicle rear appearance image instead. On a large database, "Brake Lights Patterns" (BLP) are learned by a multi-layer perception neural network. Given an image, the vehicles can be classified as "brake" or "normal" using the deep classifier. The vehicle can be detected quickly and robustly by combining multi-layer lidar (IBEO Lux fusion system) and a camera. Road segmentation and a novel vanishing point region of interest (ROI) determination method are explored to further speed up the detection and improve the robustness. The experimental results conducted on some real on-road videos have shown the robustness and efficiency of the proposed approach.
更多查看译文
关键词
appearance-based brake-light recognition,deep learning,vehicle detection,vehicle following,autonomous driving system,tail light signal detection,tail light signal recognition,autonomous vehicle,rear-end collision prevention,accident prevention,brake light recognition,vehicle rear appearance image,brake lights patterns,BLP,multilayer perception neural network,vehicle classification,deep classifier,multilayer LIDAR,IBEO Lux fusion system,camera,road segmentation,vanishing point region of interest determination method,ROI determination method,on-road videos
AI 理解论文
溯源树
样例
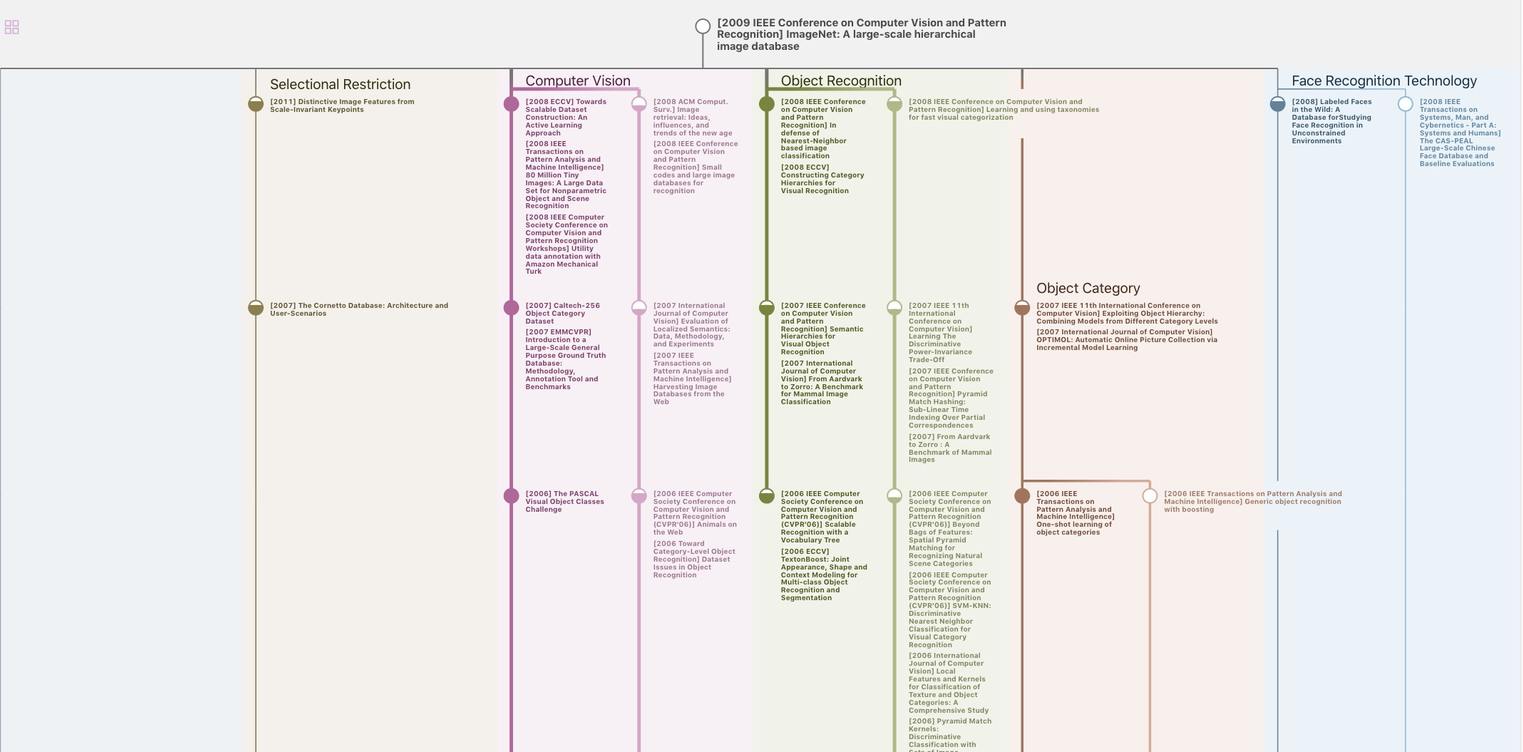
生成溯源树,研究论文发展脉络
Chat Paper
正在生成论文摘要