Generating Task-Pertinent sorted Error Lists for Speech Recognition.
LREC 2016 - TENTH INTERNATIONAL CONFERENCE ON LANGUAGE RESOURCES AND EVALUATION(2016)
摘要
Automatic Speech recognition (ASR) is one of the most widely used components in spoken language processing applications. ASR errors are of varying importance with respect to the application, making error analysis keys to improving speech processing applications. Knowing the most serious errors for the applicative case is critical to build better systems. In the context of Automatic Speech Recognition (ASR) used as a first step towards Named Entity Recognition (NER) in speech, error seriousness is usually determined by their frequency, due to the use of the WER as metric to evaluate the ASR output, despite the emergence of more relevant measures in the literature. We propose to use a different evaluation metric form the literature in order to classify ASR errors according to their seriousness for NER. Our results show that the ASR errors importance is ranked differently depending on the used evaluation metric. A more detailed analysis shows that the estimation of the error impact given by the ATENE metric is more adapted to the NER task than the estimation based only on the most used frequency metric WER.
更多查看译文
关键词
Automatic Speech Recognition,Metrics,Error Analysis
AI 理解论文
溯源树
样例
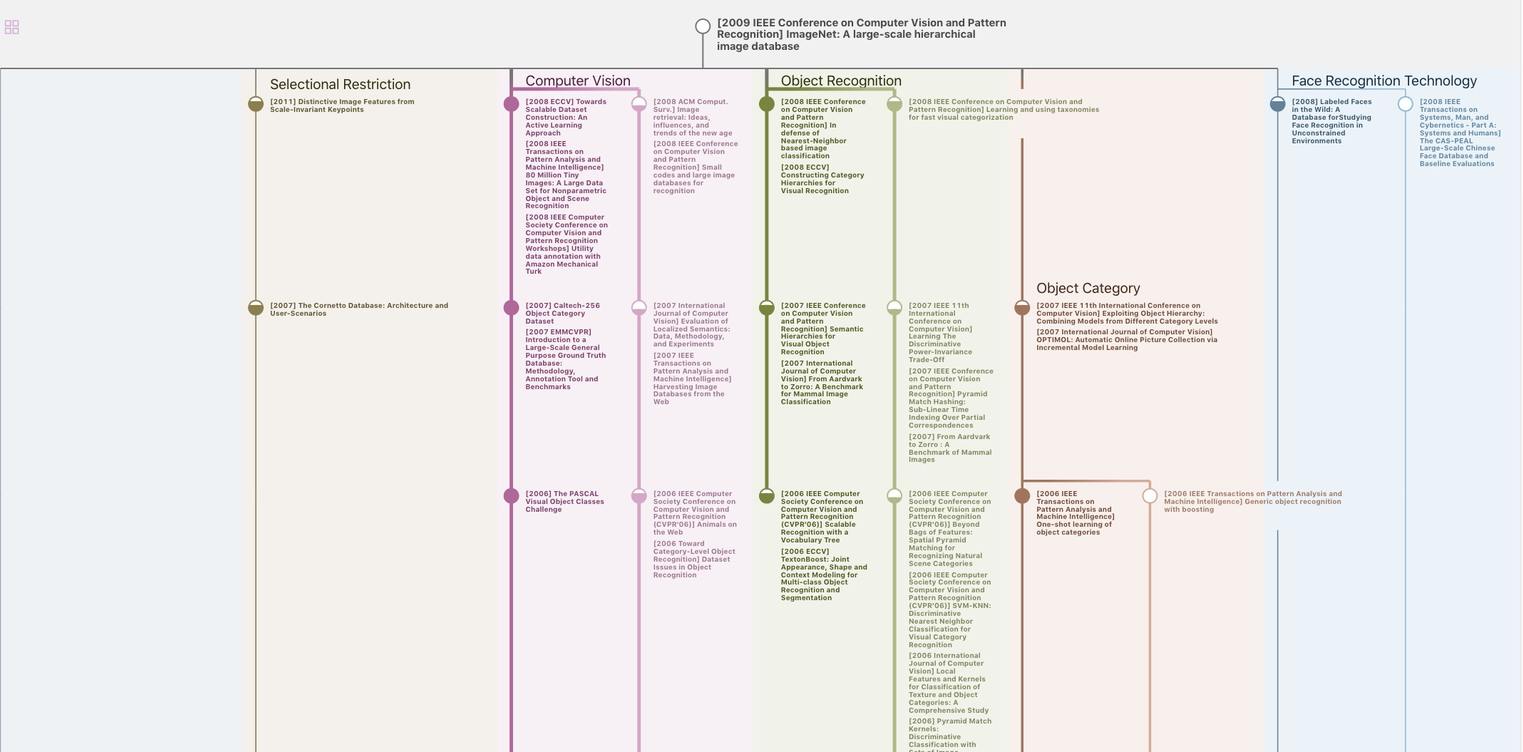
生成溯源树,研究论文发展脉络
Chat Paper
正在生成论文摘要