Half Hypersphere Confinement For Piecewise Linear Regression
2016 IEEE Winter Conference on Applications of Computer Vision (WACV)(2016)
摘要
Recent research in piecewise linear regression for Super-Resolution has shown the positive impact of training regressors with densely populated clusters whose datapoints are tight in the Euclidean space. In this paper we further research how to improve the locality condition during the training of regressors and how to better select them during testing time. We study the characteristics of the metrics best suited for the piecewise regression algorithms, in which comparisons are usually made between normalized vectors that lie on the unitary hypersphere. Even though Euclidean distance has been widely used for this purpose, it is suboptimal since it does not handle antipodal points (i.e. diametrically opposite points) properly, as vectors with same module and angle but opposite directions are, for linear regression purposes, identical. Therefore, we propose the usage of antipodally invariant metrics and introduce the Half Hypersphere Confinement (HHC), a fast alternative to Multidimensional Scaling (MDS) that allows to map antipodally invariant distances in the Euclidean space with very little approximation error. By doing so, we enable the usage of fast search structures based on Euclidean distances without undermining their speed gains with complex distance transformations. The performance of our method, which we named HHC Regression (HHCR), applied to Super-Resolution (SR) improves both in quality (PSNR) and it is faster than any other state-of-the-art method. Additionally, under an application-agnostic interpretation of our regression framework, we also test our algorithm for denoising and depth upscaling with promising results.
更多查看译文
关键词
half hypersphere confinement,piecewise linear regression,superresolution,densely populated clusters,Euclidean space,normalized vectors,unitary hypersphere,antipodal points,diametrically opposite points,antipodally invariant metrics,antipodally invariant distances,HHC regression,denoising,depth upscaling
AI 理解论文
溯源树
样例
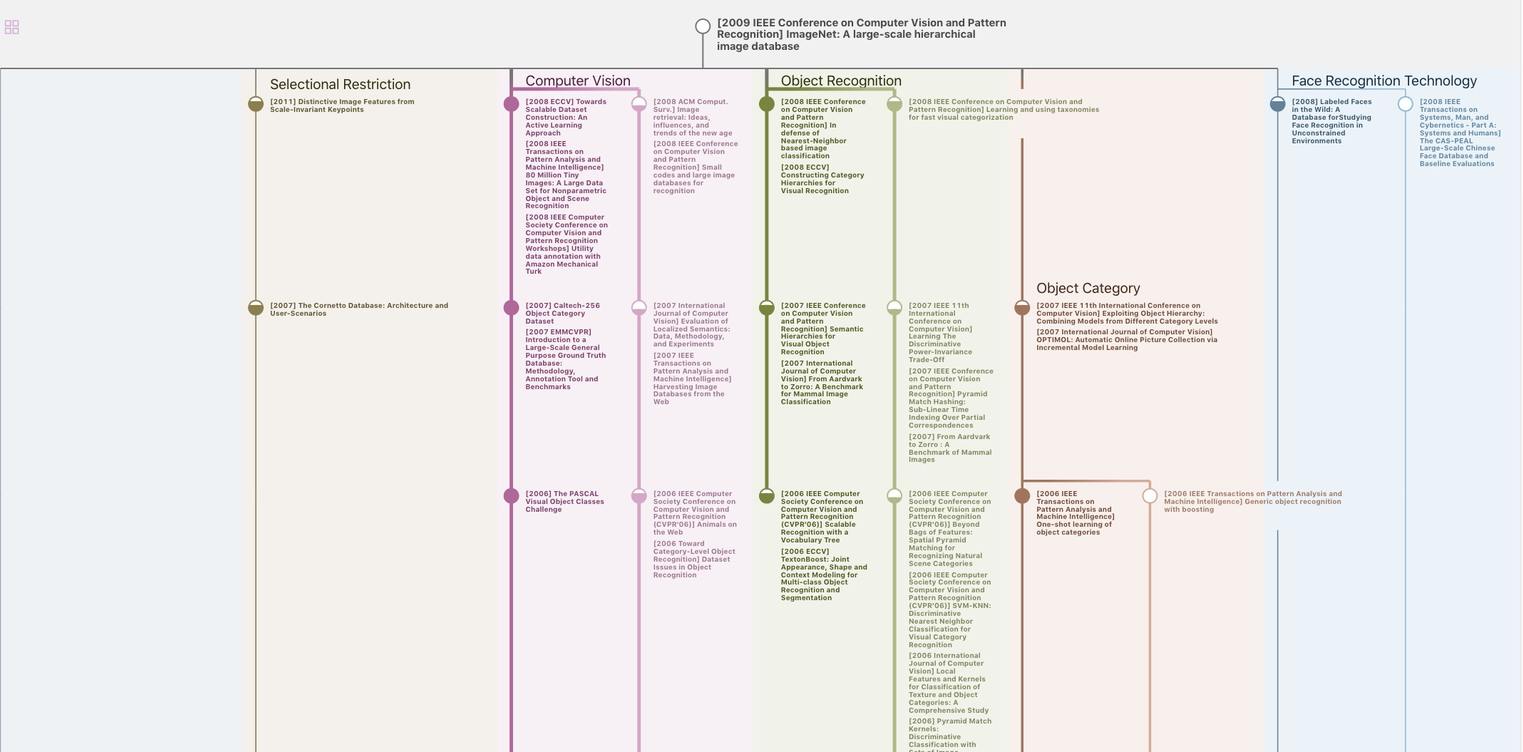
生成溯源树,研究论文发展脉络
Chat Paper
正在生成论文摘要