3D shape retrieval using a single depth image from low-cost sensors
2016 IEEE WINTER CONFERENCE ON APPLICATIONS OF COMPUTER VISION (WACV 2016)(2016)
摘要
Content-based 3D shape retrieval is an important problem in computer vision. Traditional retrieval interfaces require a 2D sketch or a manually designed 3D model as the query, which is difficult to specify and thus not practical in real applications. With the recent advance in low-cost 3D sensors such as Microsoft Kinect and Intel Realsense, capturing depth images that carry 3D information is fairly simple, making shape retrieval more practical and user-friendly. In this paper, we study the problem of cross-domain 3D shape retrieval using a single depth image from low-cost sensors as the query to search for similar human designed CAD models. We propose a novel method using an ensemble of autoencoders in which each autoencoder is trained to learn a compressed representation of depth views synthesize d from each database object. By viewing each autoencoder as a probabilistic model, a likelihood score can be derived as a similarity measure. A domain adaptation layer is built on top of autoencoder outputs to explicitly address the cross-domain issue (between noisy sensory data and clean 3D models) by incorporating training data of sensor depth images and their category labels in a weakly supennsed learning formulation. Experiments using real-world depth images and a large-scale CAD dataset demonstrate the effectiveness of our approach, which offers significant improvements over state-of-the-art 3D shape retrieval methods.
更多查看译文
关键词
single depth image,low-cost sensors,content-based 3D shape retrieval,computer vision,2D sketch,3D model,query,3D information,user-friendly,human designed CAD models,compressed representation,probabilistic model,supervised learning,large-scale CAD dataset
AI 理解论文
溯源树
样例
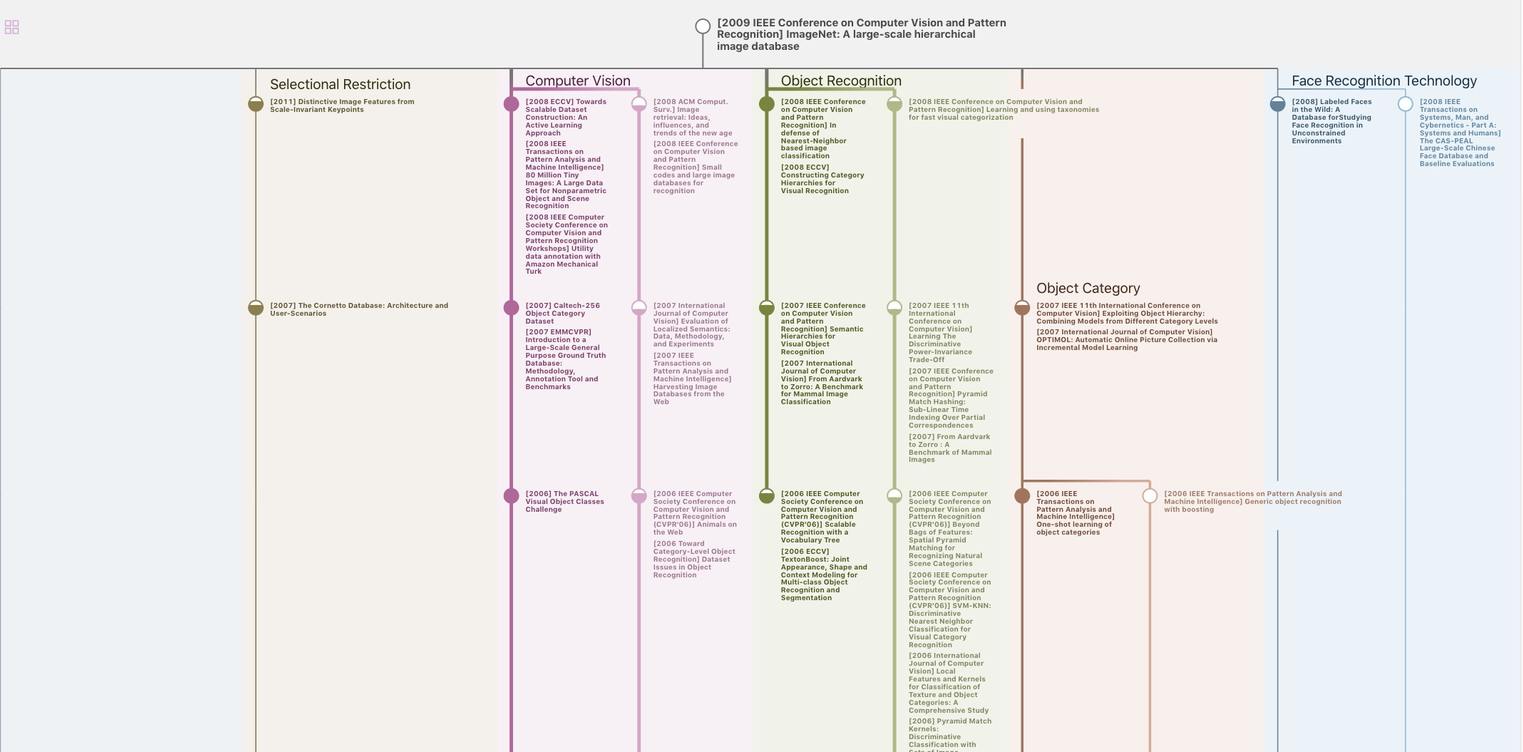
生成溯源树,研究论文发展脉络
Chat Paper
正在生成论文摘要