Unsupervised Network Pretraining via Encoding Human Design
2016 IEEE Winter Conference on Applications of Computer Vision (WACV)(2016)
摘要
Over the years, computer vision researchers have spent an immense amount of effort on designing image features for the visual object recognition task. We propose to incorporate this valuable experience to guide the task of training deep neural networks. Our idea is to pretrain the network through the task of replicating the process of hand-designed feature extraction. By learning to replicate the process, the neural network integrates previous research knowledge and learns to model visual objects in a way similar to the hand-designed features. In the succeeding finetuning step, it further learns object-specific representations from labeled data and this boosts its classification power. We pretrain two convolutional neural networks where one replicates the process of histogram of oriented gradients feature extraction, and the other replicates the process of region covariance feature extraction. After finetuning, we achieve substantially better performance than the baseline methods.
更多查看译文
关键词
unsupervised network pretraining,encoding human design,computer vision researchers,image features,visual object recognition task,deep neural networks,hand-designed feature extraction,object-specific representations,labeled data,classification power,convolutional neural networks,region covariance feature extraction
AI 理解论文
溯源树
样例
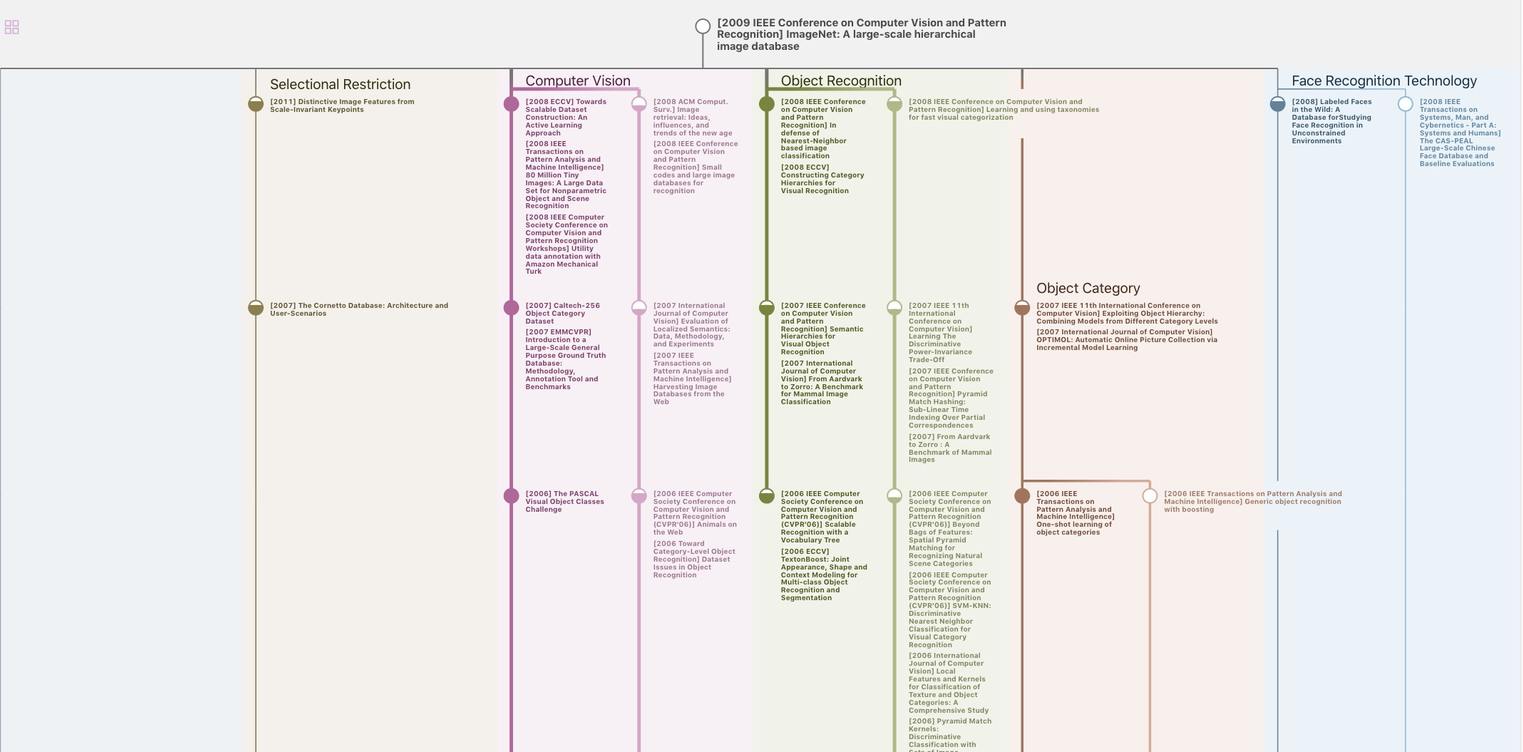
生成溯源树,研究论文发展脉络
Chat Paper
正在生成论文摘要