Generating Unsupervised Models For Online Long-Term Daily Living Activity Recognition
PROCEEDINGS 3RD IAPR ASIAN CONFERENCE ON PATTERN RECOGNITION ACPR 2015(2015)
摘要
This paper presents an unsupervised approach for learning long-term human activities without requiring any user interaction (e.g., clipping long-term videos into short-term actions, labeling huge amount of short-term actions as in supervised approaches). First, important regions in the scene are learned via clustering trajectory points and the global movement of people is presented as a sequence of primitive events. Then, using local action descriptors with bag-of-words (BoW) approach, we represent the body motion of people inside each region. Incorporating global motion information with action descriptors, a comprehensive representation of human activities is obtained by creating models that contains both global and body motion of people. Learning of zones and the construction of primitive events is automatically performed. Once models are learned, the approach provides an online recognition framework. We have tested the performance of our approach on recognizing activities of daily living and showed its efficiency over existing approaches.
更多查看译文
关键词
unsupervised model generation,online long-term daily living activity recognition,long-term human activity learning,clustering trajectory points,people global movement,local action descriptors,bag-of-words,body motion representation,human activity representation,zone learning,primitive event construction
AI 理解论文
溯源树
样例
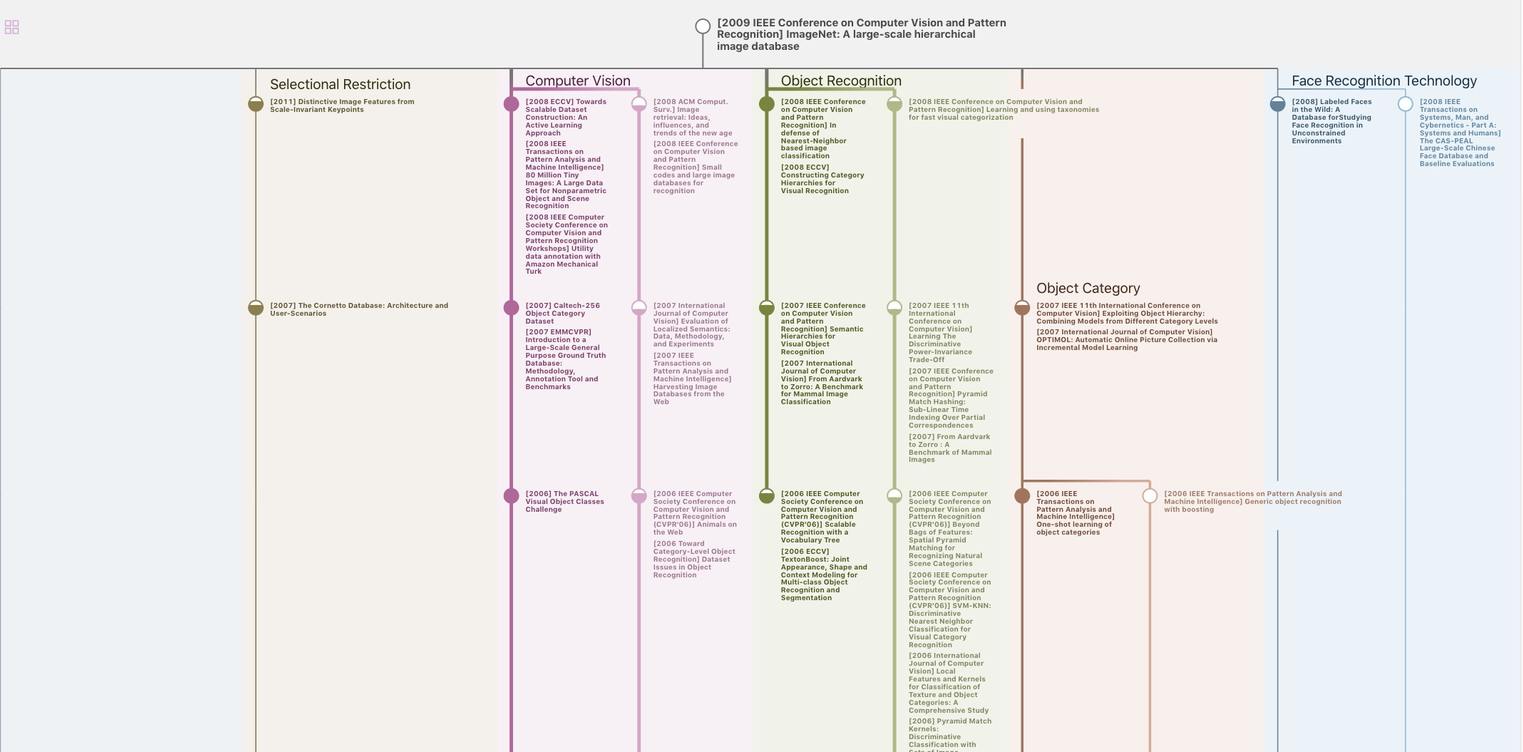
生成溯源树,研究论文发展脉络
Chat Paper
正在生成论文摘要