Interactive online learning for clinical entity recognition.
MOD(2016)
摘要
ABSTRACTNamed entity recognition and entity linking are core natural language processing components that are predominantly solved by supervised machine learning approaches. Such supervised machine learning approaches require manual annotation of training data that can be expensive to compile. The applicability of supervised, machine learning-based entity recognition and linking components in real-world applications can be hindered by the limited availability of training data. In this paper, we propose a novel approach that uses ontologies as a basis for entity recognition and linking, and captures context of neighboring tokens of the entities of interest with vectors based on syntactic and semantic features. Our approach takes user feedback so that the vector-based model can be continuously updated in an online setting. Here we demonstrate our approach in a healthcare context, using it to recognize body part and imaging modality entities within clinical documents, and map these entities to the right concepts in the RadLex and NCIT medical ontologies. Our current evaluation shows promising results on a small set of clinical documents with a precision and recall of 0.841 and 0.966. The evaluation also demonstrates that our approach is capable of continuous performance improvement with increasing size of examples. We believe that our human-in-the-loop, online learning approach to entity recognition and linking shows promise that it is suitable for real-world applications.
更多查看译文
AI 理解论文
溯源树
样例
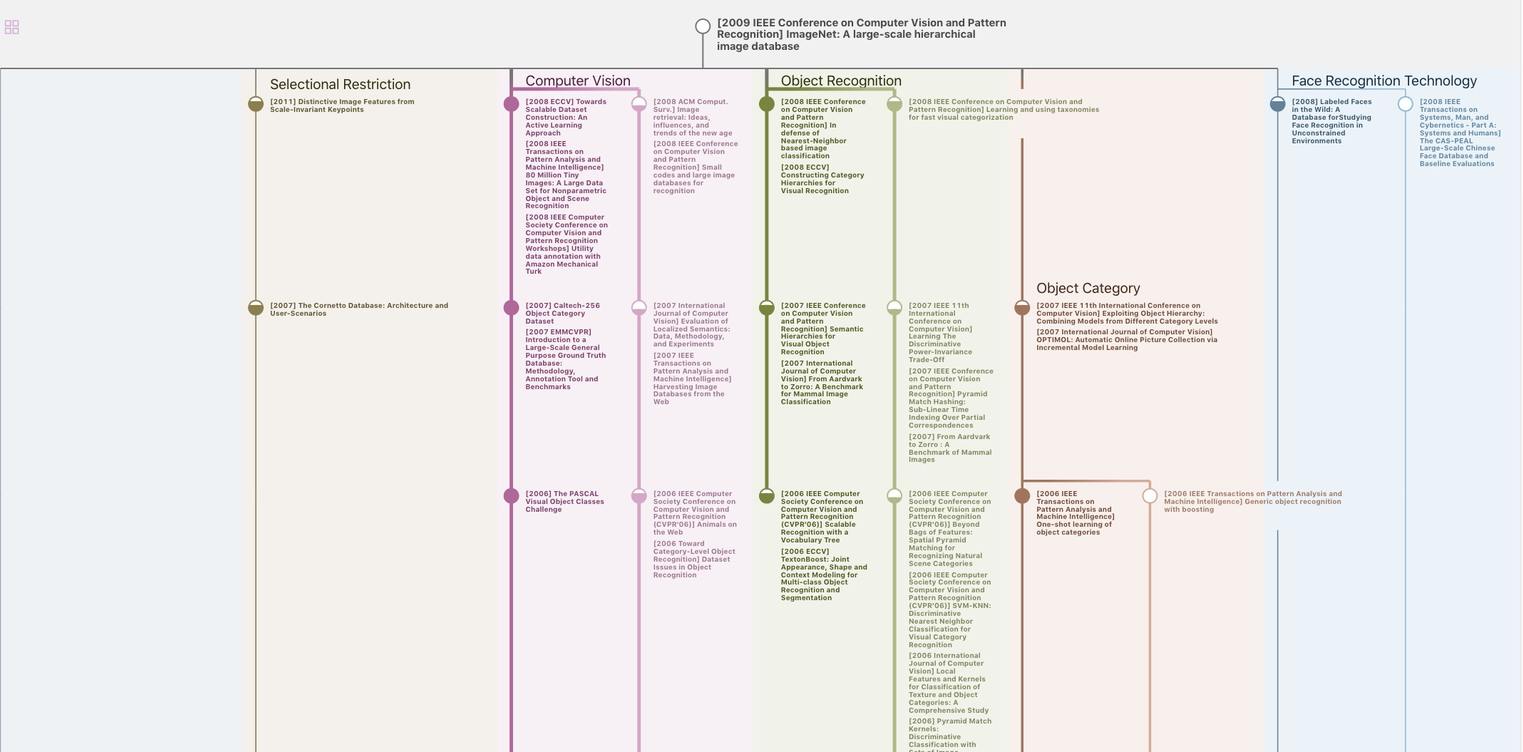
生成溯源树,研究论文发展脉络
Chat Paper
正在生成论文摘要