Variable Neighbourhood Descent With Memory: A Hybrid Metaheuristic For Supermarket Resupply
HYBRID METAHEURISTICS (HM 2016)(2016)
摘要
Supermarket supply chains represent an area in which optimisation of vehicle routes and scheduling can lead to huge cost and environmental savings. As just-in-time ordering practices become more common, traditionally fixed resupply routes and schedules are increasingly unable to meet the demands of the supermarkets. Instead, we model this as a dynamic pickup and delivery problem with soft time windows (PDPSTW). We present the variable neighbourhood descent with memory (VNDM) hybrid metaheuristic (HM) and compare its performance against Q-learning (QL), binary exponential back off (BEBO) and random descent (RD) hyperheuristics on published benchmark and real-world instances of the PDPSTW. We find that VNDM consistently generates the highest quality solutions, with the fewest routes or shortest distances, amongst the methods tested. It is capable of finding the best known solutions to 55 of 176 published benchmarks as well as producing the best results on our real-world data set, supplied by Transfaction Ltd.
更多查看译文
关键词
Variable Neighborhood Descent, Binary Exponential Back-off (BEBO), Random Descent (RD), Hyperheuristics, Real-world Data Sets
AI 理解论文
溯源树
样例
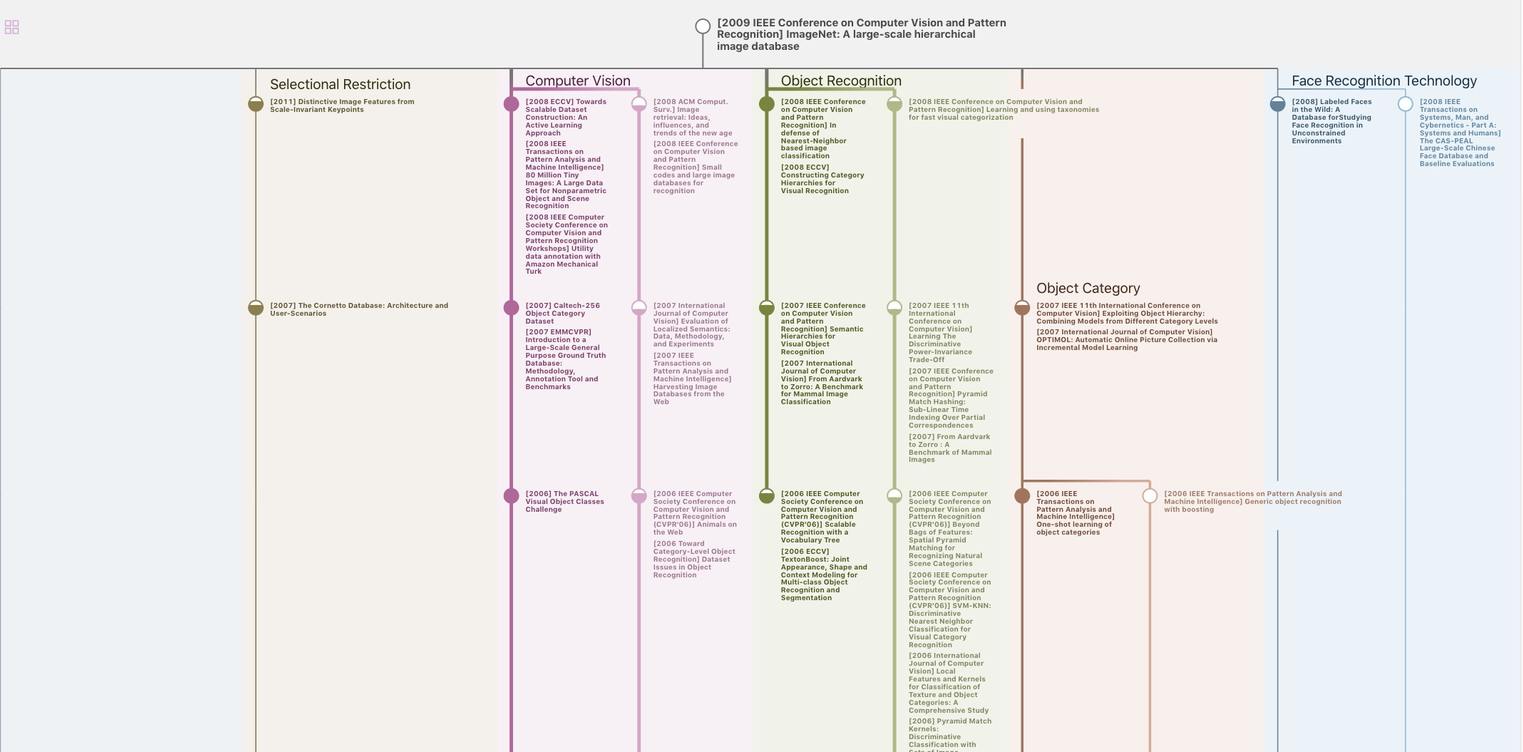
生成溯源树,研究论文发展脉络
Chat Paper
正在生成论文摘要