Predicting Early Signs of Dyslexia at a Preliterate Age by Combining Behavioral Assessment with Structural MRI.
NeuroImage(2016)
摘要
BACKGROUND:Recent studies suggest that neurobiological anomalies are already detectable in pre-school children with a family history of developmental dyslexia (DD). However, there is a lack of longitudinal studies showing a direct link between those differences at a preliterate age and the subsequent literacy difficulties seen in school. It is also not clear whether the prediction of DD in pre-school children can be significantly improved when considering neurobiological predictors, compared to models based on behavioral literacy precursors only.METHODS:We recruited 53 pre-reading children either with (N=25) or without a family risk of DD (N=28). Quantitative T1 MNI data and literacy precursor abilities were assessed at kindergarten age. A subsample of 35 children was tested for literacy skills either one or two years later, that is, either in first or second grade.RESULTS:The group comparison of quantitative T1 measures revealed significantly higher T1 intensities in the left anterior arcuate fascicle (AF), suggesting reduced myelin concentration in preliterate children at risk of DD. A logistic regression showed that DD can be predicted significantly better (p=.024) when neuroanatomical differences between groups are used as predictors (80%) compared to a model based on behavioral predictors only (63%). The Wald statistic confirmed that the T1 intensity of the left AF is a statistically significant predictor of DD (p<.05).CONCLUSIONS:Our longitudinal results provide evidence for the hypothesis that neuroanatomical anomalies in children with a family risk of DD are related to subsequent problems in acquiring literacy. Particularly, solid white matter organization in the left anterior arcuate fascicle seems to play a pivotal role.
更多查看译文
关键词
Developmental dyslexia,Arcuate fascicle,Cortical thickness,Quantitative T1,Diffusion-weighted imaging,Reading
AI 理解论文
溯源树
样例
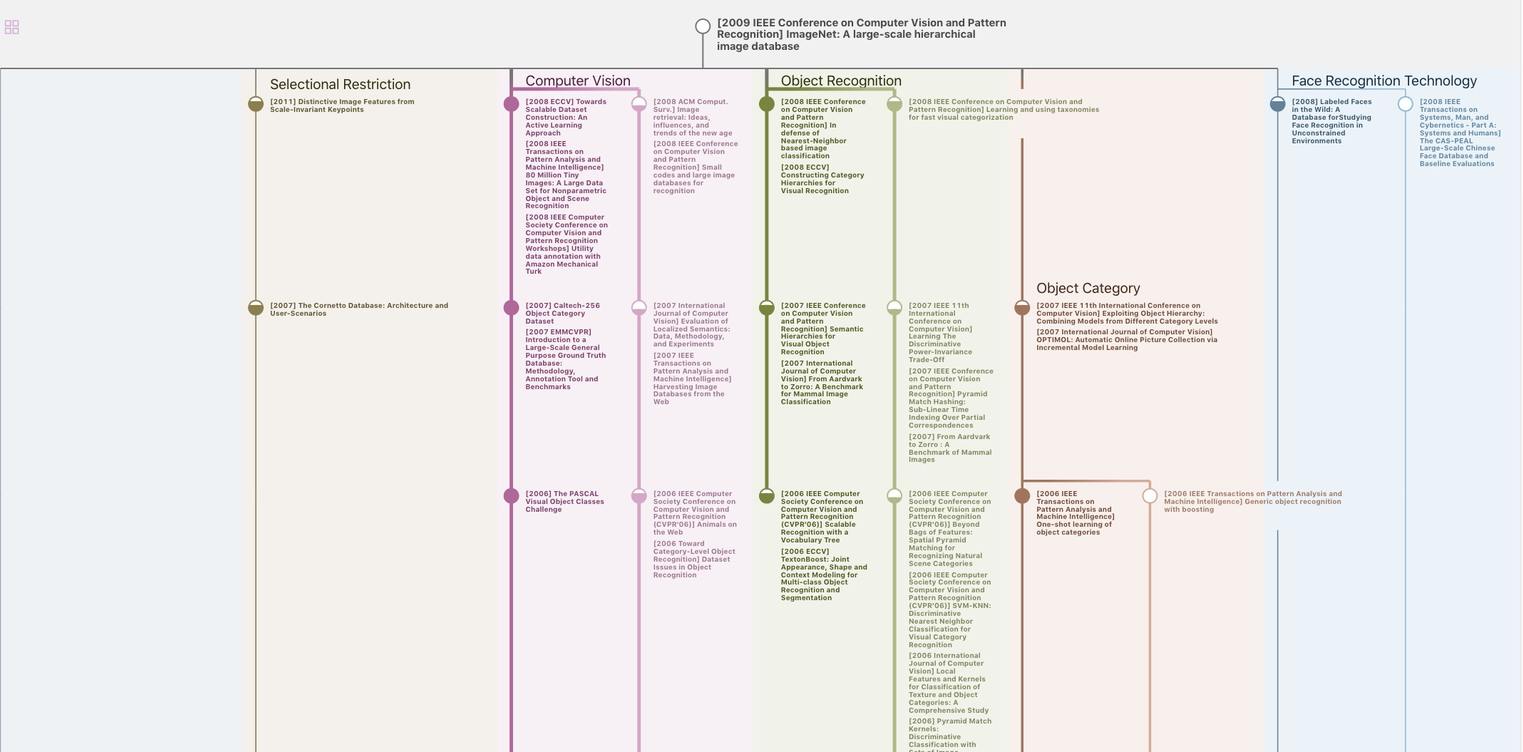
生成溯源树,研究论文发展脉络
Chat Paper
正在生成论文摘要