ZEST: A Hybrid Model on Predicting Passenger Demand for Chauffeured Car Service
ACM International Conference on Information and Knowledge Management(2016)
摘要
Chauffeured car service based on mobile applications like Uber or Didi offers an alternative for people to travel or commute. Like taxis, chauffeured car service also suffers from supply-demand disequilibrium. A proper prediction on the distribution of passenger demand can help with the decision of car dispatching and dynamic pricing to alleviate the disequilibrium and avoid misleading drivers into seeking passengers unproductively in zero-demand areas. Most of the existing studies were only focused on the strategies for individual passenger and driver, but lacked an global prediction on passenger demand especially on zero-demand areas, which also neglected the data bias between the picked up and the demanding passengers.
Therefore, in this paper, we propose a Zero-Grid Ensemble SpatioTemporal model (ZEST) to predict passenger demand with four predictors: a temporal predictor and a spatial predictor to model the influences of local and spatial factors separately, an ensemble predictor to combine the results of former two predictors comprehensively and a Zero-Grid predictor to predict zero-demand areas specifically. In temporal predictor which is based on local order data, a simple yet novel parameter FluctuationRate is proposed to reflect the similarity in the change of demand between historical and current time periods. In spatial predictor, the influence of neighborhood to the target region is modeled according to order and GPS data. We demonstrate the performance of ZEST based on actual operational data from car-hailing applications with more than 6 million order records and 500 million GPS points. Experimental results indicate our model achieves 0.68 in MAE and 12.6% in sMAPE on average, which outperforms 5 other baseline models by over 10% both in MAE and sMAPE on three-month datasets.
更多查看译文
关键词
Spatiotemporal Data Mining,Demand Prediction,Chauffeured Car Service
AI 理解论文
溯源树
样例
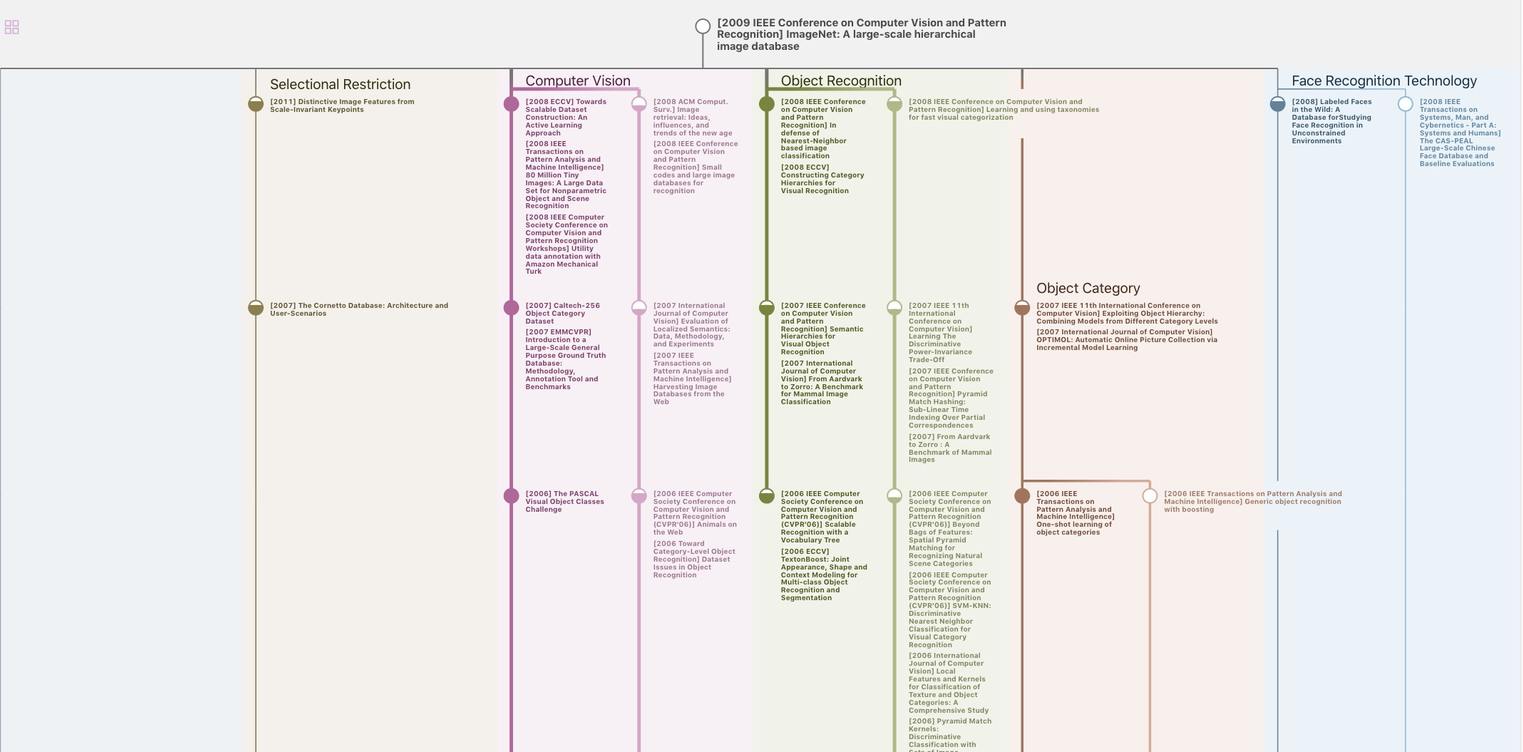
生成溯源树,研究论文发展脉络
Chat Paper
正在生成论文摘要