Near Real-time Geolocation Prediction in Twitter Streams via Matrix Factorization Based Regression
ACM International Conference on Information and Knowledge Management(2016)
摘要
Previous research on content-based geolocation in general has developed prediction methods via conducting pre-partitioning and applying classification methods. The input of these methods is the concatenation of individual tweets during a period of time. But unfortunately, these methods have some drawbacks. They discard the natural real-values properties of latitude and longitude as well as fail to capture geolocation in near real-time. In this work, we develop a novel generative content-based regression model via a matrix factorization technique to tackle the near real-time geolocation prediction problem. With this model, we aim to address a couple of un-answered questions. First, we prove that near real-time geolocation prediction can be accomplished if we leave out the concatenation. Second, we account the real-values properties of physical coordinates within a regression solution. We apply our model on Twitter datasets as an example to prove the effectiveness and generality. Our experimental results show that the proposed model outperforms a set of state-of-the-art regression models including Support Vector Machines and Factorization Machines by a reduction of localization error up to 79%.
更多查看译文
关键词
Geolocation,Matrix Factorization,Regression,Twitter
AI 理解论文
溯源树
样例
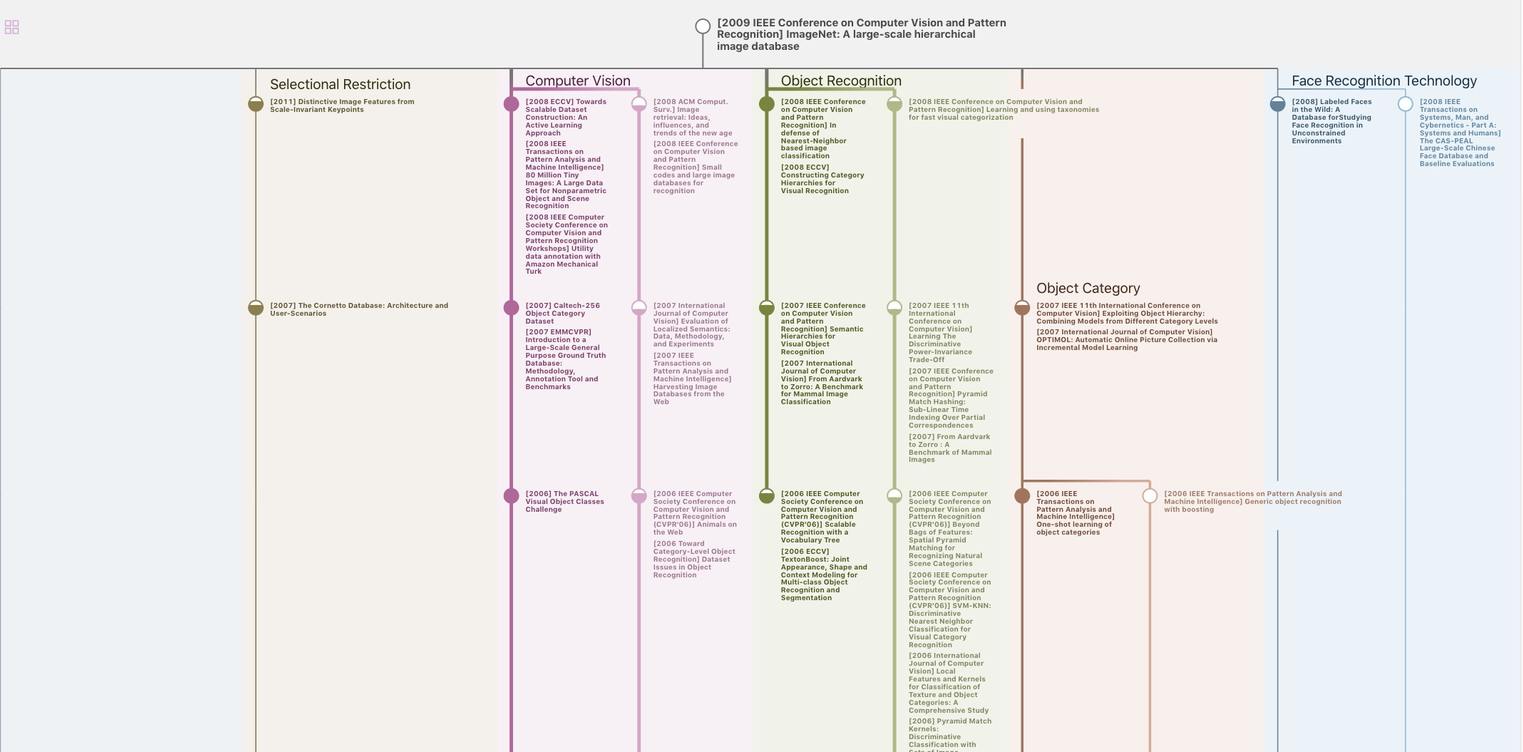
生成溯源树,研究论文发展脉络
Chat Paper
正在生成论文摘要