Adaptive Evolutionary Filtering in Real-Time Twitter Stream
ACM International Conference on Information and Knowledge Management(2016)
摘要
With the explosive growth of microblogging service, Twitter has become a leading platform consisting of real-time world wide information. Users tend to explore breaking news or general topics in Twitter according to their interests. However, the explosive amount of incoming tweets leads users to information overload. Therefore, filtering interesting tweets based on users' interest profiles from real-time stream can be helpful for users to easily access the relevant and key information hidden among the tweets. On the other hand, real-time twitter stream contains enormous amount of noisy and redundant tweets, which are not expected to be seen for users. Hence, the filtering process should consider previously pushed interesting tweets to provide users with diverse tweets. What's more, different from traditional document summarization methods which focus on static dataset, the twitter stream is dynamic, fast-arriving and large-scale, which means we have to decide whether to filter the coming tweet for users from the real-time stream as early as possible. In this paper, we propose a novel adaptive evolutionary filtering framework to push interesting tweets for users from real-time twitter stream. First, we propose an adaptive evolutionary filtering algorithm to filter interesting tweets from the twitter stream with respect to user interest profiles. And then we utilize the maximal marginal relevance model in fixed time window to estimate the relevance and diversity of potential tweets. Besides, to overcome the enormous number of redundant tweets and characterize the diversity of potential tweets, we propose a hierarchical tweet representation learning model (HTM) to learn the tweet representations dynamically over time. Experiments on large scale real-time twitter stream datasets demonstrate the efficiency and effectiveness of our framework.
更多查看译文
关键词
Twitter Stream,Evolutionary Filtering,Timeline
AI 理解论文
溯源树
样例
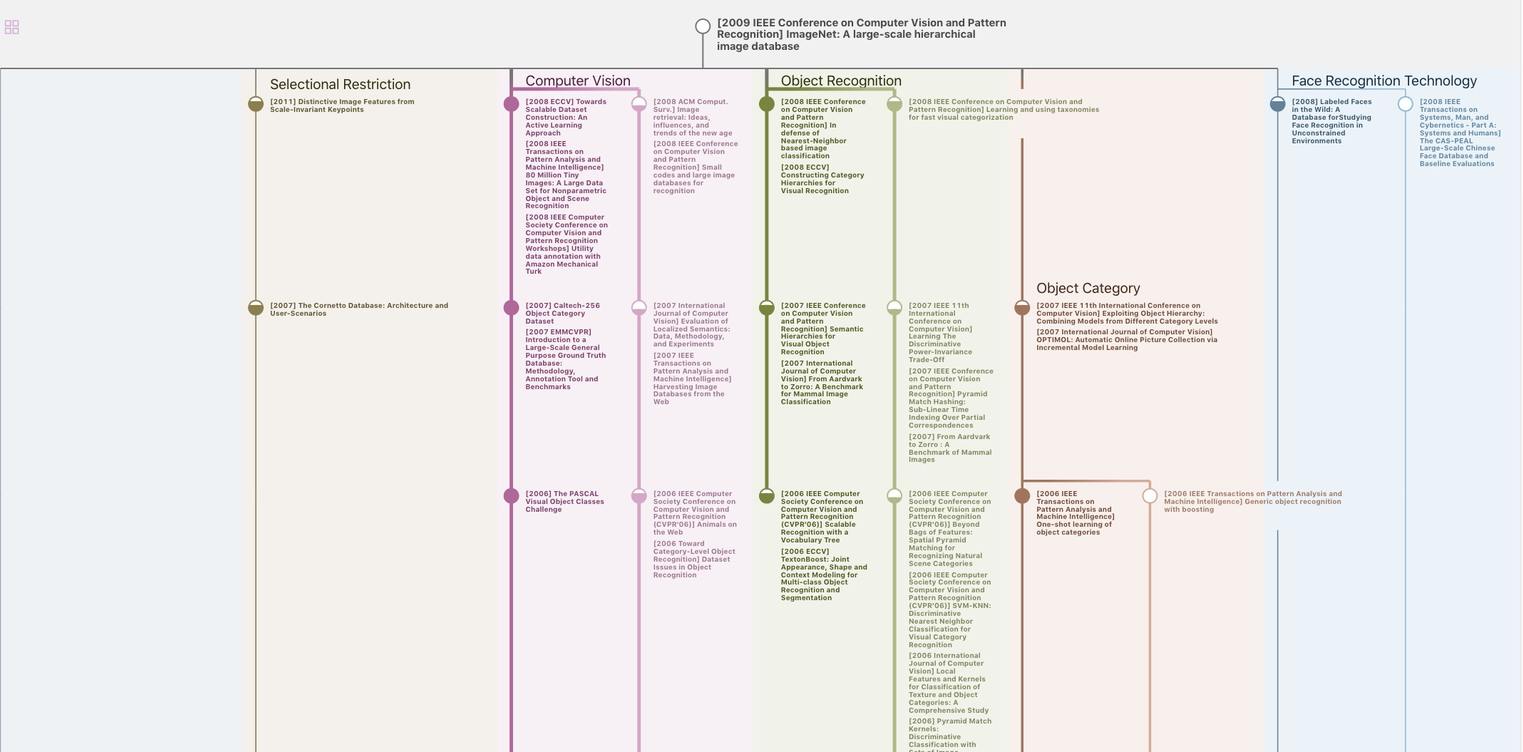
生成溯源树,研究论文发展脉络
Chat Paper
正在生成论文摘要