Learning to Extract Conditional Knowledge for Question Answering using Dialogue
ACM International Conference on Information and Knowledge Management(2016)
摘要
Knowledge based question answering (KBQA) has attracted much attention from both academia and industry in the field of Artificial Intelligence. However, many existing knowledge bases (KBs) are built by static triples. It is hard to answer user questions with different conditions, which will lead to significant answer variances in questions with similar intent. In this work, we propose to extract conditional knowledge base (CKB) from user question-answer pairs for answering user questions with different conditions through dialogue. Given a subject, we first learn user question patterns and conditions. Then we propose an embedding based co-clustering algorithm to simultaneously group the patterns and conditions by leveraging the answers as supervisor information. After that, we extract the answers to questions conditioned on both question pattern clusters and condition clusters as a CKB. As a result, when users ask a question without clearly specifying the conditions, we use dialogues in natural language to chat with users for question specification and answer retrieval. Experiments on real question answering (QA) data of two commercial organizations show that the dialogue model using automatically extracted CKB can more accurately answer user questions and significantly improve user satisfaction for questions with missing conditions.
更多查看译文
关键词
Knowledge based question answering,conditional knowledge base,dialogue
AI 理解论文
溯源树
样例
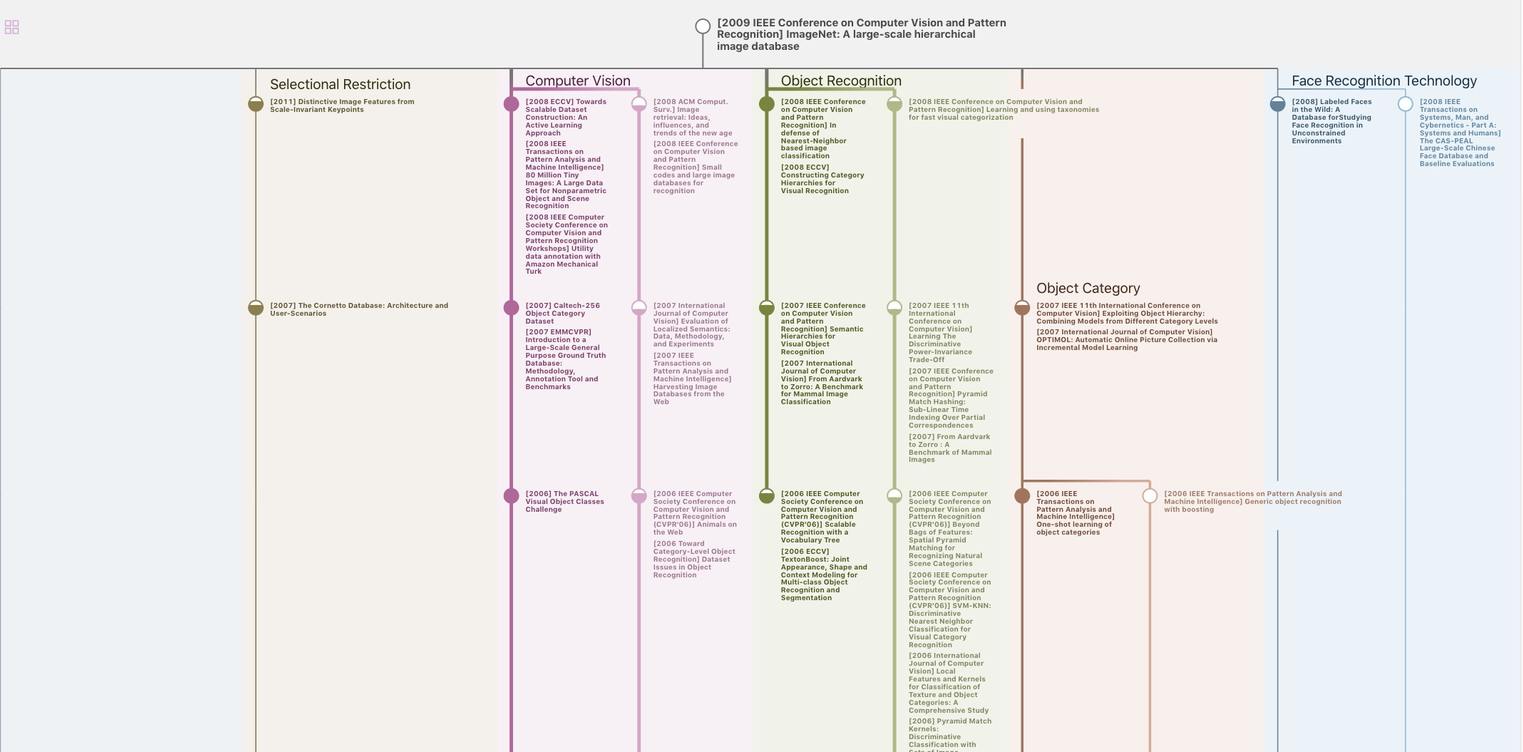
生成溯源树,研究论文发展脉络
Chat Paper
正在生成论文摘要