Estimating Time Models for News Article Excerpts
ACM International Conference on Information and Knowledge Management(2016)
摘要
Time embedded as temporal expressions have been leveraged in various tasks in information retrieval, extraction, and summarization. However, it is often difficult to ground text excerpts to precise time intervals due to the inherent uncertainty arising from either missing or multiple expressions at year, month, and day time granularities.
In this paper, we address the problem of estimating excerpt-time model (analogous to language model in text) as a probability distribution over chronons, capturing the true temporal scope of an event described in a given excerpt from a news article. We propose a semi-supervised distribution propagation framework that models various inter-excerpt relations. It then leverages the relations to propagate empirical excerpt-time models, estimated from expressions in temporally annotated excerpts, to strongly related excerpts with missing annotations. To generate a test set for experiments, we first randomly sample 100 Wikipedia events as queries, and then for each retrieve top-10 news articles containing on average 250 excerpts from the English Gigaword corpus. The evaluation shows that our method estimates better excerpt-time models, as compared to several baselines, in terms of traditional measures like Precision, Recall, F1, MAP, and NDCG, and new Model Quality measure and Generative Power measures that we propose.
更多查看译文
关键词
excerpt time model,temporally scoping excerpts,distribution propagation,temporal content analysis,probabilistic models
AI 理解论文
溯源树
样例
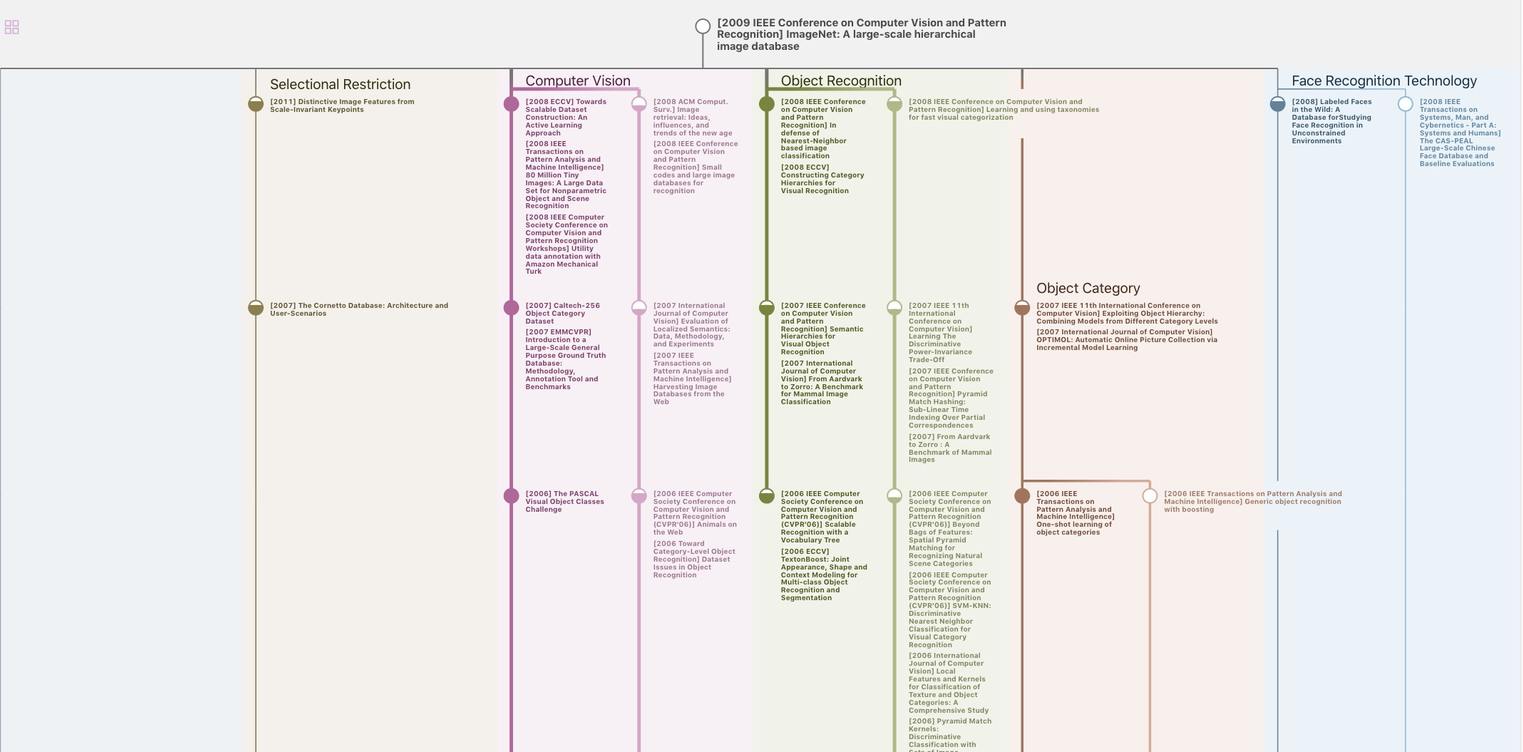
生成溯源树,研究论文发展脉络
Chat Paper
正在生成论文摘要