Attribute-based Crowd Entity Resolution
ACM International Conference on Information and Knowledge Management(2016)
摘要
We study the problem of using the crowd to perform entity resolution (ER) on a set of records. For many types of records, especially those involving images, such a task can be difficult for machines, but relatively easy for humans. Typical crowd-based ER approaches ask workers for pairwise judgments between records, which quickly becomes prohibitively expensive even for moderate numbers of records. In this paper, we reduce the cost of pairwise crowd ER approaches by soliciting the crowd for attribute labels on records, and then asking for pairwise judgments only between records with similar sets of attribute labels. However, due to errors induced by crowd-based attribute labeling, a naive attribute-based approach becomes extremely inaccurate even with few attributes. To combat these errors, we use error mitigation strategies which allow us to control the accuracy of our results while maintaining significant cost reductions. We develop a probabilistic model which allows us to determine the optimal, lowest-cost combination of error mitigation strategies needed to achieve a minimum desired accuracy. We test our approach with actual crowdworkers on a dataset of celebrity images, and find that our results yield crowd ER strategies which achieve high accuracy yet are significantly lower cost than pairwise-only approaches.
更多查看译文
关键词
crowdsourcing,crowd computation,data integration,entity resolution,database systems
AI 理解论文
溯源树
样例
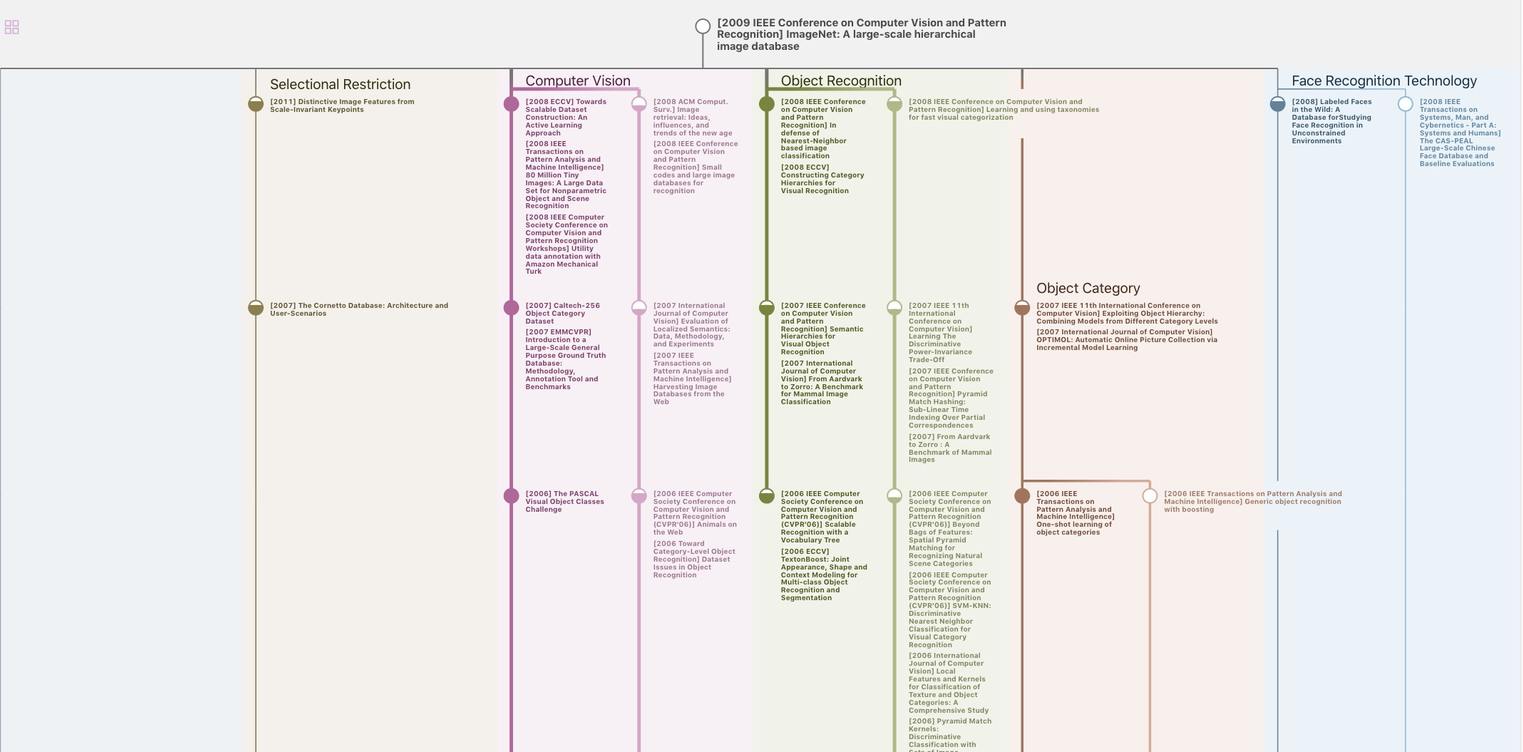
生成溯源树,研究论文发展脉络
Chat Paper
正在生成论文摘要