The Role of Relevance in Sponsored Search
ACM International Conference on Information and Knowledge Management(2016)
摘要
Sponsored search aims at retrieving the adverts that in the one hand meet users' intent reflected in their search queries, and in the other hand attract user clicks in order to generate revenue. Adverts are typically ranked based on their expected revenue that is computed as the product between their predicted probability of being clicked (i.e., namely clickability) and their advertiser provided bid. The relevance of an advert to a user query is implicitly captured by the predicted clickability of the advert, assuming that relevant adverts are more likely to attract user clicks. However, this approach easily biases the ranking toward adverts having rich click history, and may incorrectly show irrelevant adverts whose clickability is not accurately predicted due to lack of click history, or miss the opportunity to show new adverts that are highly relevant to user queries but do not have enough click history.
To address this problem, we propose to explicitly measure the relevance between an advert and a query without relying on the advert's click history, and present different ways of leveraging this relevance to improve user search experience without reducing search engine revenue. Specifically, we propose a machine learning approach that solely relies on text-based features to measure the relevance between an advert and a query. Then, we discuss how the introduced relevance can be used in four important use cases: pre-filtering of irrelevant adverts, recovering adverts with little history (i.e. cold adverts), improving clickability prediction, and re-ranking of the adverts on the final search result page. Offline experiments using large-scale query logs as well as online A/B tests demonstrate both the superiority of the proposed click-oblivious relevance model and the important roles that relevance can play in sponsored search.
更多查看译文
关键词
Relevance,Relevance Model,Sponsored Search
AI 理解论文
溯源树
样例
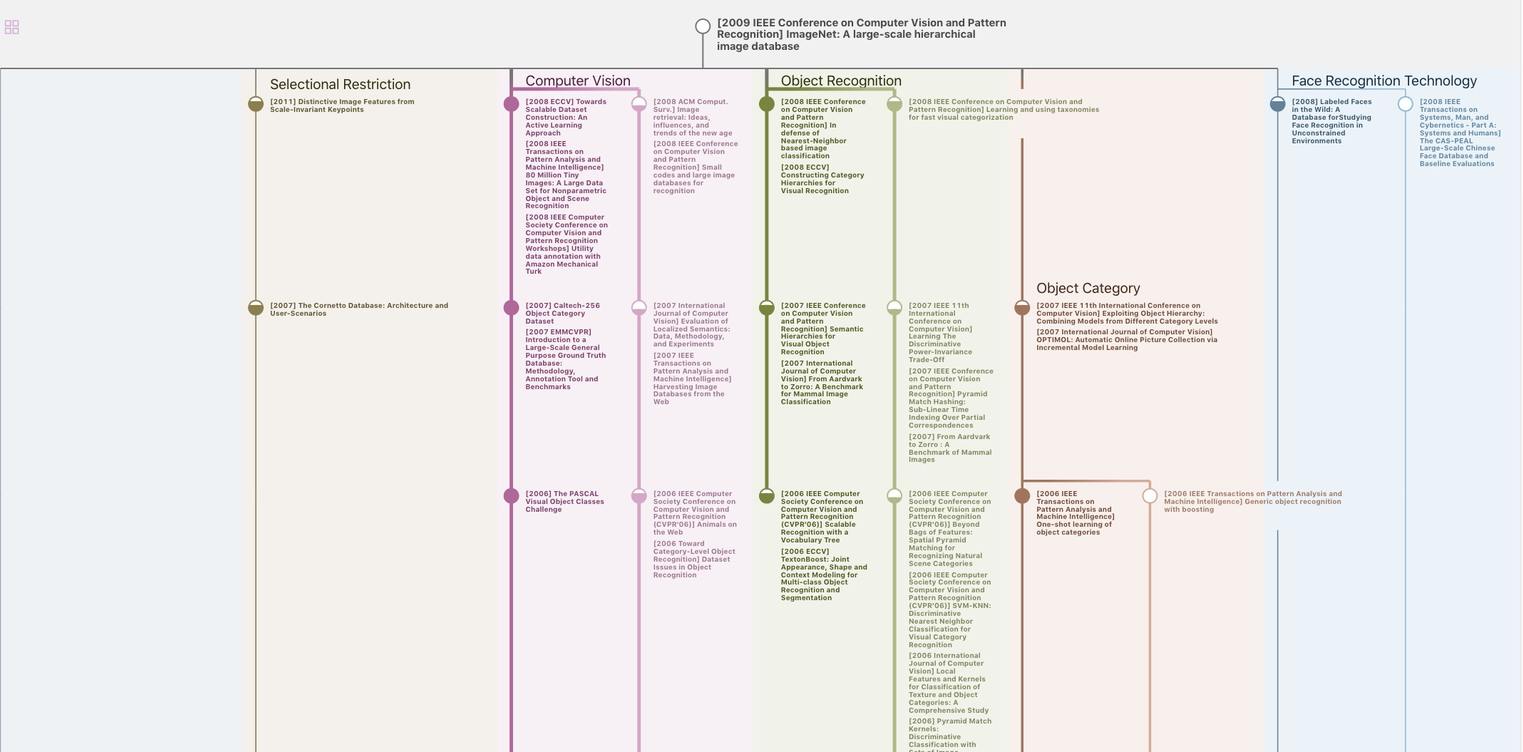
生成溯源树,研究论文发展脉络
Chat Paper
正在生成论文摘要