The difference-of-datasets framework: A statistical method to discover insight
2016 IEEE INTERNATIONAL CONFERENCE ON BIG DATA (BIG DATA)(2016)
摘要
In this paper, we motivate the utility of framing very common data analysis and business intelligence problems as a problem in understanding the differences between two datasets. We call this framework the Difference-of-Datasets (DoD) framework. We propose a simple and effective method to help find the root causes of changes, i.e. “Why did the observed change happen?” or “What drove the observed change?”. Our method is based on a hypothesis test to detect the difference in the distributions of two samples, and is tailored to large-scale correlated binary data. We apply our method to several interesting scenarios, and successfully get insights to approach the fundamental reasons for unexpected changes. While our method originates from the concepts in A/B testing, it could be extended to all areas related to data science and business intelligence.
更多查看译文
关键词
data mining, business intelligence, aggregation, hypothesis testing, causality, a/b testing, experimentation
AI 理解论文
溯源树
样例
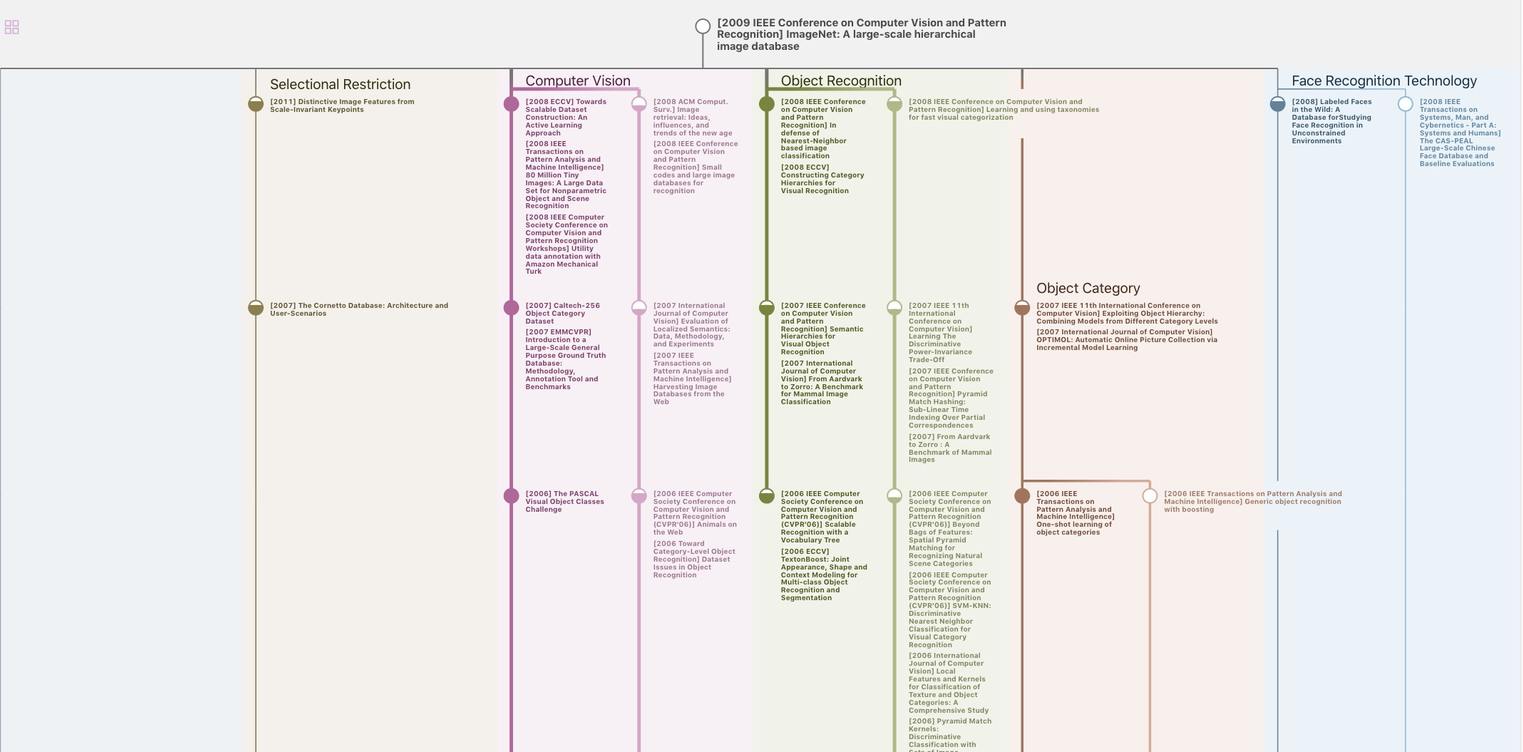
生成溯源树,研究论文发展脉络
Chat Paper
正在生成论文摘要