Feature selection for multiclass support vector machines.
AI COMMUNICATIONS(2016)
摘要
In this paper, we present and evaluate a novel method for feature selection for Multiclass Support Vector Machines (MSVM). It consists in determining the relevant features using an upper bound of generalization error proper to the multiclass case called the multiclass radius margin bound. A score derived from this bound will rank the variables in order of relevance, then, forward method will be used to select the optimal subset. The experiments are firstly conducted on simulated data to test the ability of the score to give the correct order of relevance of variables and the ability of the proposed method to find the subset giving a better error rate than the case where all features are used. Afterward, four real datasets publicly available will be used and the results will be compared with those of other methods of variable selection by MSVM.
更多查看译文
关键词
Discrimination,Multiclass Support Vectors Machines (MSVM),variables selection,hard margin MSVM models,multiclass radius-margin bound
AI 理解论文
溯源树
样例
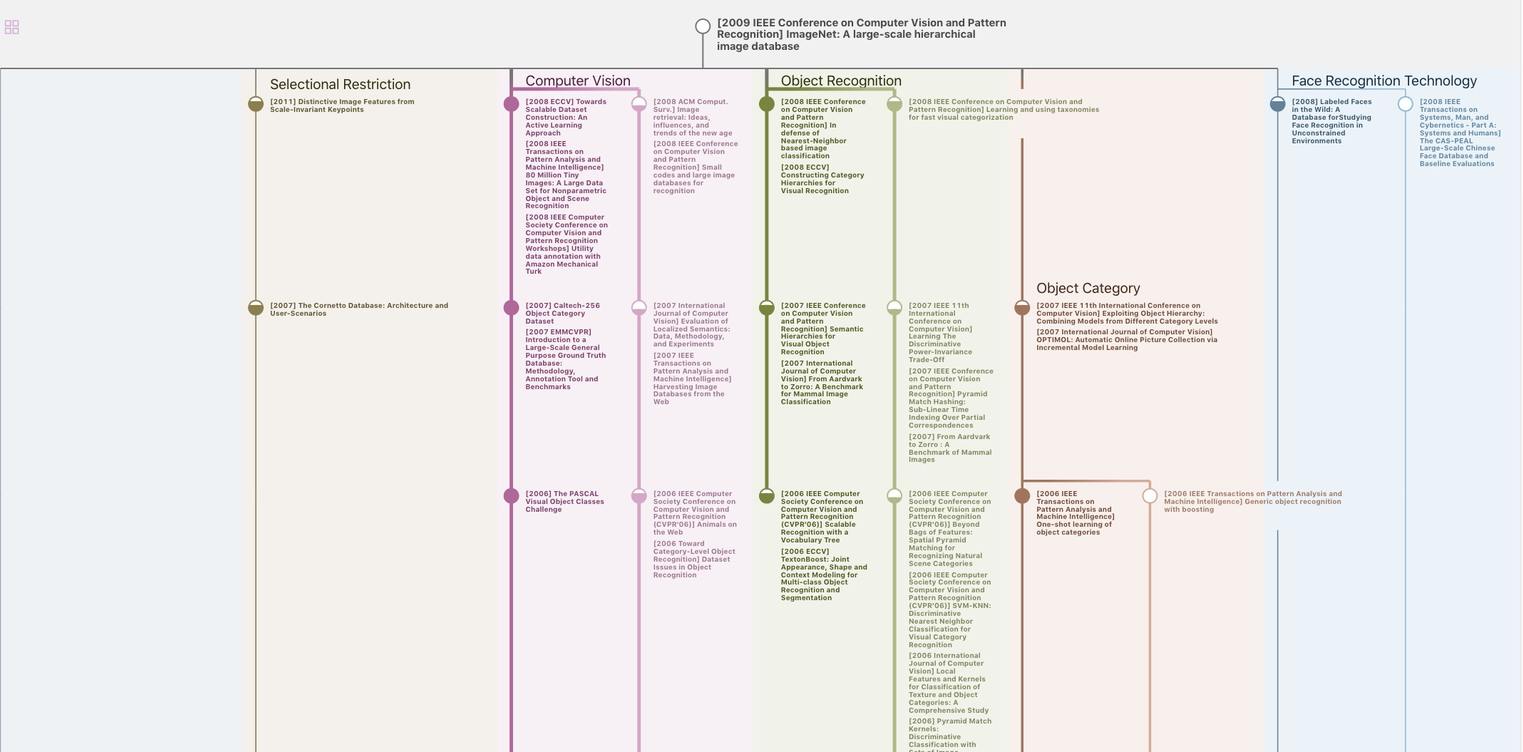
生成溯源树,研究论文发展脉络
Chat Paper
正在生成论文摘要