Efficient solutions of interactive dynamic influence diagrams using model identification.
Neurocomputing(2016)
摘要
Interactive dynamic influence diagram (I-DID) is one of the graphical frameworks for sequential decision making in partially observable environment. Subject agent in I-DID maintains beliefs over not only physical states of the environment, but also over models of the other agents. Consequently, solving I-DIDs suffers from the exponential growth of models ascribed to the other agents over time. Previous methods to solve I-DIDs aim at clustering equivalent models by comparing the entire or partial policy trees of the candidate models, which is time-consuming. In this paper, we present a new method for further reducing the model space by identifying the true model of the other agent and pruning the other irrelevant models. Toward this, we use an information-theoretic methodmutual information to measure the relevance between the candidate models and the true model in terms of predicted and observed actions of the other agent. We construct a dynamic Bayesian network to learn the value of parameters needed in the computation of mutual information. This approach bounds the model space by containing only the true model of the other agent. We evaluate our approach on multiple problem domains and empirically demonstrate the efficiency in solving I-DIDs.
更多查看译文
关键词
Interactive dynamic influence diagram,Multi-agent dynamic decision making,Mutual information,Model identification
AI 理解论文
溯源树
样例
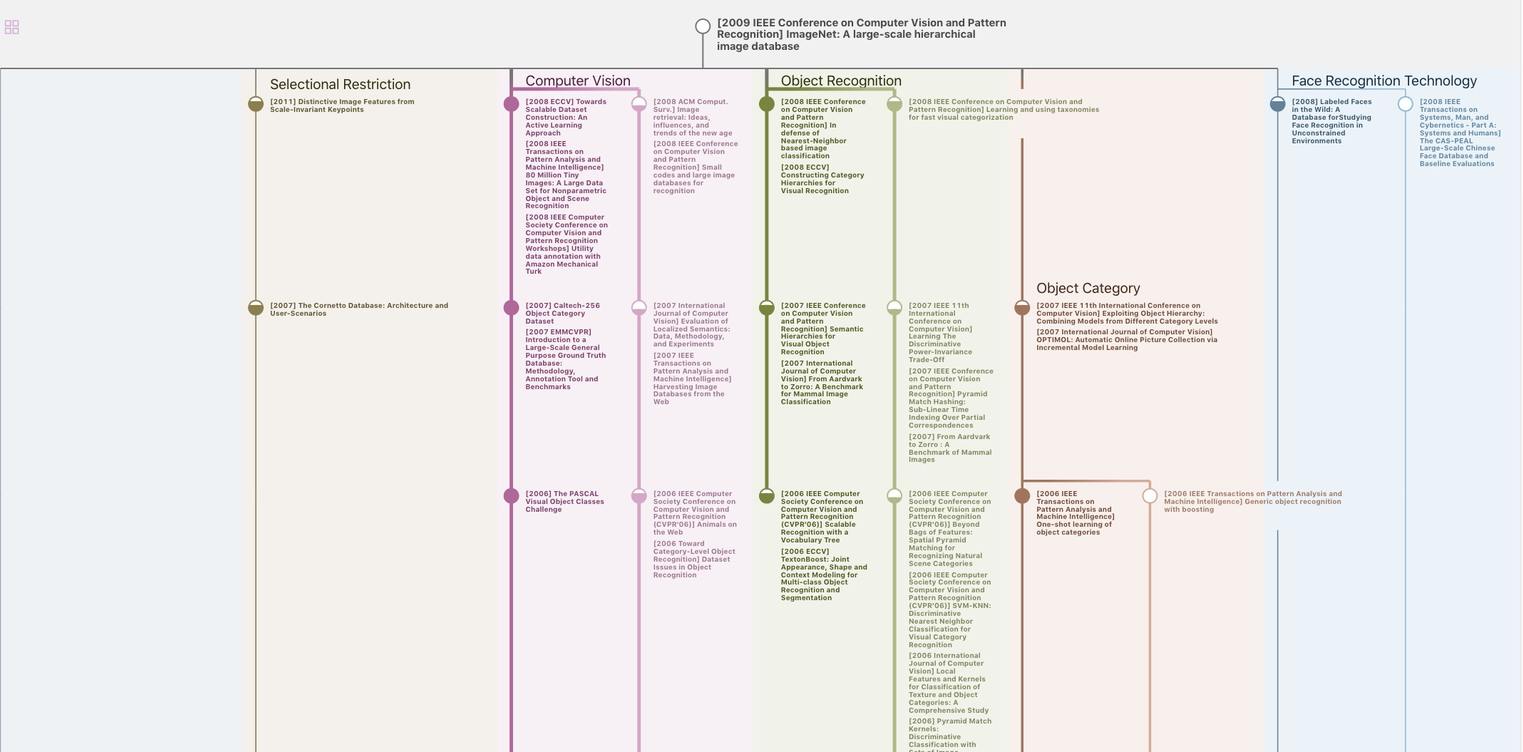
生成溯源树,研究论文发展脉络
Chat Paper
正在生成论文摘要