Automatic classification and localization of prostate cancer using multi-parametric MRI/MRS.
Biomedical Signal Processing and Control(2017)
摘要
Prostate cancer is considered to be the third and sixth leading cause of death from cancer in men in developed and developing countries, respectively. As Multiparametric Magnetic Resonance Imaging (mp-MRI) and Magnetic Resonance Spectroscopic Imaging (MRSI) play an important role in the detection and the localization of cancerous tissues, in this paper, we propose a SVM and Random Forest based supervised classification schema, based on three classes (Healthy, Benign, Malignant) and on an MRI/MRSI data base of 34 patients. A total of 7711 spectroscopy voxels were exploited. The first contribution of this paper is to present improvements of the automatic classification results compared with those of Parfait, thanks to an improvement in the quality of spectra. We also improved the global detection by introducing mp-MRI based features in the classification process. We selected the most discriminative features by evaluating several combinations of MRI modalities. Moreover, we have extended the analysis to the entire prostate gland (peripheral zone (PZ) and central gland (CG)). We evaluated the SVM classifier's ability to discriminate healthy and malignant voxels and the proposed method produces a global error rate of 1%, sensitivity of 99.1% and specificity of 98.4%. The three classes, including benign voxels data were then evaluated. An error rate of 18.2%, a sensitivity of 72% and a specificity of 88% were obtained when associating Random Forest classifier, MRSI, Dynamic Contrast-Enhanced MRI and Diffusion-Weighted MRI. We finally present classification results in the form of color-coded maps, which are a computer aided diagnosis tool which could help in the evaluation of the results and could also provide an estimation of tumor shape and volume. (C) 2016 Elsevier Ltd. All rights reserved.
更多查看译文
关键词
Prostate cancer localization,Support vector machine (SVM),Random Forest,Supervised learning,Magnetic Resonance Spectroscopy Imaging (MRSI),Magnetic Resonance Imaging (MRI),Multimodality analysis
AI 理解论文
溯源树
样例
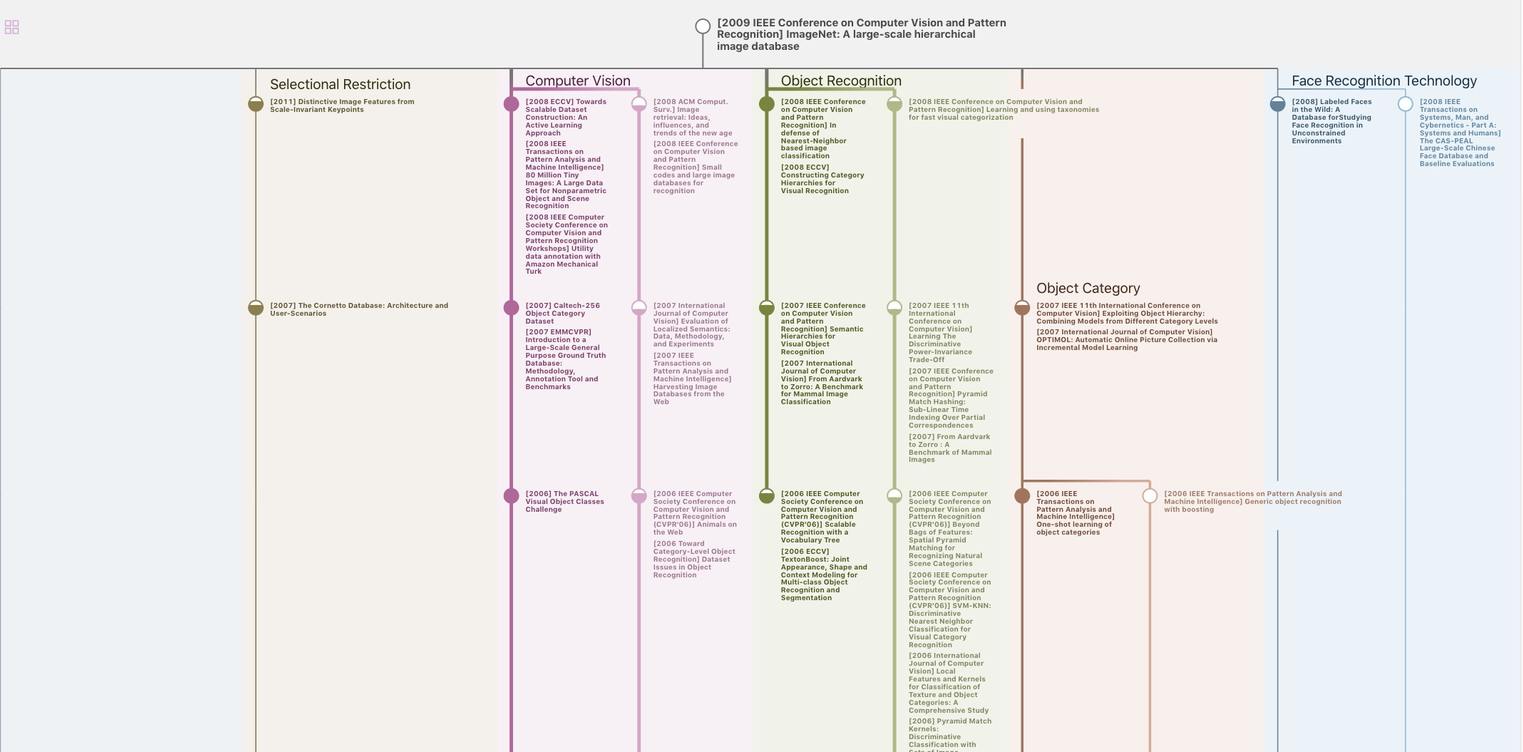
生成溯源树,研究论文发展脉络
Chat Paper
正在生成论文摘要