HMM Adaptation for Improving a Human Activity Recognition System.
ALGORITHMS(2016)
摘要
When developing a fully automatic system for evaluating motor activities performed by a person, it is necessary to segment and recognize the different activities in order to focus the analysis. This process must be carried out by a Human Activity Recognition (HAR) system. This paper proposes a user adaptation technique for improving a HAR system based on Hidden Markov Models (HMMs). This system segments and recognizes six different physical activities (walking, walking upstairs, walking downstairs, sitting, standing and lying down) using inertial signals from a smartphone. The system is composed of a feature extractor for obtaining the most relevant characteristics from the inertial signals, a module for training the six HMMs (one per activity), and the last module for segmenting new activity sequences using these models. The user adaptation technique consists of a Maximum A Posteriori (MAP) approach that adapts the activity HMMs to the user, using some activity examples from this specific user. The main results on a public dataset have reported a significant relative error rate reduction of more than 30%. In conclusion, adapting a HAR system to the user who is performing the physical activities provides significant improvement in the system's performance.
更多查看译文
关键词
user adaptation,human activity segmentation,HMMs,smartphone inertial sensors
AI 理解论文
溯源树
样例
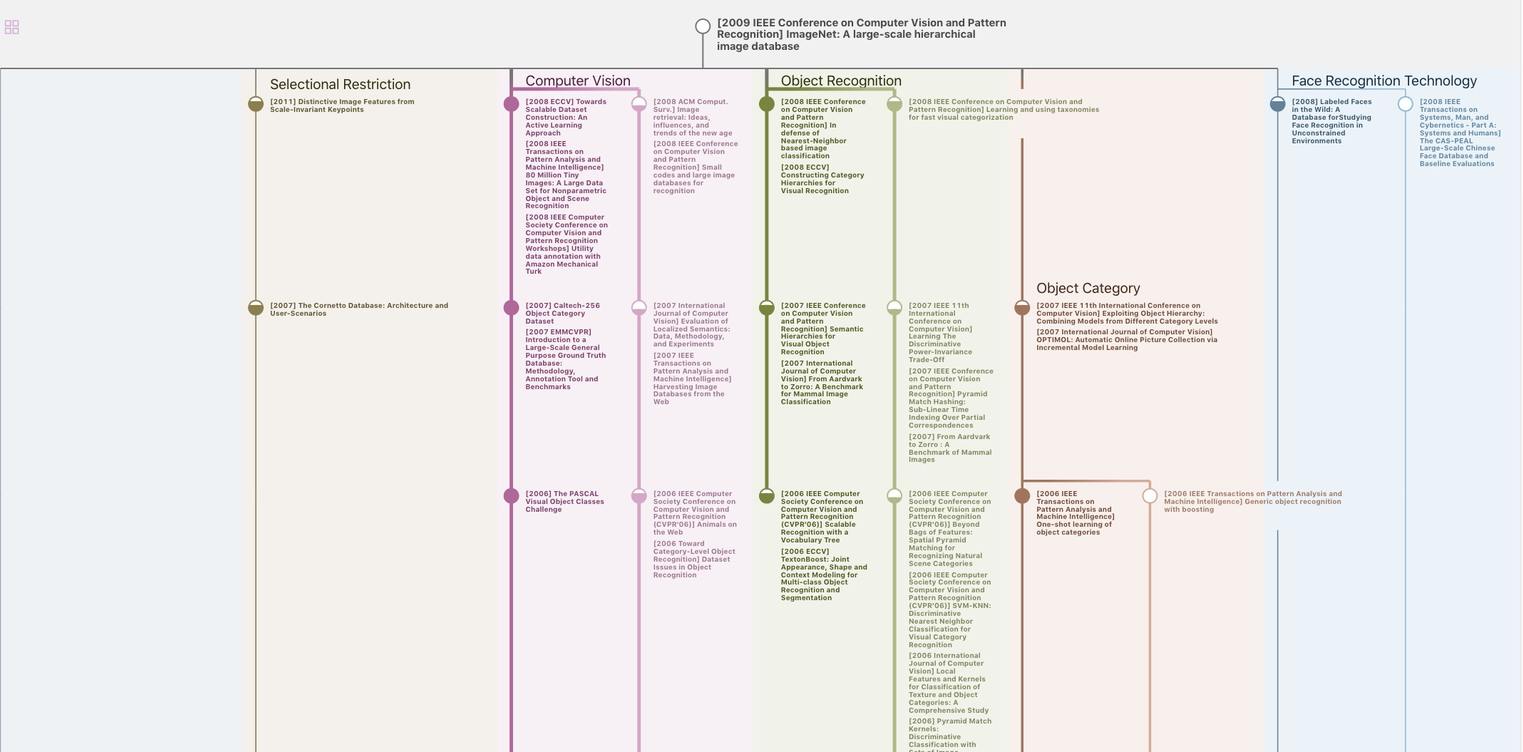
生成溯源树,研究论文发展脉络
Chat Paper
正在生成论文摘要