Photo Filter Recommendation by Category-Aware Aesthetic Learning
IEEE transactions on multimedia(2017)
摘要
Nowadays, social media has become a popular platform for the public to share photos. To make photos more visually appealing, users usually apply filters on their photos without domain knowledge. However, due to the growing number of filter types, it becomes a major issue for users to choose the best filter type. For this purpose, filter recommendation for photo aesthetics takes an important role in image quality ranking problems. In these years, several works have declared that convolutional neural networks (CNNs) outperform traditional methods in image aesthetic categorization, which classifies images into high or low quality. Most of them do not consider the effect on filtered images; hence, we propose a novel image aesthetic learning for filter recommendation. Instead of binarizing image quality, we adjust the state-of-the-art CNN architectures and design a pairwise loss function to learn the embedded aesthetic responses in hidden layers for filtered images. Based on our pilot study, we observe image categories (e.g., portrait, landscape, food) will affect user preference on filter selection. We further integrate category classification into our proposed aesthetic-oriented models. To the best of our knowledge, there is no public dataset for aesthetic judgment with filtered images. We create a new dataset called filter aesthetic comparison dataset (FACD). It is the first dataset containing 28 160 filtered images and 42 240 user preference labels. We conduct experiments on the collected FACD for filter recommendation, and the results show that our proposed category-aware aesthetic learning outperforms aesthetic classification methods (e.g., 12% relative improvement).
更多查看译文
关键词
Image quality,Feature extraction,Social network services,Neural networks,Image color analysis,Electronic mail,Object detection
AI 理解论文
溯源树
样例
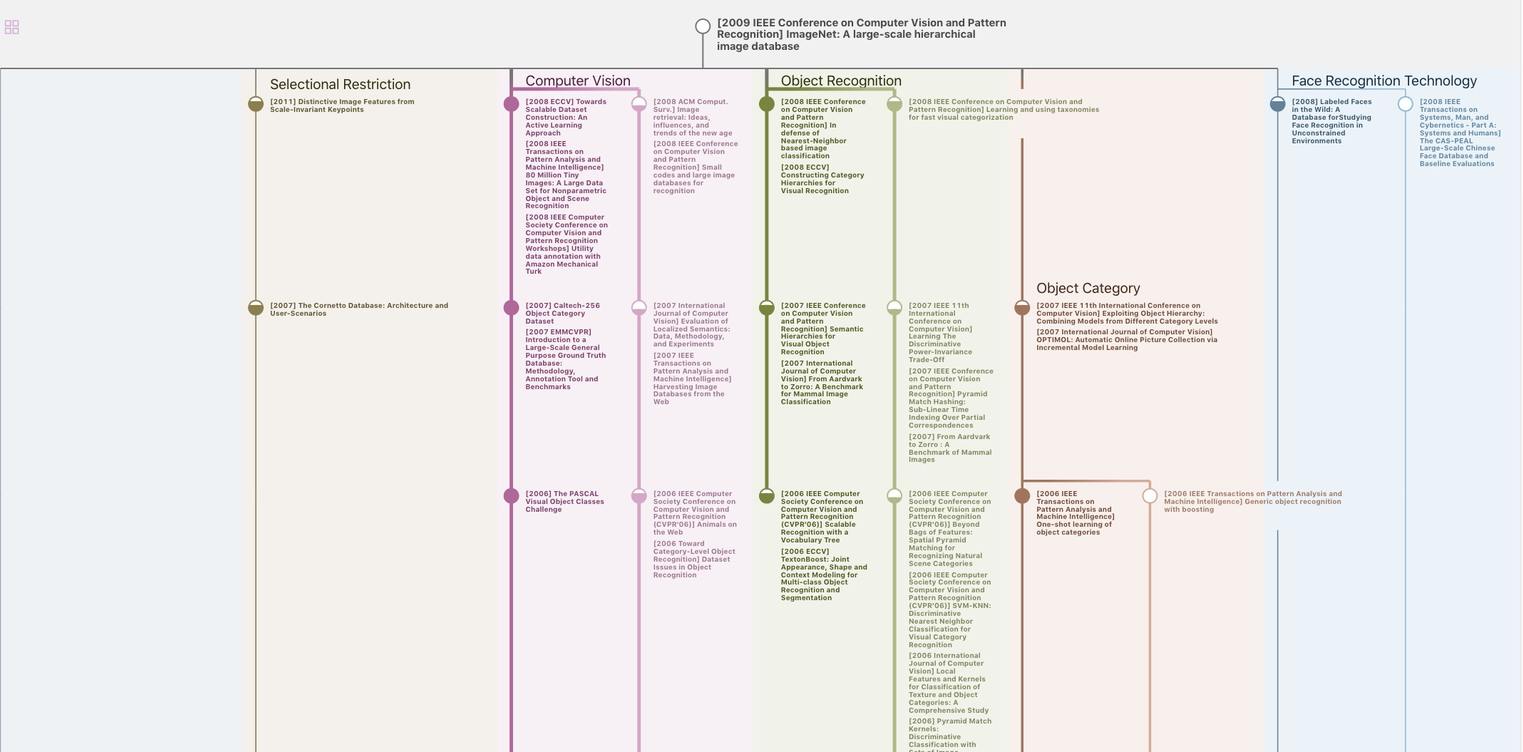
生成溯源树,研究论文发展脉络
Chat Paper
正在生成论文摘要