Approximate Search with Quantized Sparse Representations
Computer Vision – ECCV 2016 Lecture Notes in Computer Science(2016)
Abstract
This paper tackles the task of storing a large collection of vectors, such as visual descriptors, and of searching in it. To this end, we propose to approximate database vectors by constrained sparse coding, where possible atom weights are restricted to belong to a finite subset. This formulation encompasses, as particular cases, previous state-of-the-art methods such as product or residual quantization. As opposed to traditional sparse coding methods, quantized sparse coding includes memory usage as a design constraint, thereby allowing us to index a large collection such as the BIGANN billion-sized benchmark. Our experiments, carried out on standard benchmarks, show that our formulation leads to competitive solutions when considering different trade-offs between learning/coding time, index size and search quality.
MoreTranslated text
Key words
Indexing,Approximate nearest neighbor search,Vector quantization,Sparse coding
AI Read Science
Must-Reading Tree
Example
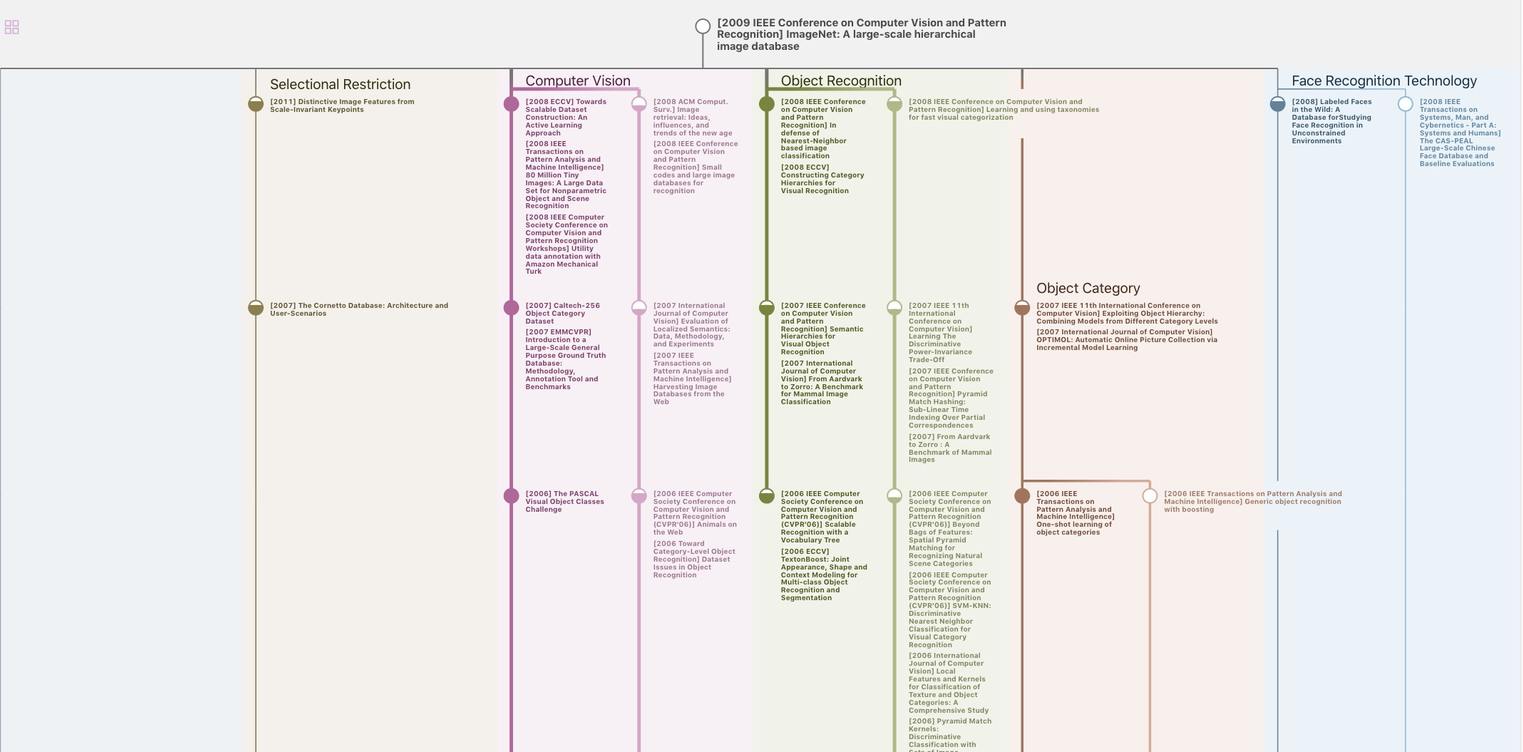
Generate MRT to find the research sequence of this paper
Chat Paper
Summary is being generated by the instructions you defined