Understanding Data Augmentation for Classification: When to Warp?
2016 INTERNATIONAL CONFERENCE ON DIGITAL IMAGE COMPUTING: TECHNIQUES AND APPLICATIONS (DICTA)(2016)
摘要
In this paper we investigate the benefit of augmenting data with synthetically created samples when training a machine learning classifier. Two approaches for creating additional training samples are data warping, which generates additional samples through transformations applied in the data-space, and synthetic over-sampling, which creates additional samples in feature-space. We experimentally evaluate the benefits of data augmentation for a convolutional backpropagation-trained neural network, a convolutional support vector machine and a convolutional extreme learning machine classifier, using the standard MNIST handwritten digit dataset. We found that while it is possible to perform generic augmentation in feature-space, if plausible transforms for the data are known then augmentation in data-space provides a greater benefit for improving performance and reducing overfitting.
更多查看译文
关键词
data augmentation,classification,machine learning classifier,data warping,synthetic over-sampling,feature-space,convolutional backpropagation-trained neural network,convolutional support vector machine,convolutional extreme learning machine classifier,MNIST handwritten digit dataset
AI 理解论文
溯源树
样例
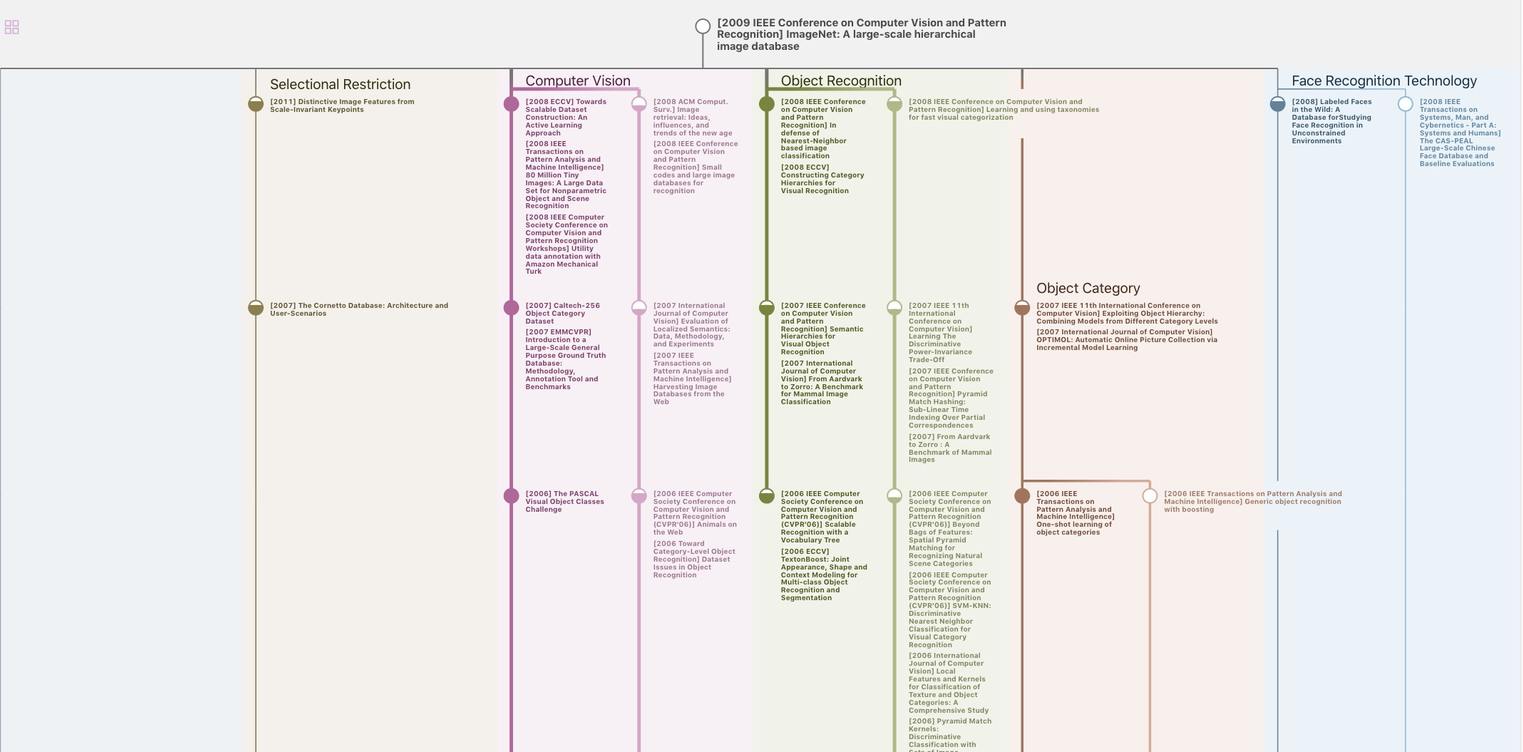
生成溯源树,研究论文发展脉络
Chat Paper
正在生成论文摘要