GARCH-based robust clustering of time series.
Fuzzy Sets and Systems(2016)
摘要
In this paper we propose different robust fuzzy clustering models for classifying heteroskedastic (volatility) time series, following the so-called model-based approach to time series clustering and using a partitioning around medoids procedure. The proposed models are based on a GARCH parametric modeling of the time series, i.e. the unconditional volatility and the time-varying volatility GARCH representation of the time series. We first suggest a timid robustification of the fuzzy clustering. Then, we propose three robust fuzzy clustering models belonging to the so-called metric, noise and trimmed approaches, respectively. Each model neutralizes the negative effects of the outliers in the clustering process in a different manner. In particular, the first robust model, based on the metric approach, achieves its robustness with respect to outliers by taking into account a robust distance measure; the second, based on the noise approach, achieves its robustness by introducing a noise cluster represented by a noise prototype; the third, based on the trimmed approach, achieves its robustness by trimming away a certain fraction of outlying time series. The usefulness and effectiveness of the proposed clustering models is illustrated by means of a simulation study and two applications in finance and economics.
更多查看译文
关键词
Heteroskedastic time series,Unconditional and time-varying volatility,GARCH model,Fuzzy partitioning around medoids,Outliers,Robust metric,Noise cluster,Trimming,Volatilities daily stocks returns,International stock-market volatility daily returns
AI 理解论文
溯源树
样例
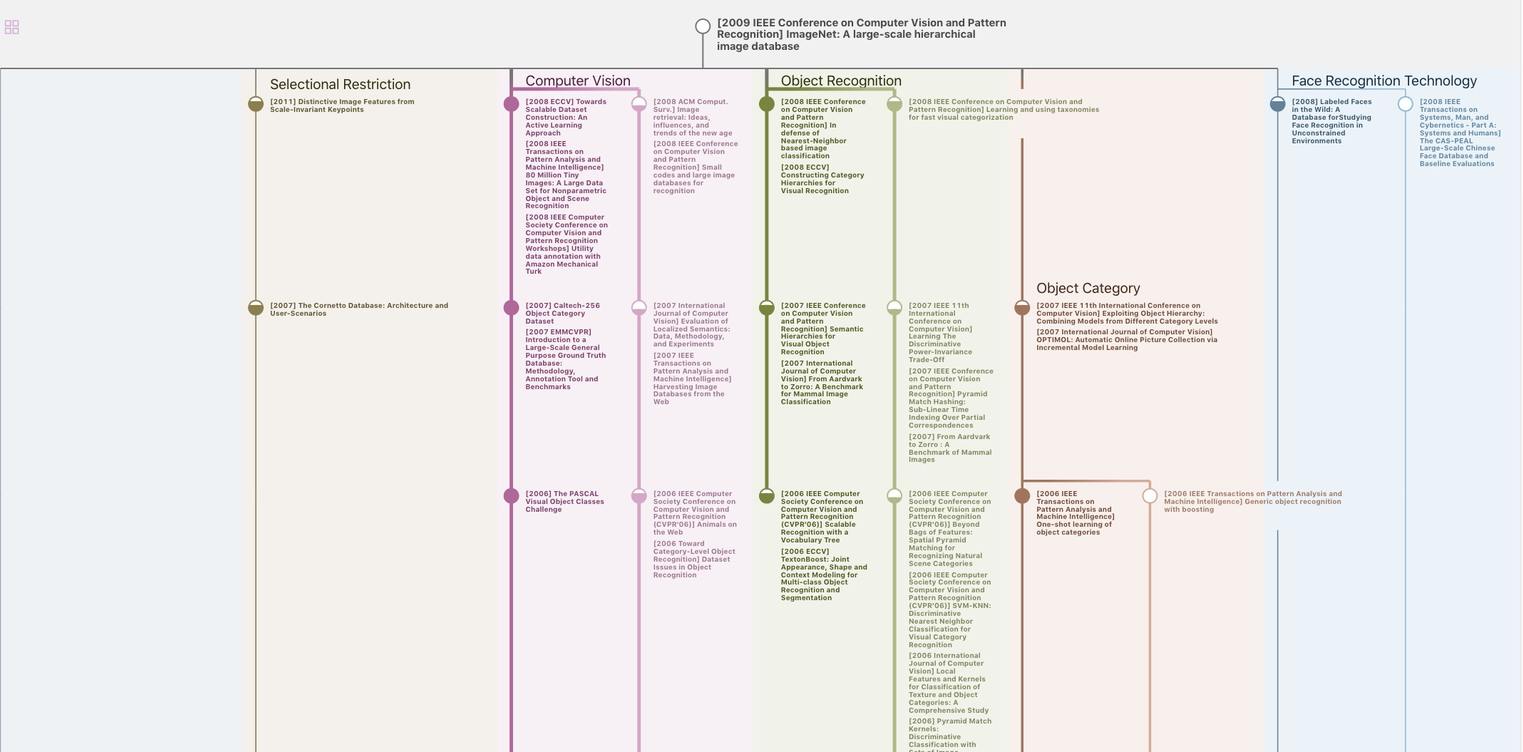
生成溯源树,研究论文发展脉络
Chat Paper
正在生成论文摘要