Graphical models via joint quantile regression with component selection
Journal of Multivariate Analysis(2016)
摘要
A graphical model is used for describing interrelationships among multiple variables. In many cases, the multivariate Gaussian assumption is made partly for its simplicity but the assumption is hardly met in actual applications. In order to avoid dependence on a rather strong assumption, we propose to infer the graphical model via joint quantile regression with component selection, since the components of quantile regression carry information to infer the conditional independence. We demonstrate the advantages of our approach using simulation studies and apply our method to an interesting real biological dataset, where the dependence structure is highly complex.
更多查看译文
关键词
62
AI 理解论文
溯源树
样例
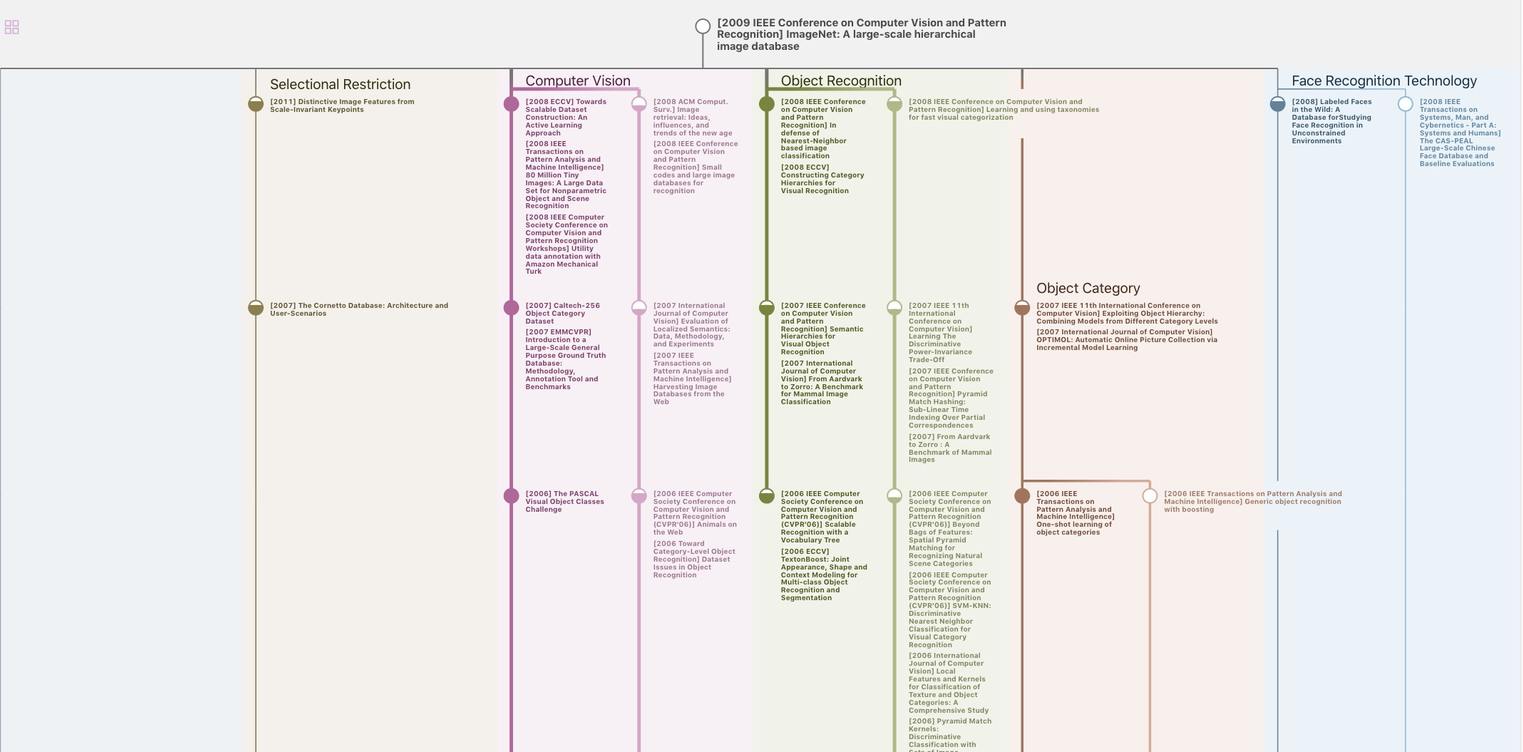
生成溯源树,研究论文发展脉络
Chat Paper
正在生成论文摘要