Integrating Topic and Latent Factors for Scalable Personalized Review-based Rating Prediction.
IEEE Trans. Knowl. Data Eng.(2016)
摘要
Personalized review-based rating prediction, a newly emerged research problem, aims at inferring users’ ratings over their unrated items using existing reviews and corresponding ratings. While some researchers proposed to learn topic factor from review text to obtain interpretability for rating prediction, they often overlooked the fact that the learned topic factors are limited to review text and cannot fully reveal the complicated relations between reviews and ratings. Moreover, topic modeling based solutions for this problem usually utilize Gibbs sampling algorithms to learn topics and word distributions, resulting in non-negligible computational overload. To address the above challenges, we propose an integrated topic and latent factor model (ITLFM), which combines topic and latent factors in a linear way to make them complement each other for better accuracies in rating prediction tasks. In addition, ITLFM models review text through an additive topic model to reveal user's and item's topic factors simultaneously. To ensure high learning efficiency, we design a hybrid stochastic learning algorithm for ITLFM. We evaluate ITLFM on several standard benchmarks and compare with representative approaches. The experimental results demonstrate that the proposed ITLFM method is computationally efficient and accurate, as well as scalable for large scale applications.
更多查看译文
关键词
Predictive models,Prediction algorithms,Stochastic processes,Computational modeling,Algorithm design and analysis,Additives,Recommender systems
AI 理解论文
溯源树
样例
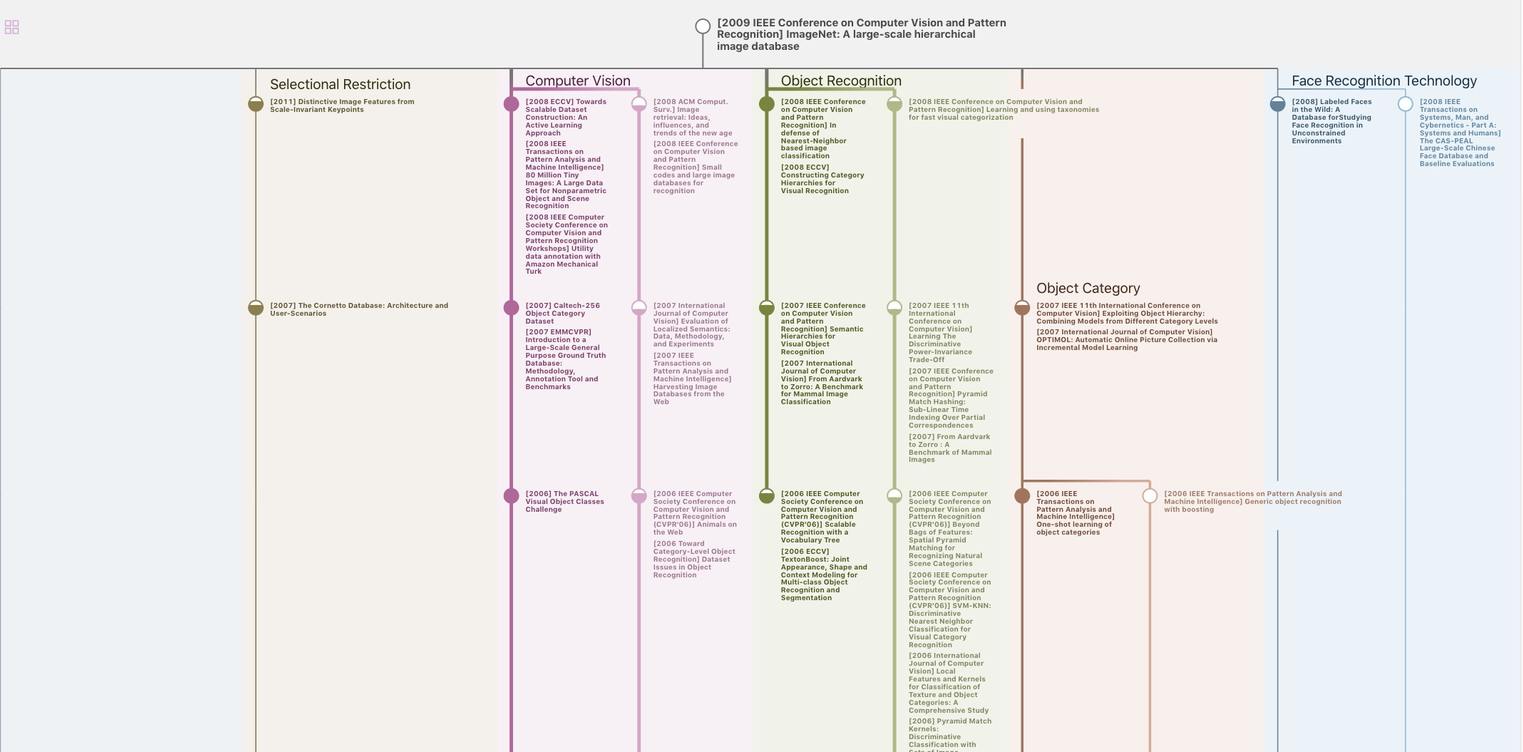
生成溯源树,研究论文发展脉络
Chat Paper
正在生成论文摘要