Effective and Efficient Global Context Verification for Image Copy Detection.
IEEE Trans. Information Forensics and Security(2017)
摘要
To detect illegal copies of copyrighted images, recent copy detection methods mostly rely on the bag-of-visual-words (BOW) model, in which local features are quantized into visual words for image matching. However, both the limited discriminability of local features and the BOW quantization errors will lead to many false local matches, which make it hard to distinguish similar images from copies. Geometric consistency verification is a popular technology for reducing the false matches, but it neglects global context information of local features and thus cannot solve this problem well. To address this problem, this paper proposes a global context verification scheme to filter false matches for copy detection. More specifically, after obtaining initial scale invariant feature transform (SIFT) matches between images based on the BOW quantization, the overlapping region-based global context descriptor (OR-GCD) is proposed for the verification of these matches to filter false matches. The OR-GCD not only encodes relatively rich global context information of SIFT features but also has good robustness and efficiency. Thus, it allows an effective and efficient verification. Furthermore, a fast image similarity measurement based on random verification is proposed to efficiently implement copy detection. In addition, we also extend the proposed method for partial-duplicate image detection. Extensive experiments demonstrate that our method achieves higher accuracy than the state-of-the-art methods, and has comparable efficiency to the baseline method based on the BOW quantization.
更多查看译文
关键词
Feature extraction,Context,Quantization (signal),Velocity measurement,Robustness,Visualization,Information filtering
AI 理解论文
溯源树
样例
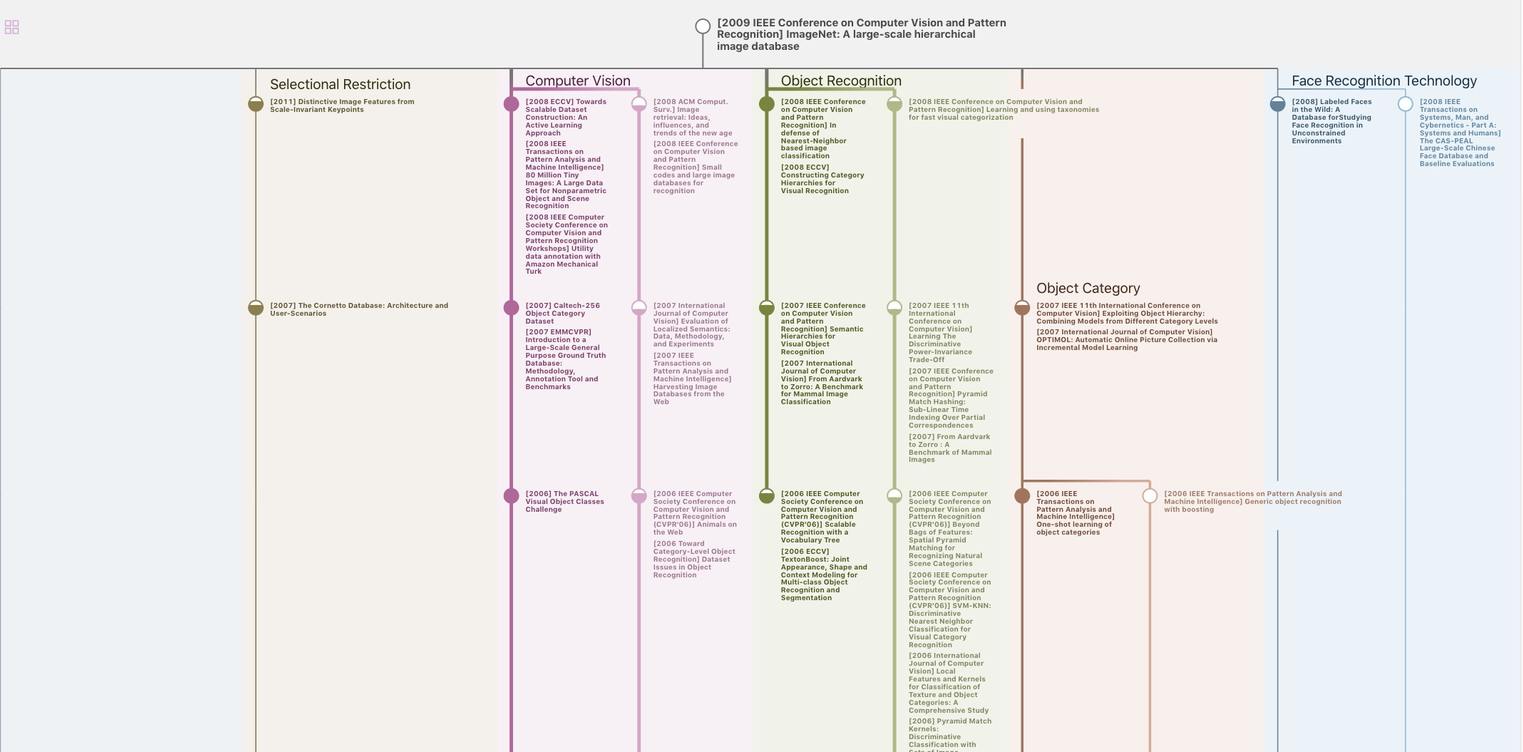
生成溯源树,研究论文发展脉络
Chat Paper
正在生成论文摘要