Ensemble Reduction via Logic Minimization.
ACM Trans. Design Autom. Electr. Syst.(2016)
摘要
An ensemble of machine learning classifiers usually improves generalization performance and is useful for many applications. However, the extra memory storage and computational cost incurred from the combined models often limits their potential applications. In this article, we propose a new ensemble reduction method called CANOPY that significantly reduces memory storage and computations. CANOPY uses a technique from logic minimization for digital circuits to select and combine particular classification models from an initial pool in the form of a Boolean function, through which the reduced ensemble performs classification. Experiments on 20 UCI datasets demonstrate that CANOPY either outperforms or is very competitive with the initial ensemble and one state-of-the-art ensemble reduction method in terms of generalization error, and is superior to all existing reduction methods surveyed for identifying the smallest numbers of models in the reduced ensembles.
更多查看译文
关键词
Ensemble reduction,logic minimization,machine learning
AI 理解论文
溯源树
样例
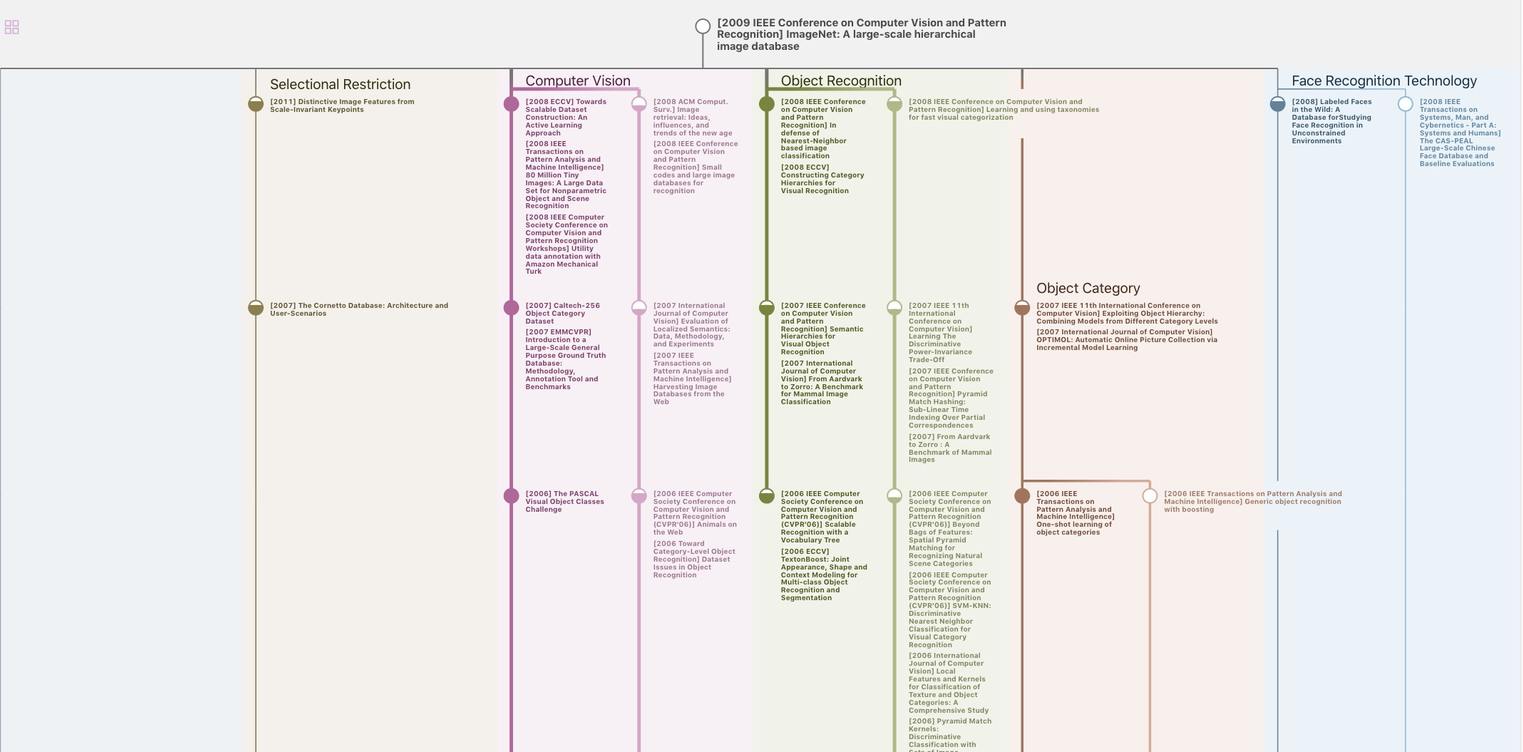
生成溯源树,研究论文发展脉络
Chat Paper
正在生成论文摘要